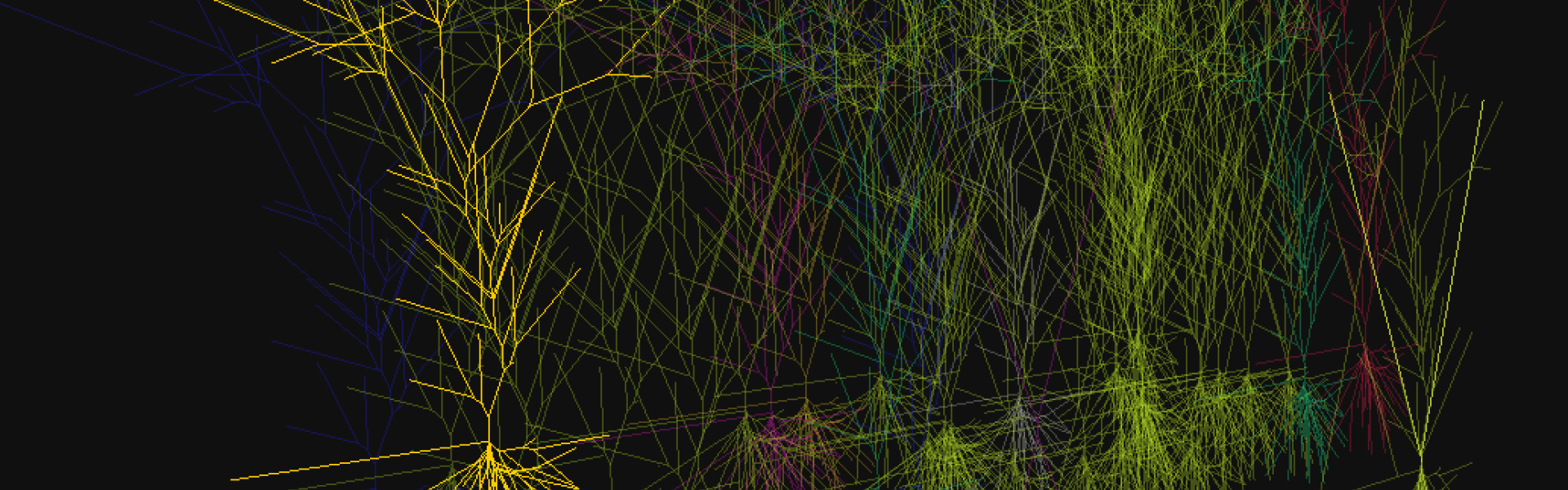
The hippocampus: a predictive map?
An interview with Kim Stachenfeld, PhD, Research Scientist, DeepMind, conducted by April Cashin-Garbutt, MA (Cantab)
Groundbreaking research has established that neurons in the hippocampus encode a spatial representation of the environment, but clinical observations and additional evidence indicate that this brain region can process non-spatial, episodic information. In the following interview, Kim Stachenfeld, a Research Scientist at DeepMind, outlines how a representation learning approach has shown that the hippocampus may act as a predictive map for more general sorts of information.
What is representation learning and why is it a useful idea to neuroscientists?
Representation learning refers to the practice of learning to encode features that are relevant to a set of tasks that you care about. It is useful for reinforcement learning, as often there can be a lot of features that are not relevant that can be filtered out. In essence, the problem is filtering stimuli for relevant information and then conducting learning in the space of these relevant representations.
By limiting learning to only relevant information, we can learn a lot faster. There are a lot of situations in the real world in which reinforcement learning on unprocessed stimuli would progress prohibitively slowly. For instance, if you only see rewards every once in a while, then you really need to have a sense of what causes those rewards in order to inform your actions.
I think representation learning is particularly useful for neuroscience because, like reinforcement learning, it gives us a normative framework to interpret brain representations. We can interpret them in terms of what these representations should be useful for, and how they might relate to an animal’s goals.
Representation learning lets you break down the reinforcement learning problem into parts. You have the problem of learning to maximize reward, and then the problem of learning the relevant features that can make that reward learning go faster. So it basically makes the normative scaffolding of reinforcement learning a little bit denser.
We have more things that we can anchor our understanding of neural representations to, because we’ve taken this reinforcement learning problem and expanded it into a sub-problem, which is finding a good representation to make this learning work better.
