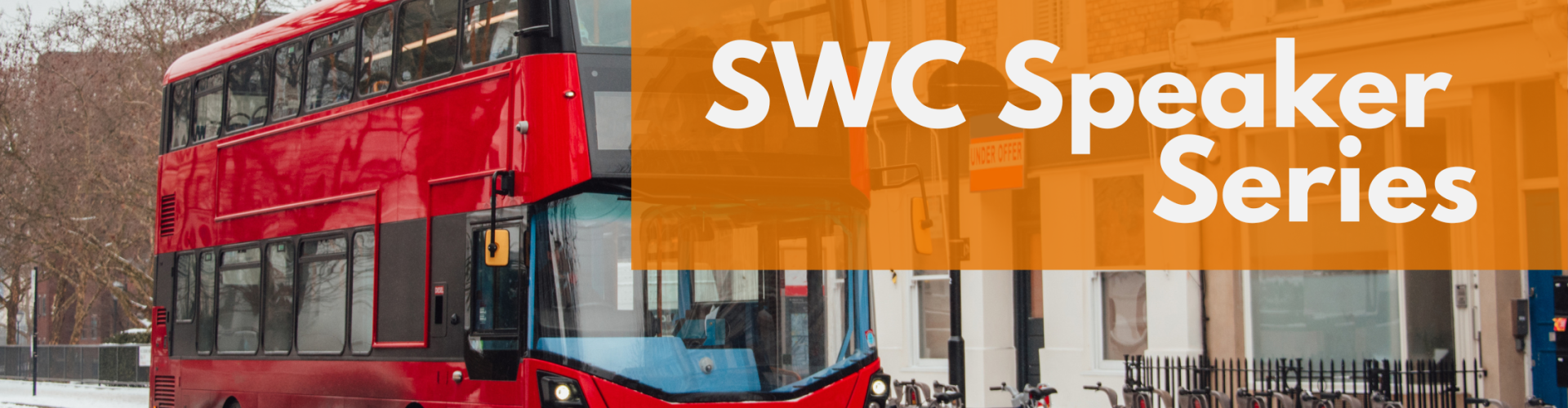
Exploring the maze of goal-directed behaviour
An interview with Dr P. Dylan Rich, Princeton University, conducted by Hyewon Kim
How do we get to the same grocery store using different routes in the face of obstacles, such as road works? We have a model of the neighbourhood from which we base our navigation decisions. In the third ENSS talk of 2023/24, Dr Dylan Rich shared his work on understanding model-based decision-making in rats using mazes.
In this Q&A, Dr Rich discusses historical roots of studying animal goal-directed behaviour, the design of experimental mazes, and the intriguing use of odour cues in learning paradigms. He also delves into potential game-changing implications of voluntary head restraint in neuroscience experiments, paving the way for future investigations into cognitive aging and beyond.
What prompted you to apply for the SWC Emerging Neuroscientists Seminar Series (ENSS)?
I was excited to share my research with a broader audience. Going to UCL as an undergraduate was a part of my journey as a scientist, particularly as a neuroscientist. I thought it would be fun to apply to give a talk back at UCL and visit the institute. People in the neuroscience community often talk about the Sainsbury Welcome Centre in London as a hub of great research; so I was very happy to get an excuse to visit.
How useful has the experience been?
It's been really good to meet with people and talk, get into more detail about the work. I thought the seminar atmosphere was great – It is always good to get some questions in the middle of the talk, for example. I also really enjoyed touring the building and meeting with the research community here.
What advice would you give to those considering applying to ENSS 2024/25?
I think the application process and format were nice. I liked the fact It was quite structured in terms of asking specific questions, which I enjoyed because it is different than just writing a longer research description. It’s definitely worth applying for.
How has goal-directed behaviour been historically studied?
