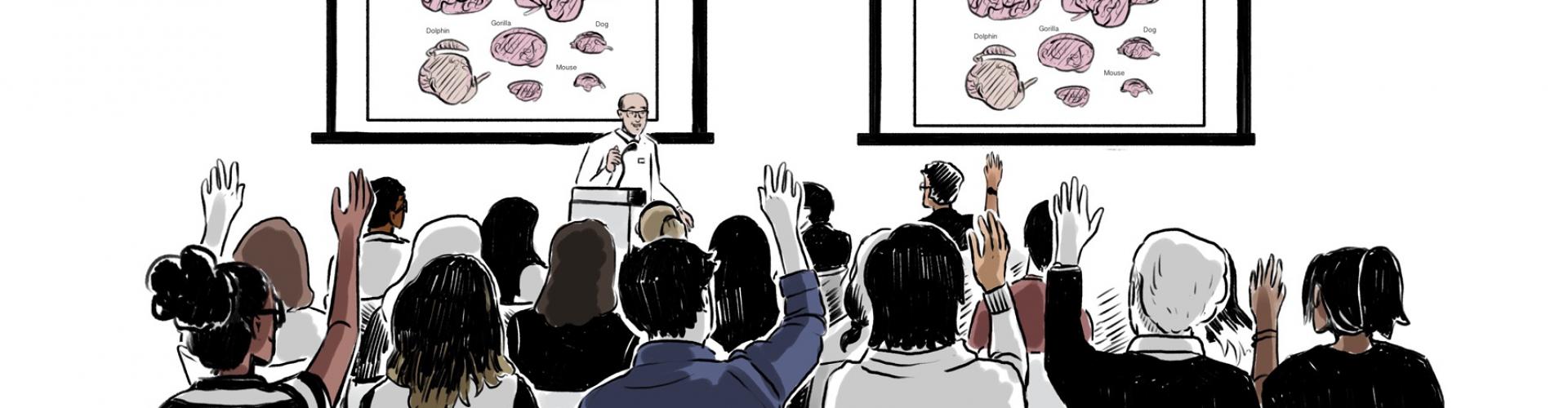
Learning rapidly from limited experience
An interview with Dr Thomas Akam, Department of Experimental Psychology, Oxford University, conducted by April Cashin-Garbutt, MA (Cantab)
How do internal models help humans and other animals learn rapidly and from limited experience?
Much of our day-to-day behaviour is only possible because we have rich internal models that allow us to make strong predictions about what is likely to happen next. Such rich world models are critical for allowing us to behave flexibly.
When something unexpected happens, for example you get to the train station and it is closed, you are very quickly able to find another solution by searching your internal model. Thus we are able to infer the consequences of new information and we don’t have to learn by trial and error for each situation.
We have done some experiments with humans where we presented people with simple learning tasks without any context. I was amazed at how poor humans were at learning what happens next in these tasks and I think that reflects the fact that we have such rich prior knowledge about how things behave, which is what allows us to make predictions in daily life. We are only able to behave flexibly by bringing to bear an immense amount of prior knowledge about how the world works.
