Susumu Tonegawa and Quentin R.V. Ferry will discuss work by Afif J. Aqrabawi, Quentin R.V. Ferry, Michele Pignatelli, Susumu Tonegawa.
Abstract:
Plasticity of synaptic strength plays a crucial role in learning and memory (1). At the cellular level, neuronal ensembles are activated during learning, thereby forming memory engrams; their subsequent reactivation results in memory recall (2). While these concepts have been reasonably well established for episodic memory, the study of the physical underpinnings of semantic memory (3), memory of generalizable abstract knowledge or ‘schemas’, have been elusive and just began (4). Knowledge affords an organism the ability to appropriate past experience for ongoing survival. Gradually and persistently, our memories build associative networks of abstractions derived from the statistical regularities existing between episodes (5). This culminates into a practical model of the world that guides prediction and future action. The hippocampus and prefrontal cortex have been implicated in knowledge formation and instantiation (6). We hypothesized that the repeated strengthening of the synapses of the engram-bearing cells that overlap between episodes with similar features serve as a representation of generalizable knowledge.
We devised a novel and general molecular genetic method that permits exclusive labeling and bidirectional manipulations of overlapping engram (OLE) cells, and applied it simultaneously to the anterior olfactory nucleus (AON), ventral hippocampus and medial prefrontal cortex of mice that were iteratively trained for reinforced olfactory “transitive inference”, a knowledge-dependent form of deductive reasoning (7). Thereby, we identified the causal role of the OLE cells in one or more of these brain regions in the generalizable abstract knowledge.
We also investigated the calcium activity of individual AON-OLE cells of mice that had undergone iterated training of acontextual olfactory memory, and found that these neurons responded to the familiar odorant more robustly than non-OLE cells. Moreover, the OLE cells exhibited a synchronous firing pattern that was accompanied by the near-simultaneous inhibition of a parallel non-OLE cell population. Individual AON neurons are known to exhibit an odorant-specific bottom-up sensory response (8,9). In contrast, the individual OLE neurons responded to a structurally distinct novel odorant as robustly as to the familiar one, suggesting that the OLE cell activities represent the generalizable abstract knowledge of the olfactory memory task.
Indeed, by reducing the dimensionality of the data (10), we discovered three latent variables that exhibit a unique pattern of oscillation known as a double-scroll attractor (11). This dynamic property of the variables is closely linked to the activity of OLE cells, which suggests that the brain organizes its representation of learned knowledge in a way that allows for efficient interpretation and integration of new information, reminiscent of a ‘cognitive map' (12). Specifically, the brain appears to use a generalized