Goal-directed behaviour has its roots in the study of animal psychology. As people became interested in what guides animal’s behaviour, two separate schools of thought emerged. First there were the behaviouralists, prominently B.F. Skinner, who pioneered this work using operant boxes and animals pressing levers. They believed that actions and behaviour could be explained solely as links between stimulus and actions. This approach has had a lot of merit, and many behaviours can be understood by it.
Concurrently, there was a move to understand behaviour from a more ethological perspective, considering animals as embodied agents that were actually thinking and planning in their environments. This was an understanding of behaviour pioneered by Edward Tollman, who coined the term cognitive map and used mazes and spatial problems to investigate behaviour.
Modern work on this, from computational, theoretical, and experimental perspectives, suggests that animals and people use actually use both types of strategies, as they each have different pros and cons.
Historically, goal-directed behaviour has been studied using paradigms such as devaluation. For instance, you might train an animal to press a lever to receive a certain reward like a chocolate pellet. Then, you would give the animal a drug that made it feel nauseous. Finally, you would present the lever to the animal and see if it would press it. These experiments were designed to test whether the animal was pressing the lever out of habit or because it wanted the chocolate. All of us are somewhat aware of these types of behaviours in our own lives, which become automatic if repeated often enough.
The work I've been trying to do is to take these ideas and instead of doing one-off experiments with devaluation procedures, to do multiple instances of learning within the same animal in the same session, to understand how the algorithms that the brain solves these problems.
Why did you design the maze the way that you did?
The maze was designed to be the simplest possible maze that would allow us to detect if an animal was relying on a goal-direct behavioural strategy. It's analogous to thinking about how we navigate in a city, for instance, from one place to another, like from your home to your work. Under what conditions are you able to learn short cuts? Or can you take a new route if you know there is a road-block on your normal route.