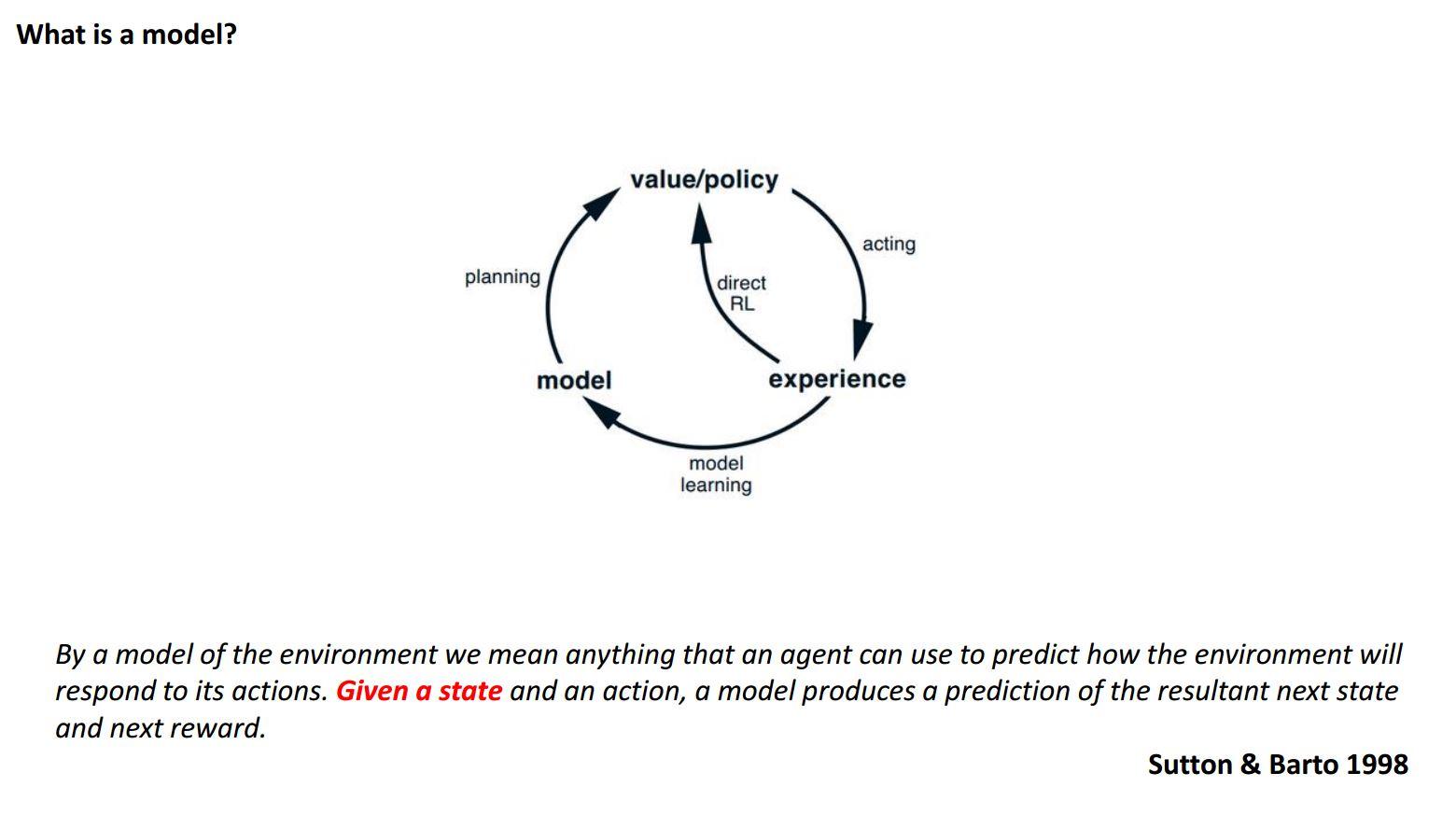
What first sparked your interest in studying planning and generalisation in model-based decision making?
I first worked on behavioural neuroscience during a PhD rotation at UCL with Ray Dolan. During the three-month rotation, I was exposed to the idea that there are multiple decision-making systems in the brain. There are habitual actions that can be thought of as mappings from a particular situation to a particular response. For example, when you tie your shoelace you don’t think in detail about the consequences of all the steps, it is just a sequence that you know how to do and you execute it very quickly. By contrast, there are other actions that are much more deliberative and they involve thinking through the consequences of the action.
