Please note time of seminar has changed.
Abstract
A hallmark of intelligence is the ability to learn associations between causes and effects (e.g., environmental cues and associated rewards). The near consensus understanding of the last few decades is that animals learn cause-effect associations from errors in the prediction of the effect (e.g., a reward prediction error or RPE). This theory has been hugely influential in neuroscience as decades of evidence suggested that mesolimbic dopamine (DA)— known to be critical for associative learning—appears to signal RPE. Though some evidence questioned whether DA signals RPE, the RPE hypothesis remained the best explanation of learning because no other normative theory of learning explained experimental observations inconsistent with RPE while also capturing phenomena explained by RPE. My lab has recently provided such an alternative. Specifically, we proposed a new theory of associative learning (named ANCCR, read “anchor”) which postulates that animals learn associations by retrospectively identifying causes of meaningful effects such as rewards and that mesolimbic dopamine conveys that a current event is meaningful. The core idea is simple: you can learn to predict the future by retrodicting the past, and you retrodict the past only after meaningful events. Here, I will present the basic formulation of this theory, and present highly counterintuitive consequences of the theory in terms of learning rate dependence on reward rate—a set of predictions that allows non-trial-based mechanisms for few-shot learning. I will then present unpublished experimental results testing these predictions and demonstrating that behavioral and dopaminergic learning rates from cue-reward experiences are proportionally scaled by reward rate under a variety of experimental settings.
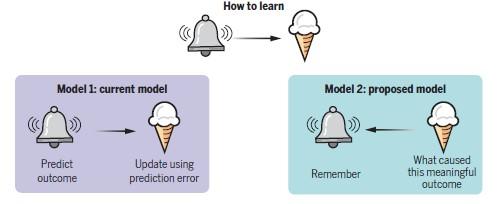
Biography
Dr. Namboodiri is an Assistant Professor of Neurology at the University of California, San Francisco. He is a member of the Neuroscience Graduate Program, Center for Integrative Neuroscience, and Kavli Institute for Fundamental Neuroscience at UCSF. His lab works on the biological algorithms and neural mechanisms underlying learning, memory, and decision-making. His lab has recently published an alternative conceptual framework of animal learning based on the retrospective learning of causes of meaningful effects.