In my seminar I used the example of commuting to UCL when I lived in Camden as an undergraduate student. I would normally take the number 29 bus, but one occasion three consecutive buses were full and I was going to be late for a lecture! However, I was able to plan a new route (taking the northern line tube), despite never having taken that particular door-to-door option before.
In the real world navigating a city or town is very complex and uncontrolled. We wanted to simulate that type of experience in the lab and reduce it to its simplest format, which would still provide enough complexity for animals to make navigational decisions.
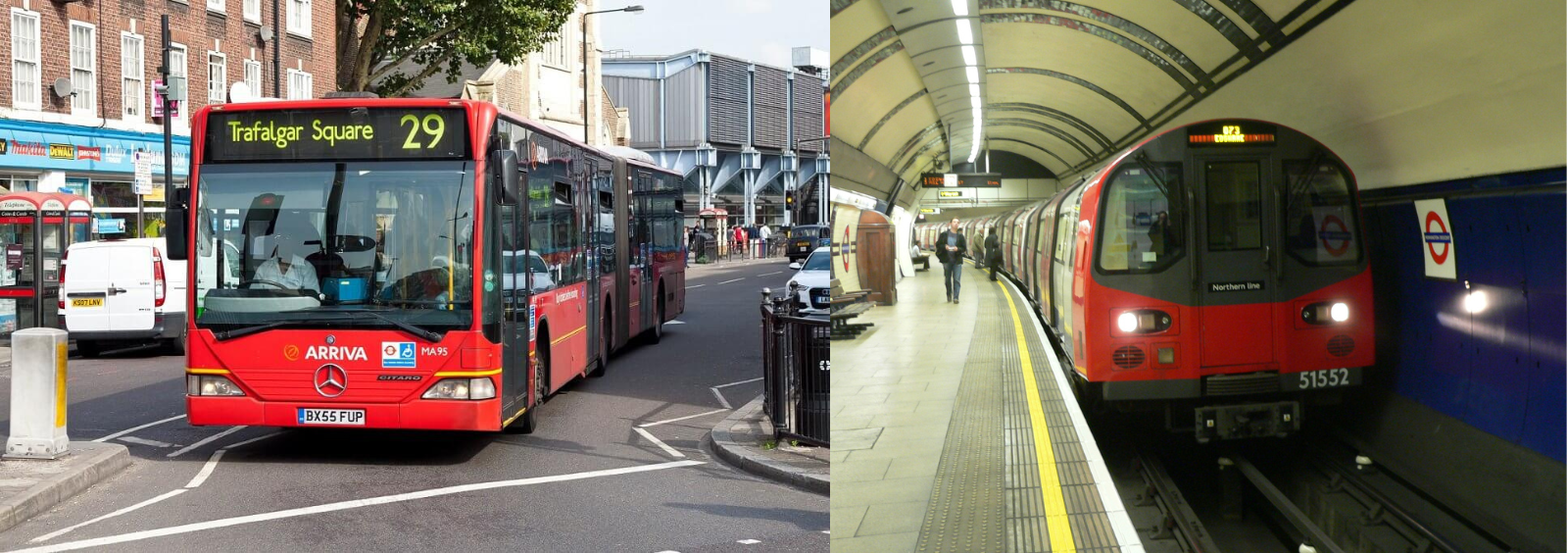
Is there a reason why you used odour cues rather than any other sensory cue, like sound?
There's an interesting phenomenon whereby animals, especially rats and mice, seem to learn better with odours than with sounds or lights. This has been investigated in psychology literature to some extent. One explanation is that rats are more olfactory-based animals and pay more attention to odours. However, we think this is not the whole story, as animals can still perform visual and auditory discrimination tasks.
There seems to be an extra benefit or extra abilities that animals have when learning from different odours, and some learning capacities in rodents have only been demonstrated using odours, such as fast learning from single examples or adapting to new configurations. When looking at the literature of complex cognitive tasks in rodents, the stimuli used are almost invariably odorants. We don't fully understand why this is, but it may be related to the special role that the olfactory sense has in the naturalistic behaviour of these animals.
Another reason to use odours is that there are a lot of them and they are quite distinct! Early on in the development of the experiment, I tried using jelly beans as odorants, since they were easy to get hold of, they were shelf-stable and had consistent odours. Eventually I ended up using a combination of chemical odours and spices since it turns out jelly beans don’t have that strong an odour!