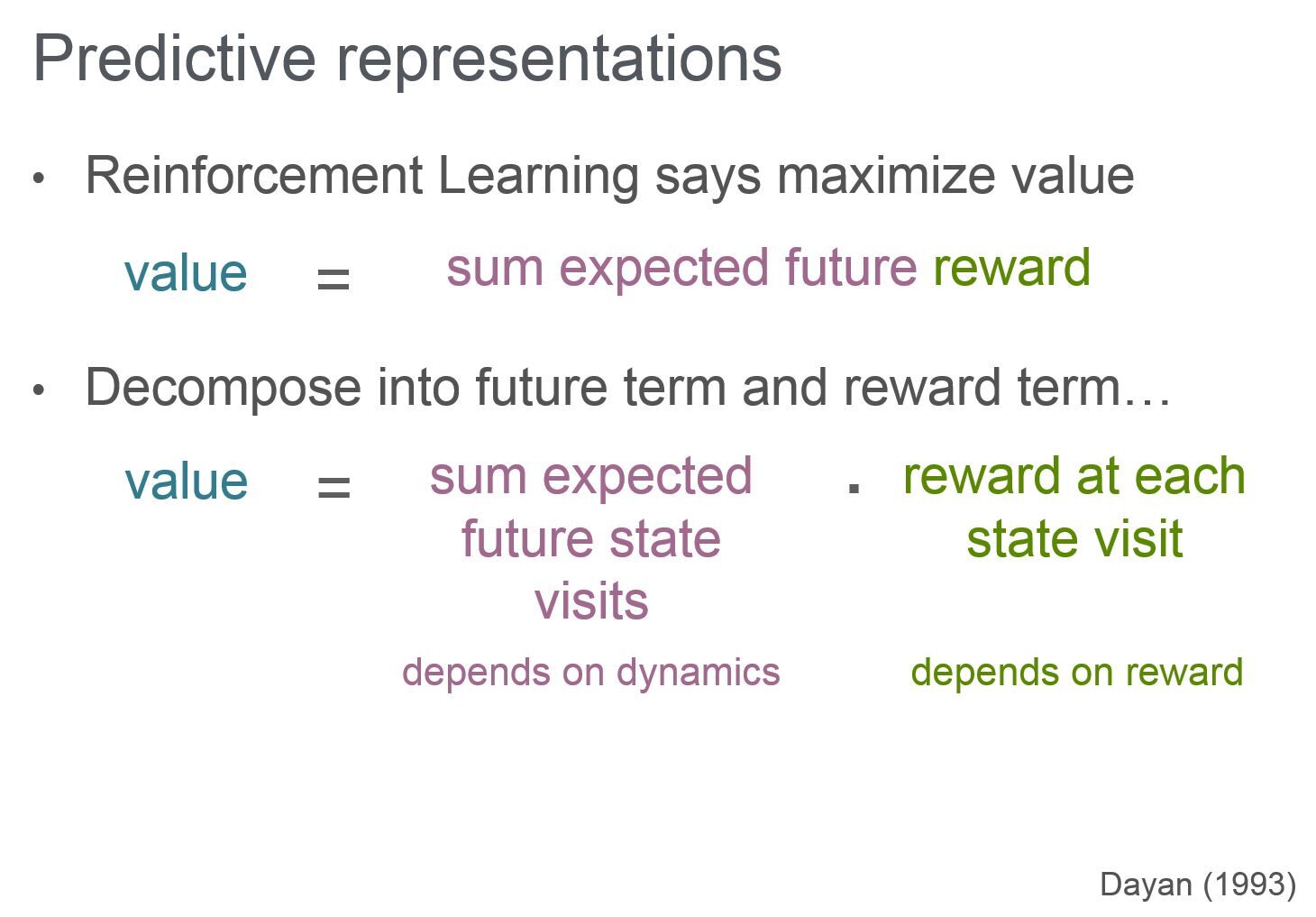
How does the brain work out which features are relevant?
That’s the question! With end-to-end reinforcement learning, you don’t have to make any additional decisions about what’s important, whereas with representation learning, you have to make decisions.
For example, a potential representation learning objective might be to learn to classify the objects you are looking at. You don’t get rewarded directly for knowing that a cup is a cup, for example, but knowing what a cup is can help you solve other problems that are more directly rewarding (for instance, preparing tea).