structure that is instantiated by the activity of OLE cells and can be flexibly applied to a wide range of situations. In addition, our whole-brain anatomical mapping of the olfactory knowledge network elucidated the organization of OLEs within nested AON-hippocampal-cortical feedback loops allowing the reverberation of continuous attractor states representing knowledge. Collectively, these data uncover an intuitively understandable biological mechanism for knowledge representation in the brain.
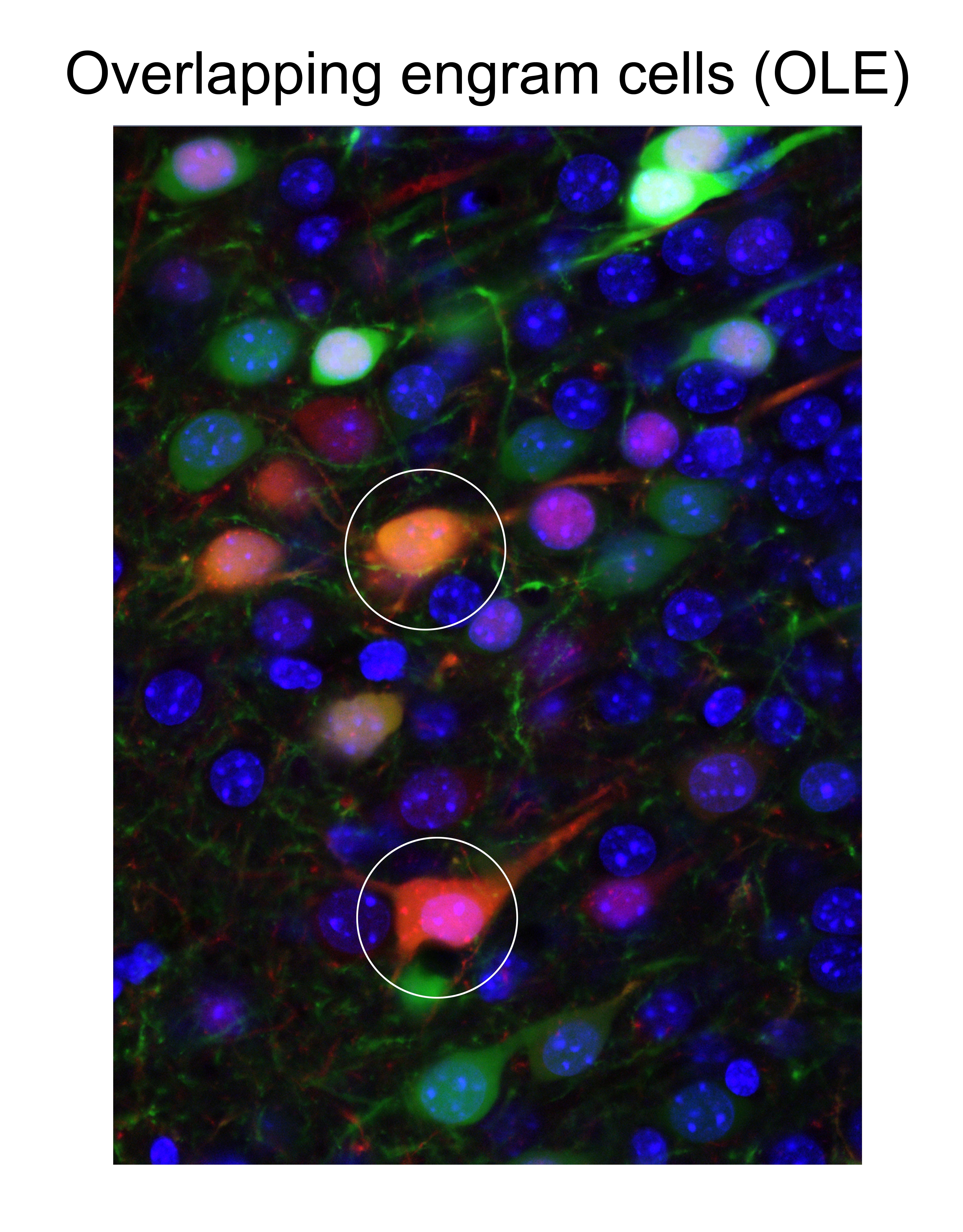
Biography:
Susumu Tonegawa received his B. Sc. from Kyoto University and his Ph.D. from University of California, San Diego (UCSD). He then undertook postdoctoral work at the Salk Institute in San Diego, before working at the Basel Institute for Immunology in Basel, Switzerland, where he performed his landmark immunology experiments. Tonegawa was the sole recipient of the Nobel Prize for Physiology or Medicine in 1987 for “his discovery of the genetic principle for generation of antibody diversity.” Tonegawa switched his field of research to brain science and founded MIT’s Center for Learning and Memory in 1994, which was renamed The Picower Institute for Learning and Memory in 2002. Using advanced techniques of gene manipulation, Tonegawa is now unraveling the molecular, cellular and neural circuit mechanisms that underlie learning and memory. His studies have broad implications for psychiatric and neurologic diseases. Tonegawa is currently the Picower Professor of Biology and Neuroscience at the Massachusetts Institute of Technology (MIT) and the Principal Investigator of the RIKEN-MIT Laboratory for Neural Circuit Genetics at MIT, as well as a RIKEN Fellow. He is also an investigator at the Howard Hughes Medical Institute. Tonegawa’s other numerous honors include: the Order of Culture (Bunkakunsho) bestowed by Japan’s Emperor, the Lasker Award granted by the Lasker Foundation in the US, the Gairdner Foundation International Award granted by the Gairdner Foundation of Canada, and the Robert Koch Prize granted by the Koch Foundation of Germany.
Please note this talk will not be recorded.
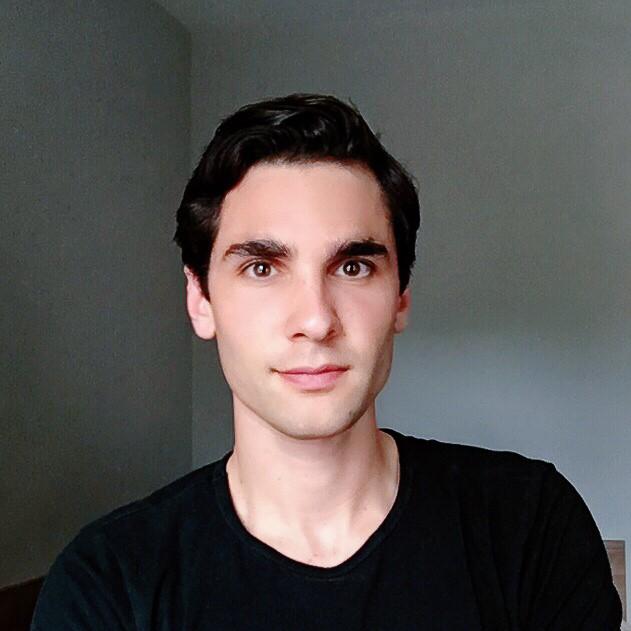
Talk Title: On the Emergence and Role of Abstract Representations in Deep Learning Systems
Abstract:
At the core of human intelligence lies the brain’s ability to learn and maintain an abstract “world model,” which attempts to succinctly and faithfully capture the latent blueprint of our environment. Amongst others, such models allow us to symbolically ground our perceptions, reason, plan, and generalize prior knowledge to effortlessly navigate novel situations.
We hypothesize that world models are formed through an iterative learning process that progressively evolves stable abstract representations (abstractions) to encode statistical regularities in our experiences. By providing a computational bottleneck, whereby the representations of perceptually distinct yet semantically-related instances converge, abstractions effectively allow downstream computations to be recycled (generalized).