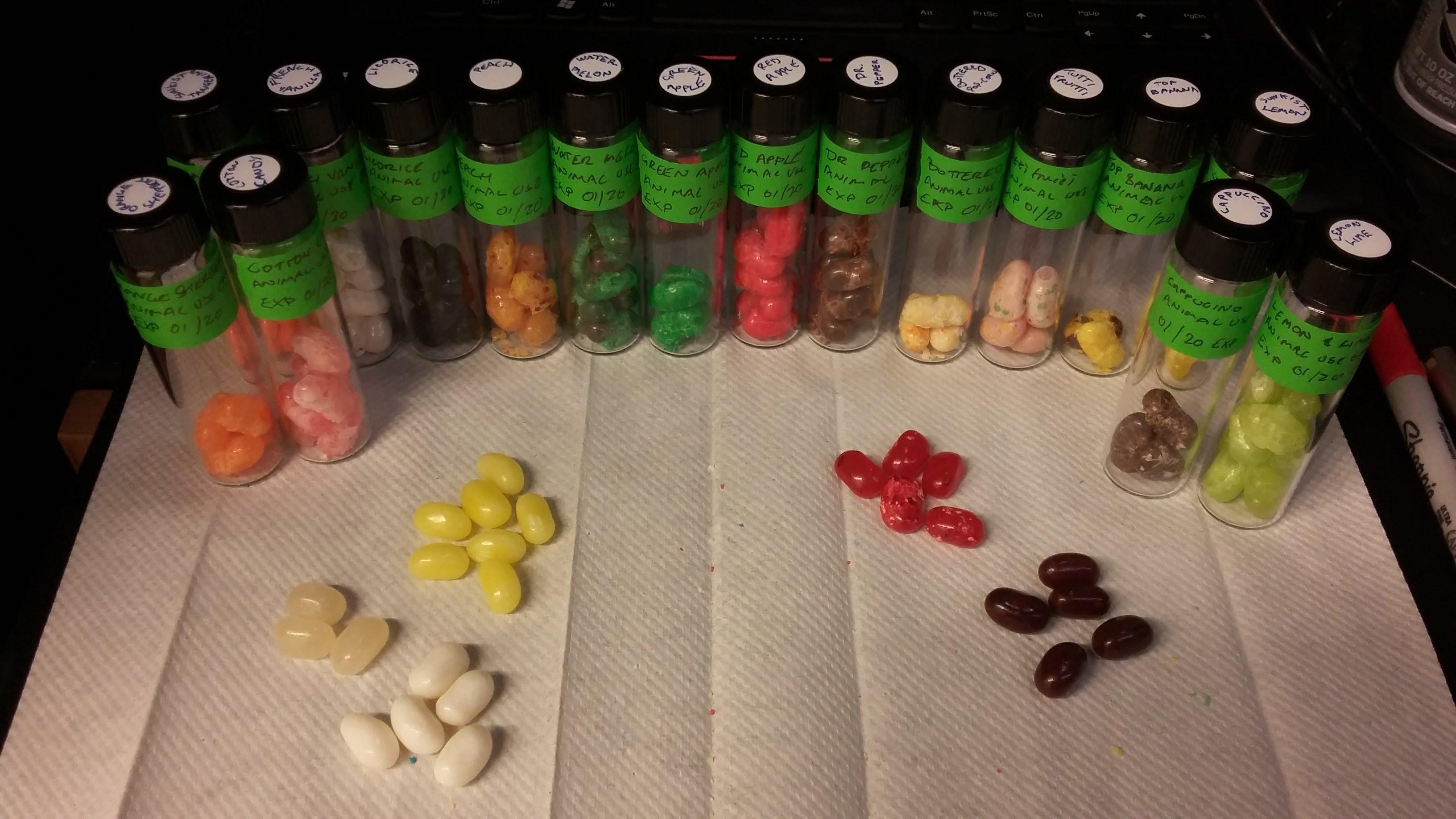
How did you involve the hippocampus in the design, excluding possible confounding effects?
When an animal is moving around in an environment, cells in the hippocampus fire in different areas of space. These cells, originally discovered by faculty member at SWC, John O'Keefe, are termed place cells and are thought to be the basis of a cognitive map that enables flexible goal-directed navigation. The discovery of place cells, and the subsequent research around them has been hugely influential in neuroscience.
However, there is increasing evidence in recent years that, in addition to spatial coding, the hippocampus also encodes other types of stimuli and different modalities. A significant challenge in this research area is accounting for the hippocampus's strong spatial associations when examining its responses to different modalities, such as odours or sounds. It's crucial to consider the animal's spatial position to ensure that observed changes in neural activity are attributable to the stimuli themselves, rather than to movements of the head. Various approached have been adopted to address this issue; In my experiments, I used a new technique I developed, magnetic voluntary head-fixation to ensure the animal's head is in a same position, down to the micron, from trial-to-trial.
Why do you think magnetic voluntary head-fixation could be game-changing going forward?
I think it allows for the type of experiments that are currently unavailable. It brings together two different domains of systems neuroscience experiments. One involves animals that are head-fixed under a microscope. Here we can use the best, state of the art equipment for recording or stimulating, and build as much hardware as we want above the animal to peer into its brain.
The other style of experiment involves animals freely running around and expressing their natural behavioural repertoire. However, there are more constraints on how we can record and perturb the brain in this case, such as needing cables or wireless equipment, or limitations on what the animal can carry on its head.
By using this new technique, magnetic voluntary head-fixation, we can use the latest optical tools of available, but still allow animals to express complex spatial behaviour.