How are you using a representation learning approach to develop a model of hippocampal representations?
In this project we are trying to understand how hippocampal representations are useful for the downstream learning processes that depend on hippocampus. If the animal wants to compute how rewarding some future action is, what representation might the hippocampus be providing that would make this computation a lot easier and faster? How would this representation relate to data about the hippocampus and how does experience modify the place representations in hippocampus?
To do this, we drew on Peter Dayan’s idea of using the successor representation to break down the reinforcement learning problem. This takes the problem of estimating your expected cumulative future reward, and breaks it into two problems:
- estimating which locations (or “states”) you’re going to visit in the environment, and how often (a “successor representation”)
- estimating how much reward you’re going to get at each of these states
The first term, i.e. how much am I going to visit every state in the environment, is a predictive representation because you are asking what’s going to happen in the future, on average? The second term, learning how immediately rewarding each state is relatively easy. You just need to visit each state once, rather than keeping track of all future rewards. Thus, once you have a successor representation, learning in the the space of this predictive representation can be a lot easier than learning to estimate expected cumulative reward directly.
It also is more flexible, because if the reward changes you only need to change the reward term. The term that keeps information about predictions can stay, mostly, the same.
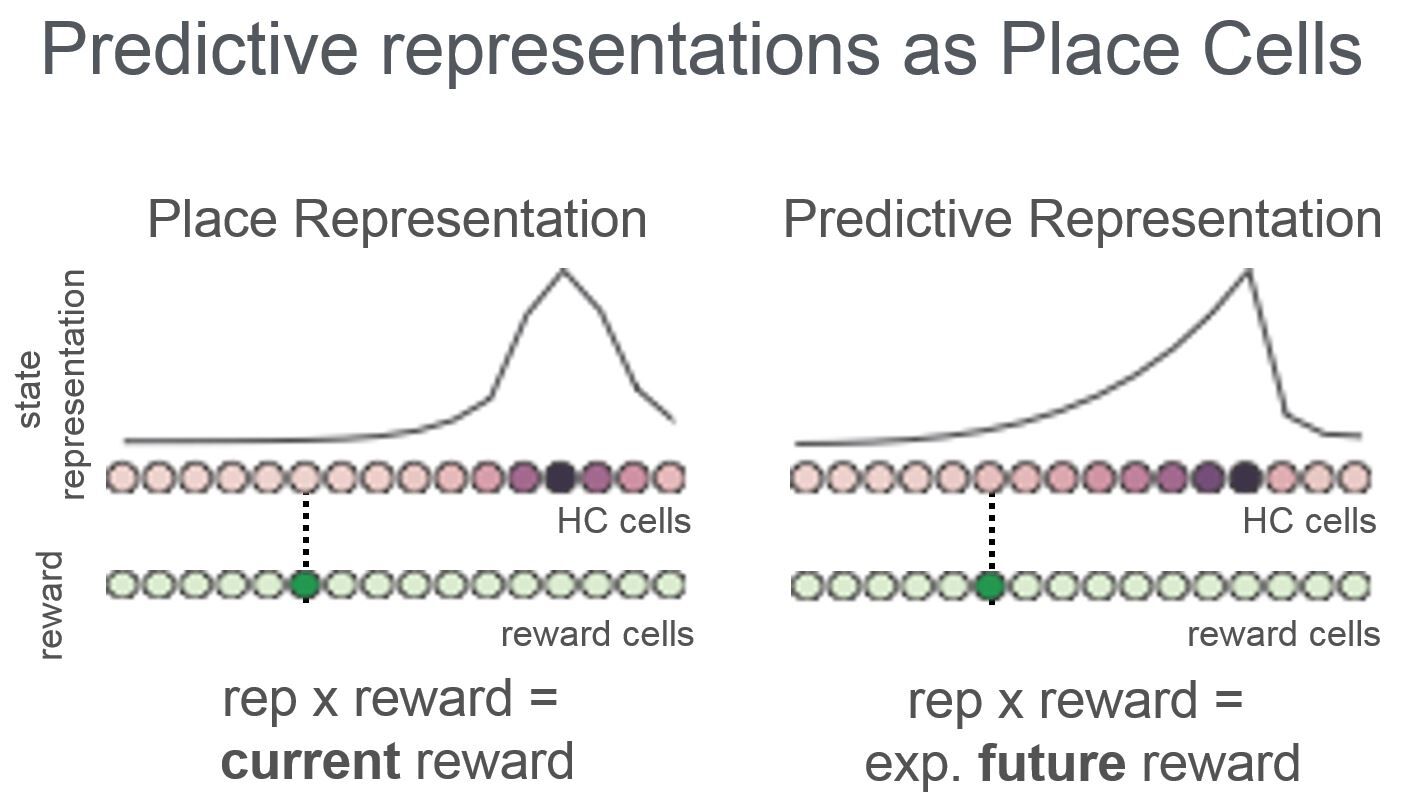
What evidence is there to suggest that representation in the hippocampus is not purely spatial?