I was really inspired by a theoretical paper by Nathaniel Daw, Yael Niv and Peter Dayan where they framed this distinction between actions and habits in a computational framework. I thought this was a really interesting quantitative perspective on this aspect of behaviour. While I ended-up doing my PhD on something completely different, these ideas were planted in the back of my mind and when I was thinking about what I wanted to do as a postdoc, I knew I wanted to come back to these questions about behaviour as they were interesting, poorly understood and seemingly tractable.
What are the main challenges in studying planning?
A big challenge is that planning is only one out of a number of different strategies that humans and other animals use. Imagine you are trying to navigate from a train station to a certain location. You might plan a route by using a mental map of the different streets, or you might use a much simpler model by imagining the relative location of the places not using the details of the streets but just knowing the direction that you need to go in. The latter is another kind of model but it is not the step-by-step planning that I am most interested in. Also, if it is a route you have done lots of times, you might just take the same route you always take, i.e. you might solve the problem habitually.
So one of the main challenges in trying to understand mechanistically how planning works is that you need to be able to isolate the contribution that planning is making to the behaviour from the contribution of other action selection systems. In experimental psychology there have been a lot tasks developed that do this in very beautiful ways, however, you often only get a very small number of informative trials.
To give an example, you can train rats to press one lever to get chocolate milk and another lever to get sugar pellets. Then if you were to pre-feed the rat before the test session with lots of chocolate milk, so it doesn’t want the milk anymore, then during the task the animal will favour the lever for the sugar pellets, because it is satiated on chocolate milk. This shows you that the animal specifically knows that one lever gives chocolate milk and the other gives sugar pellets. It is a rudimentary kind of planning, but it shows that the brain knows the action has a specific consequence, not just that the action is “good”.