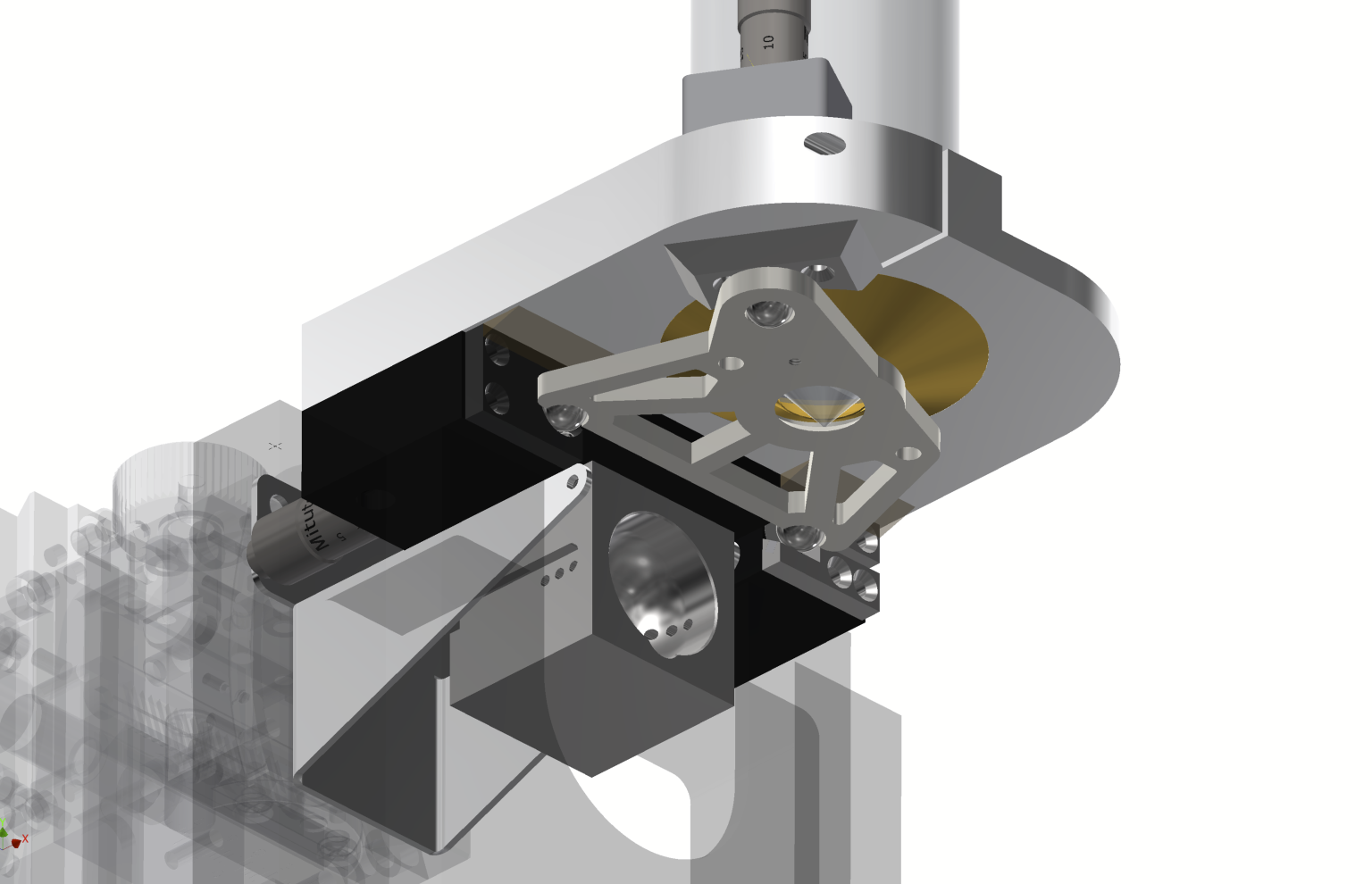
Were you surprised to find that rats showed signatures of goal-directed behaviour?