There are a few categories of evidence. One is that the hippocampus will encode your state, which is an abstract position, in tasks that are non-spatial as well as spatial. This is by no means comprehensive, just a few things that were easy for me to think of.
Recent research by Dmitriy Aronov, Rhino Nevers and David Tank showed that the hippocampus could represent the pitch of a stimulus when it was task relevant. In the experiment, animals had to navigate to the target tone by pressing a lever to change the tone and releasing when the tone was at the target. Just like you find hippocampal representation for location in spatial tasks, Aronov et al. found hippocampal representations of tone, in this tone navigation task.
There have also been reports of time cells, that Howard Eichenbaum found a while ago, that encode time in a way similar to how hippocampus encodes space.
Also there are a lot of behaviourally-dependent modifications that happen to the place cell representations with experience. For example, when the animal is first brought into the environment, firing of place cells mostly depends on location, but as the animal gains experience the representations are modified. For instance, place cell splitting can occur, which is where a cell that used to fire on multiple routes will only fire on one route.
In addition, there is also backwards expansion of place cells, where place cells will start off firing near one location regardless of direction, but then as the animal travels more and more in one direction, they will start to skew backwards, opposite the direction of travel. This effect is key to the modelling we did in our paper.
So there are a couple of these experience dependent manipulations that happen on top of the place cell representation, even in spatial navigation tasks.
In the cognitive neuroscience field, a lot of research has focused on the hippocampus’ role in a variety of nonspatial roles, including episodic recall, and memory for narratives, and autobiographical memory and constructive imagination.
How have you shown that the hippocampus acts as a predictive map?
We showed that this predictive map model can describe a lot of deviations of the hippocampal representations from being a purely spatial representation. The model captures the backwards skewing of place cells, in particular, because after an animal has travelled in one direction along the track a lot, then upcoming locations can be predicted further in advance, so this predicts that the place cells should skew backwards.
The predictive map model also predicts how boundaries and obstacles should interact with place cells, and how travelling in a consistent direction should. It also makes predictions about how clustering of the environments should affect place cells. For instance, if a bunch of states are connected in the cluster, then they’ll tend to predict each other a lot. If you’re moving around, you’re a likely to remain in the same cluster, and it is only a rare event that takes you out of the cluster.
The predictive map captures this expansion of place fields within a cluster, but not outside of that. It also works for spatial and non-spatial environments, and unifies findings across the board.
What are place cells and grid cells thought to encode in this predictive map?
Place cells are thought to encode the successor representation, which means that each place cell will have some state that it encodes, and its firing will be proportional to the expected discounted number of times that its state will be visited in the future, given the animal’s current state. In essence, given where I currently am, how much am I going to visit the state that the cell cares about?
The grid cells are, in our model, forming a compact representation of the predictive map. So we encode this by having each grid cell encode one eigenvector of the successor representation matrix. Basically, that’s saying that each grid cell is participating in this more compressed representation of what the hippocampal cells are encoding and that this captures large scale statistics about the environment, because it ends up being more efficient.
You can encode the predictive map more efficiently if you encode coarse grained statistics in some eigenvectors, and then increasingly fine grained statistics in the consecutive eigenvectors. If you order your information according to how important it is to reconstructing the predictive map, this lets you cut it off earlier and not lose that much information.
Where can readers find further information on your work?
