Abstract:
Hippocampal place fields show a variety of remapping phenomena in response to environmental changes. These phenomena get characterized in spatial terms such as object vector cells, landmark vector cells, distance coding, etc. But what if these myriad phenomena are side effects of mapping hippocampal neuronal responses on to Euclidean maps?
In this talk I will describe how treating space as a sequence can resolve many of the confusing phenomena that are ascribed to spatial remapping. Using our Clone-Structured Cognitive Graphs (CSCG) model [1], we’ll show how allocentric “spatial” representations naturally arise from higher-order sequence learning on ordinal egocentric sensory inputs, without making any Euclidean assumptions, and without having locations as an input. An organism can utilize CSCG for navigation, foraging, context-recognizing, etc. without ever having to compute place fields, or having to think about locations. A wide variety of spatial and temporal phenomena — splitter cells, boundary/landmark/object vector coding, event-specific representations, transitive learning and inference, partial remapping, rate remapping, varying sizes of place fields, place field repetition, etc. — have succinct mechanistic explanations in CSCG using the simple principle of latent higher-order sequence learning. Importantly, I’ll show how many of these observed phenomena are purely side effects of mapping neural responses to a Euclidean space, and describe why care should be taken to not over-interpret place field phenomena.
CSCGs can also offer explanations for schema formation, short-cut finding in novel environments, and different kinds of offline and online hippocampal replay. Overall latent sequence learning using graphical representations might provide a unifying framework for understanding hippocampal function, and could be a pathway for forming temporal and relational abstractions in artificial intelligence.
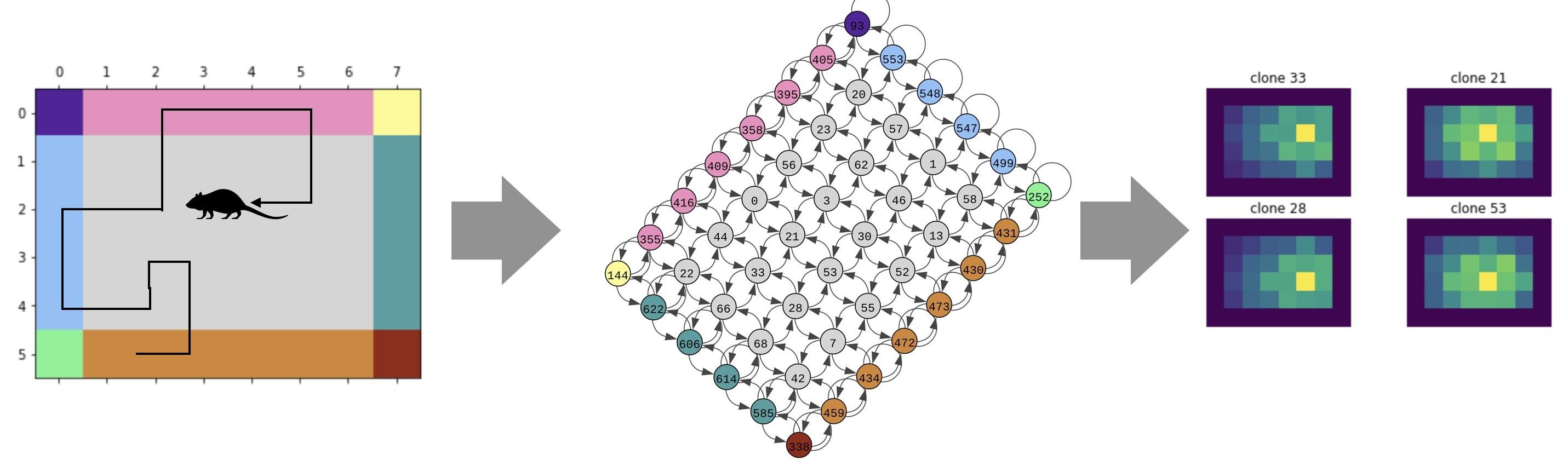
Biography:
Dr. Dileep George is an entrepreneur, scientist and engineer working on AI, robotics, and neuroscience. He co-founded two companies in AI — Numenta, and Vicarious. At Numenta he co-developed the theory of Hierarchical Temporal Memory with Jeff Hawkins. Vicarious, his second company, was a pioneer in AI for robotics, and developed neuroscience-inspired models for vision, mapping, and concept learning. Vicarious was recently acquired by Alphabet to accelerate their efforts in robotics and AGI. As part of the acquisition, Dr. George and a team of researchers joined DeepMind where he is currently at. Dr. George has an MS and Ph.D in Electrical Engineering from Stanford University, and a B.Tech in Electrical Engineering from IIT Bombay. Twitter: @dileeplearning Website: www.dileeplearning.com