We weren't surprised to find that rats show these signatures because it has been established with other experiments that animals can use these behavioural strategies. Traditionally, demonstrations of these cognitive abilities have been in one-off experiments where the animal gets one chance to demonstrate its knowledge. We were trying to create a task that required continual learning over the course of a whole session, and still see evidence of goal-directed decision making. One parameter that seems to encourage animals to become more habitual (and less goal-directed) is repetition. Often, animals resort to more habitual strategies in such scenarios, but we were trying to encourage more deliberative behaviour while still maintaining an experiment with many trials for analysing the neural data.
How do these neural dynamics found in the data support model-based decision-making?
One of the most striking features when we looked at the data was the non-stationarity across the whole session. Traditionally, there's been an assumption about neural responses that if you present the same stimuli to the animal on one trial and then present the same stimuli on another trial, the response in the brain will be essentially the same, subject to some stochastic variability.
However, what we found is that neurons change their responses over longer time periods, such as minutes or hours. When you come back and repeat the same stimuli, the brain's response is different. This result fits into a growing literature on the phenomenon known as representational drift, whereby the neural representation changes over time. An open question that many people are wondering right now is what is the function of representational drift? We found evidence that in our experiments, drift is modulated by the animals' learning in this task and seems to be related to the specific goal-directed, model-based type of learning the animals are engaged in.