DeepMind published a blog post on our work a while ago, which has a great summary: https://deepmind.com/blog/hippocampus-predictive-map/
About Kim Stachenfeld, PhD
Kim Stachenfeld is a research scientist on DeepMind’s Neuroscience team. Her main focus is on representations to support efficient reinforcement learning and planning and she works on both neuroscience and machine learning problems in that space.
Research interests include hippocampus and entorhinal cortex, reinforcement learning (deep or otherwise), efficient representations for reinforcement learning, and on good days, fMRI.
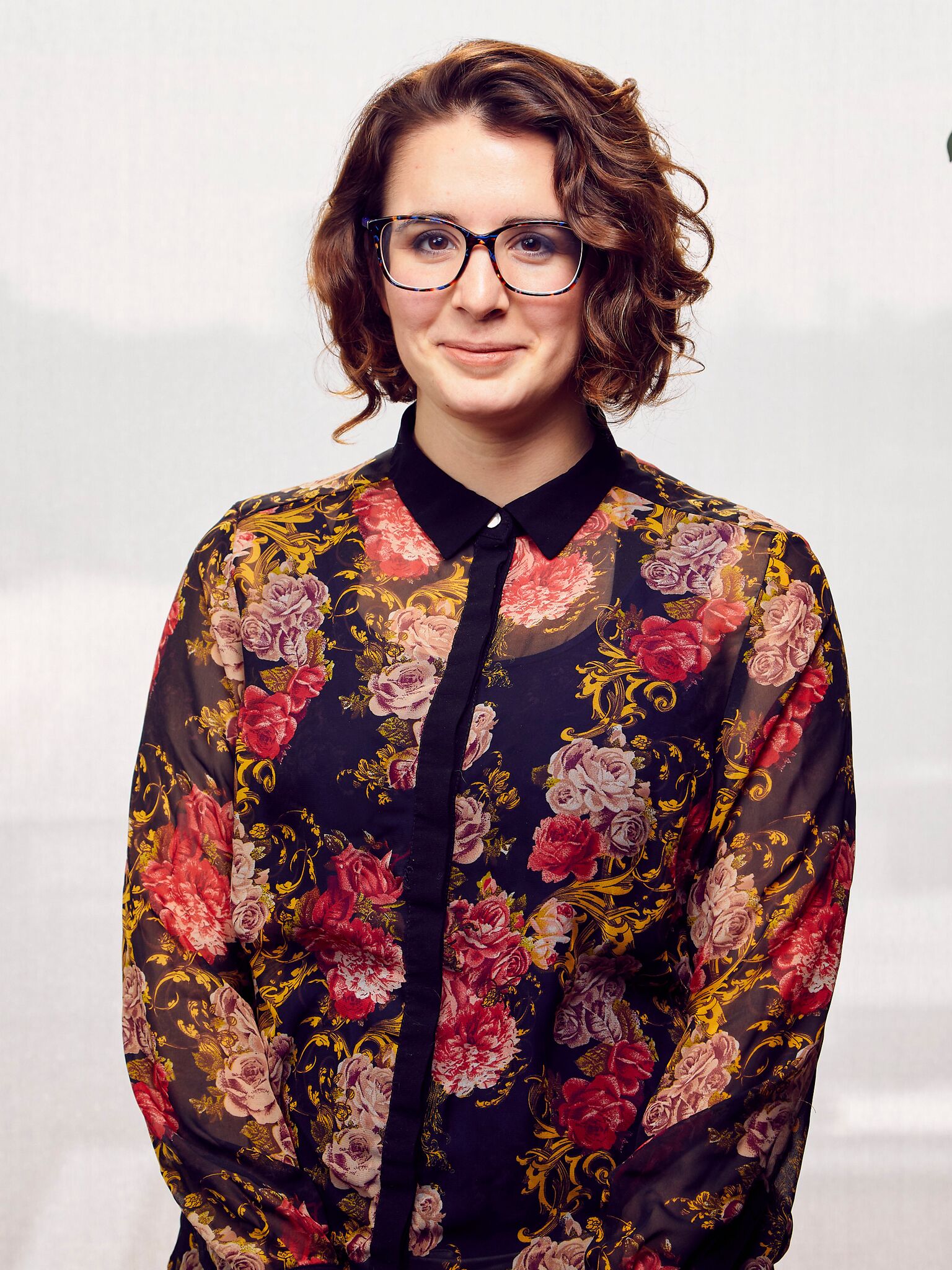