These behavioural tasks are very elegant for demonstrating these rudimentary planning mechanisms exist, but they are not very good for looking at brain mechanisms. In order to show that they are using online prediction you have to withhold the rewarding outcome during the test sessions. This means that you only get a handful of trials as the rats quickly realise that the lever isn’t doing anything so they stop doing anything.
A large part of what I have been trying to do for the last few years is to develop tasks where we can show the contribution of planning to the behaviour, but also get the animals to make many decisions in one behavioural session so that we can really understand, in a very quantitative way, the relationship between brain activity and behaviour.
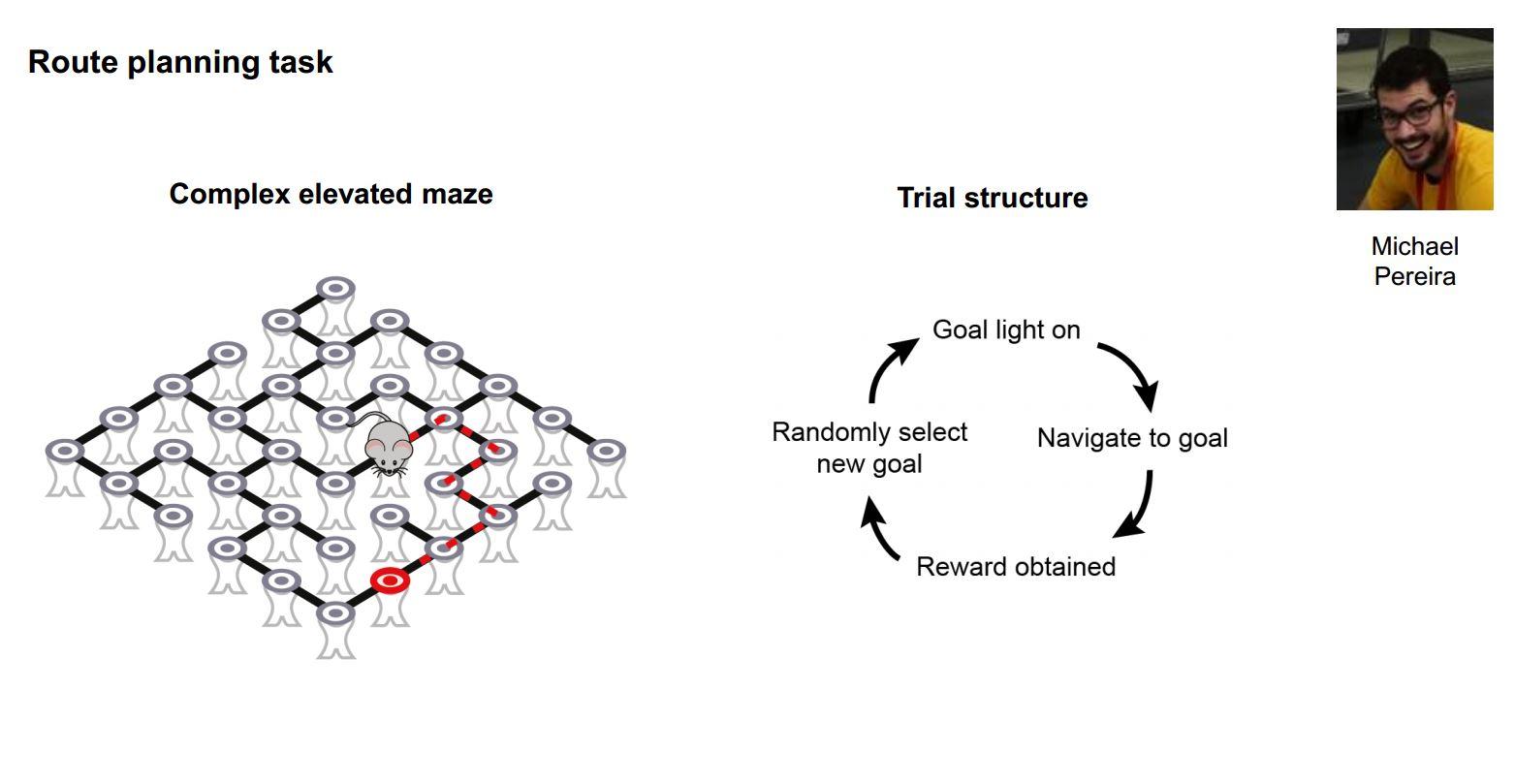
Do mice show behaviour consistent with planning?
Yes absolutely. We have demonstrated this in our current work where we place mice in complex elevated mazes and cue one of many possible goal locations by turning on a light. When the mouse reaches the goal they get a reward, we randomly cue another location and so on. By looking at the sequence of choices they make, we can show that they have a map of the maze in their head and are planning a route, i.e. when the shortest path to reward requires taking a route that is not directly in the direction of the visible goal they prefer the shortest path. So we can show that the mice are doing something that looks a lot like route planning using a mental model of the maze structure.
So I think there is really clear evidence that mice have predictive models of the world, which help them make good decisions. Those models are massively less rich than the kinds of models that humans have, but what we hope is that many of the principles of how those mechanisms work in the mouse are going to be the same in humans.
However, the expressive capacity of the human model of the world is so much bigger, and that partly reflects the fact that we just have a lot more neurons so we have the ability to reflect or to represent a much richer set of relationships between things in the world.