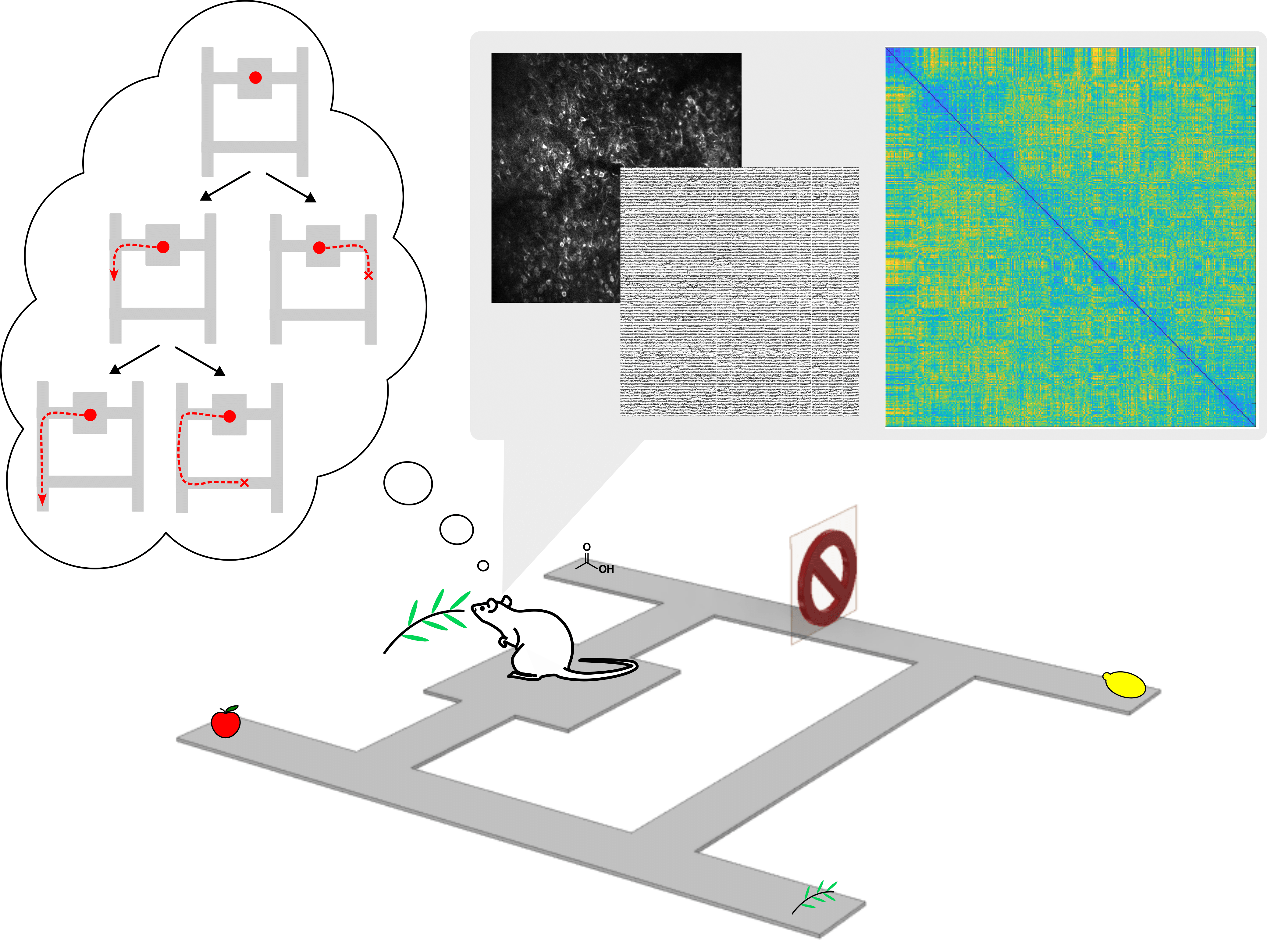
How did you build reinforcement learning models of behaviour?
Reinforcement learning is a powerful and flexible framework for understanding many types of behaviour. The central question that RL addresses is how does an agent explore and operate in the world to maximize rewards?
You can think of an RL model as the kind of instruction you'd need to programme a computer agent or robot. The robot may only have access to certain types of information, such as the action it has just taken, and if it received any reward, so your model will have to account for that. When we are building a model, we have to make certain choices including things like its architecture and also the values of certain parameters, such as the learning rate. We then want to find the best model with the best parameters that matches what we see in the animal's behaviour. This becomes a mathematical and statistical problem to work out, and there are many approaches to doing this.
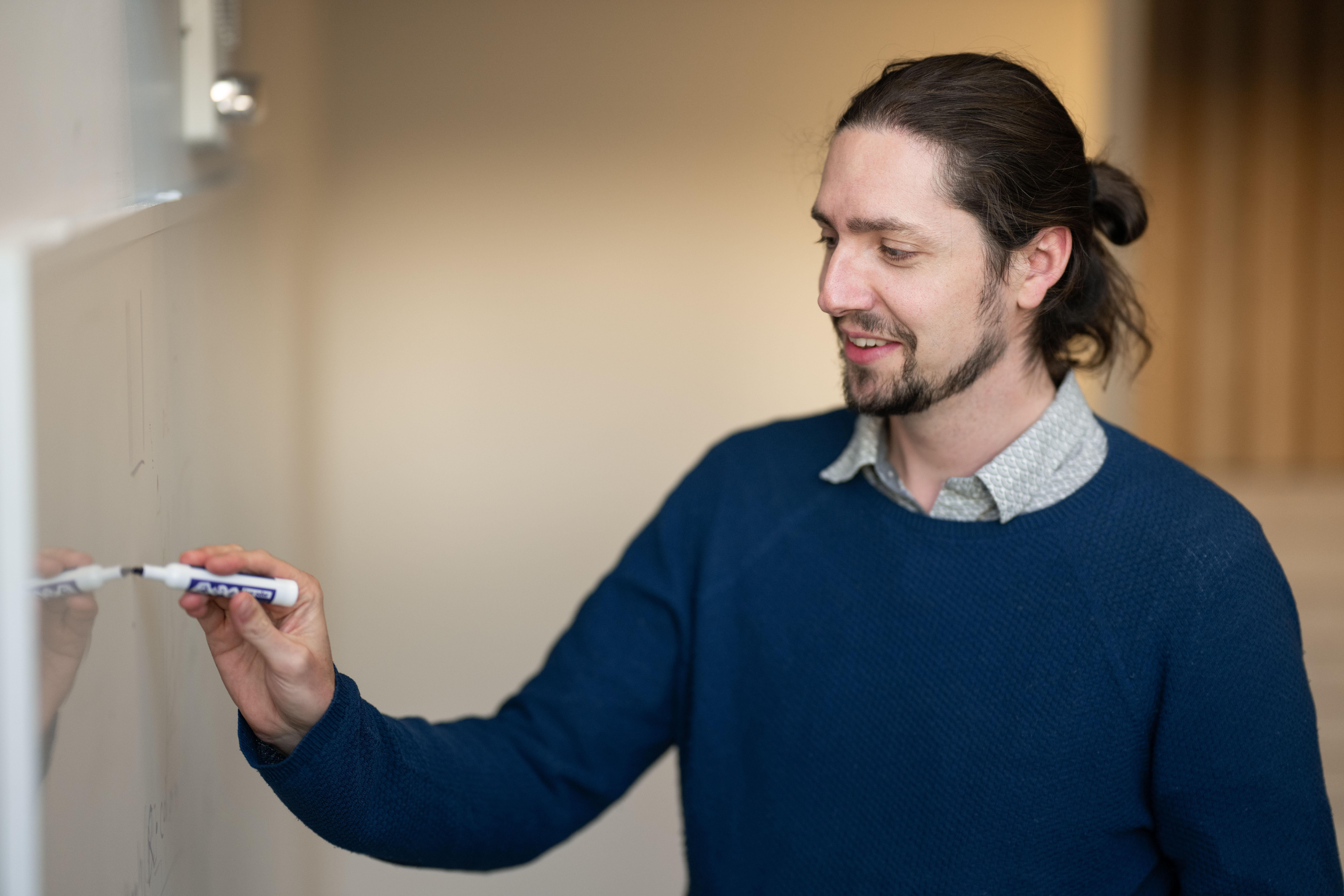
What are some next steps you’re excited about, including or beyond long-term voluntary calcium imaging?
In the last 50 years, neuroscience has primarily focused on understanding cognition and brain function by looked at young or adult animals. However, one of the largest challenges that society is facing right now is cognitive and neurophysiological difficulties that arise with age. This includes specific pathologies like Alzheimer's disease or nonspecific cognitive decline experienced