To complement recent results from our lab, demonstrating the existence and importance of these stable abstract representations in the mouse brain, we ask whether state-of-the-art artificial brains rely on similar computational strategies. We find that deep learning architectures (autoencoder, RNN, transformer) trained with self-supervised objectives (reconstruction and/or prediction) also evolve stable abstractions, which all together encode in silico counterparts to the aforementioned world models.
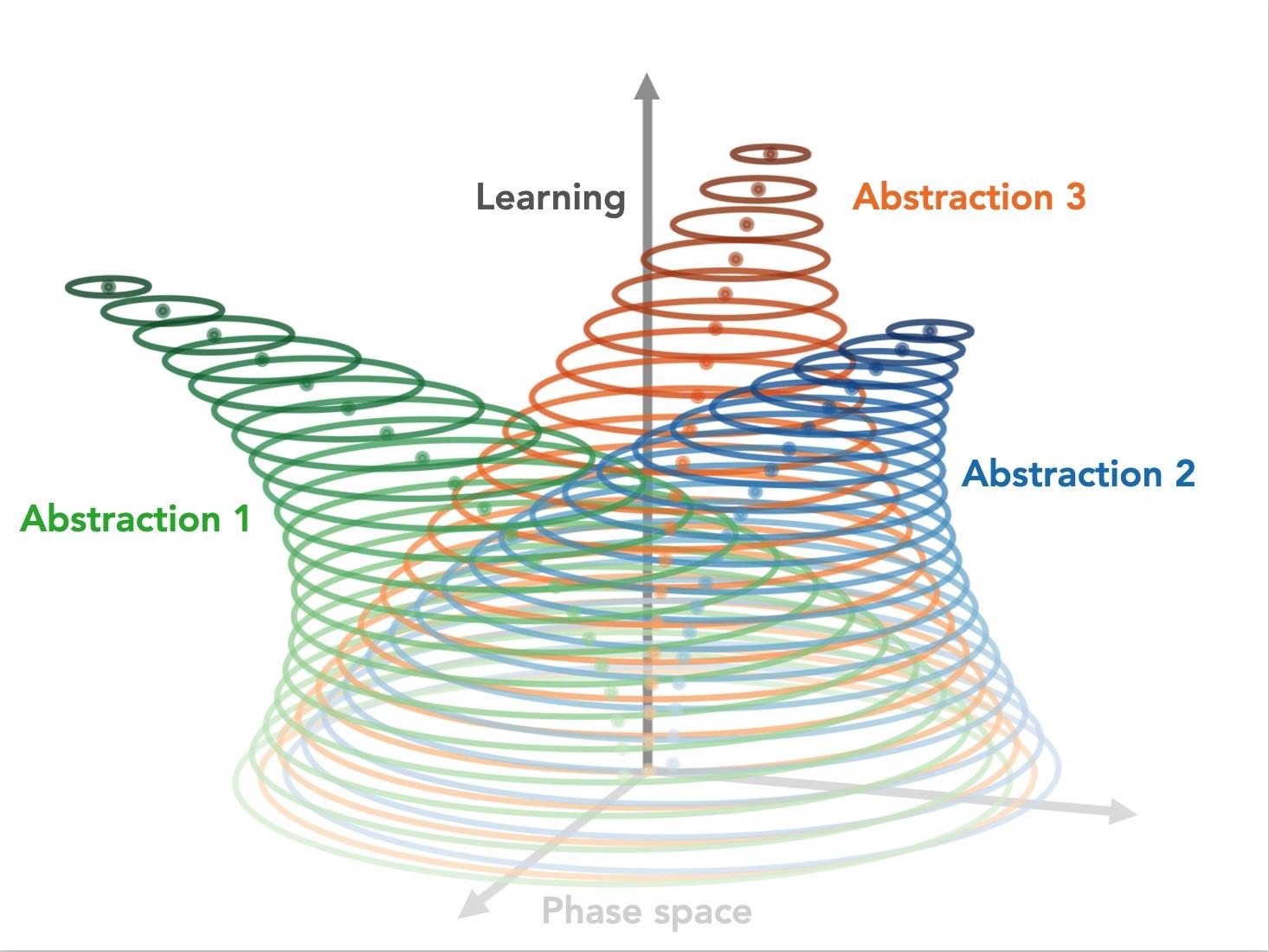
Biography:
Originally trained as an engineer in the early 2010s at the École Centrale de Nantes (France), Quentin Ferry went on to do an MSc. in Biomedical Engineering and Ph.D. in Molecular Biology at The University of Oxford (UK). His engineering work and research have spanned several academic fields, including machine learning, genetics, molecular and synthetic biology, genome engineering, and, more recently, neuroscience. Quentin is currently a postdoctoral researcher in the Tonegawa Lab at the Picower Institute of Learning Memory (MIT), where he, alongside other lab members, is attempting to shed light on the formation, storage, and generalization of knowledge in biological and artificial brains. He is the recipient of several distinctions and awards, including the Picower Postdoctoral Fellowship currently supporting his research.