Abstract:
Our motor outputs are constantly re-calibrated to adapt to systematic perturbations – we can learn to use new tools and often improve upon already learned motor skills. In this talk, I will first discuss my development of a mouse model of forelimb motor adaptation and experiments to probe the role of sensory and reward prediction errors in driving the adaptive behavior that was observed. Then, by systematically varying the task parameters, I will show that reward feedback defines the global incentive for a particular motor output (i.e. the goal), but does not provide trial-by-trial feedback that alters performance. To causally test which proprioceptive feedback pathways were required to adapt, I perturbed regions that receive feedback, namely cerebellar and cortical circuits. It was found that a closed-loop optogenetic photoinhibition of somatosensory cortex (S1) applied concurrently with the force field abolished adaptation (yet did not impair basic motor patterns or reward-based learning), which suggests that S1 is required to learn to adapt to forelimb perturbations. Next, to explore the neural circuits required for adaptation, we first built a deep learning toolbox for pose estimation (DeepLabCut) that allows us to perform high fidelity tracking of the mouse, and we now use this suite of behavioral and computational tools to study neural population dynamics across multiple regions of the brain during adaptation.
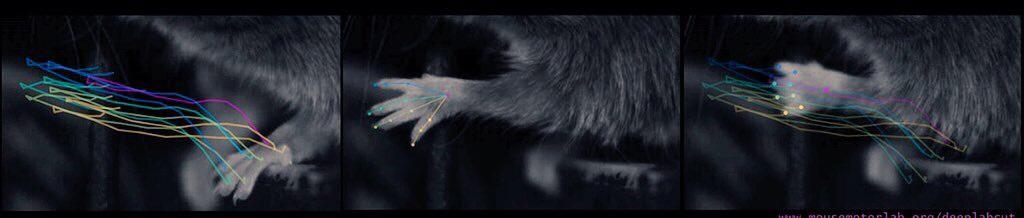
Biography:
Dr. Mackenzie Mathis is a Rowland Fellow at Harvard University. The lab studies adaptive motor behavior in mice, performs large-scale recordings of neural populations, builds new robotic tools for neural circuit interrogation & behavioral studies, as well as machine learning tools for behavioral analysis. Previously, she was a Postdoctoral Fellow with Prof. Matthias Bethge (University of Tübingen), and completed her PhD studies in March 2017 at Harvard University under the direction of Prof. Naoshige Uchida. Her thesis work was focused on uncovering the neural circuits and mechanisms underlying sensorimotor learning.