by many as they grow older. Understanding how the brain, and neurons change during aging and relating those changes to cognition will be crucial in beginning to better understand the aging process and eventually help people with age-related cognitive decline.
Voluntary magnetic head-fixation provides the ability to record from the same neurons throughout an animal's life while continually assessing its learning ability. Relating these two things can shed light on how memory, learning, and cognitive abilities change over time. This is a totally new type of data that has not be available before for understanding of how the brain works. The knowledge gained from these types of long-term experiments have a huge potential to transform the understanding and treatment of age-related cognitive issues that many of us already or will eventually face.
About Dr Dylan Rich
Dylan started his education at UCL on the undergraduate program in Neuroscience where he performed behavioural experiments in the Lab of Jennifer Linden. The first year of his joint PhD program was spent in the lab of Jeff Dalley at the University of Cambridge working with novel wireless electrophysiology. He completed his PhD at Janelia Research Campus in the USA where he worked in the Lab of Albert Lee. He discovered a novel principle of how the hippocampus encodes large naturalistic spaces, and was part of the collaboration that developed the first generation of NeuroPixel probes. Dylan is currently a post-doc in the labs of Nathaniel Daw and David Tank at Princeton university, where he has developed new techniques for two-photon calcium imaging in rats and is using them to study the neural basis of flexible decision making in the hippocampus.