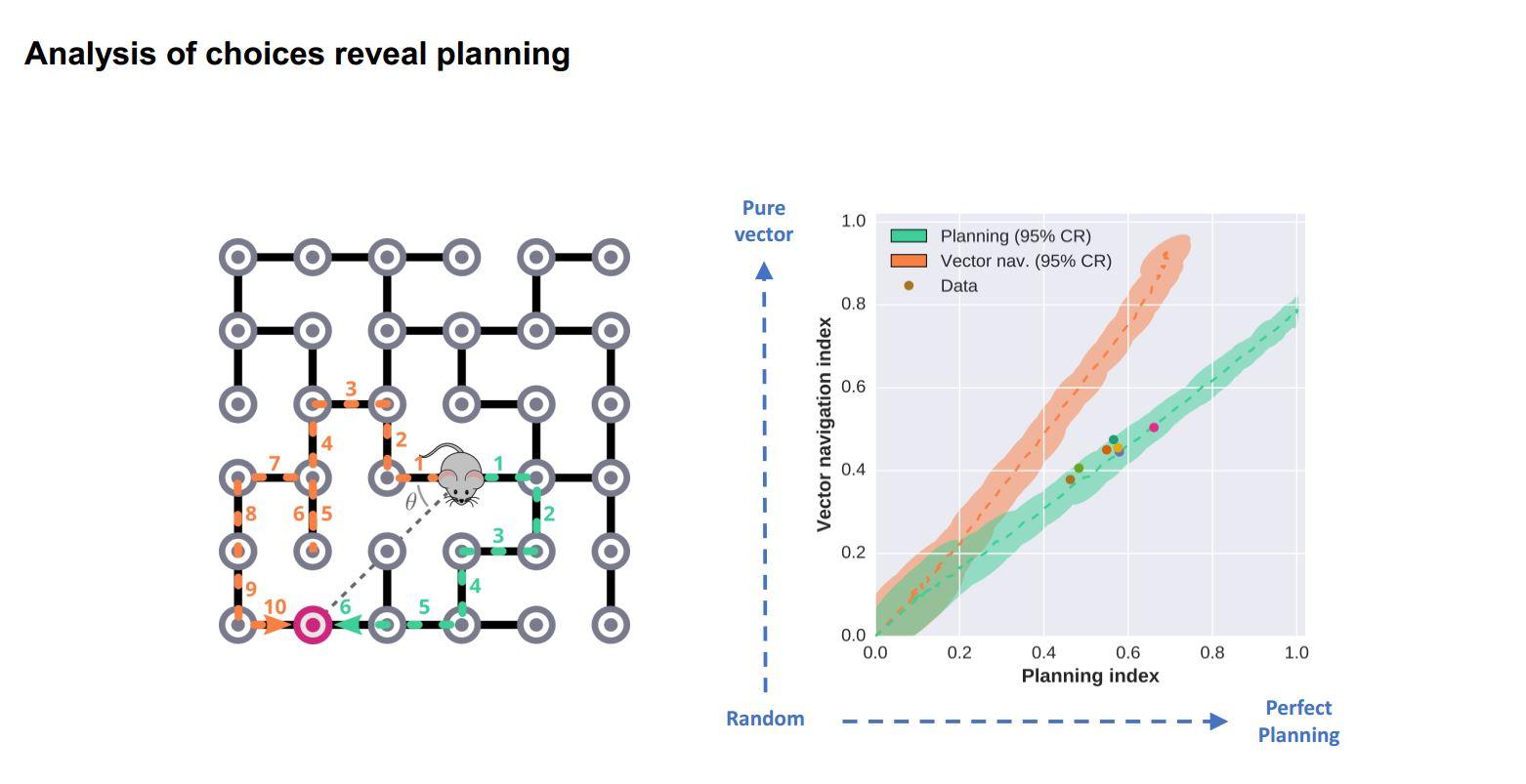
How much research has there been into whether other animals plan?
The work started back in the 30’s and 40’s with rats. There was a big debate in psychology where the behaviouralists were convinced that everything was stimulus-response habit in a similar way to Pavlov’s research; whereas people like Tolman argued that even rats learn much richer models of how the world works. While this work started with rats, I think these behaviours are certainly common to all mammals to some extent or other, although some mammals are going to have vastly richer models of the world than others. These are all questions that behavioural ecologists, and people looking at primate behaviour in the wild, are looking into.
How and why do you study generalisation in mice and what have been your main findings?
We do different behaviours all the time and generally there are components that are common across many behaviours and components that are distinct. For example, if you go from driving a car in the UK to driving a car in Europe, there are a lot of differences, such as you drive on the other side of road, you use a different hand for the gearstick and so forth. However, a huge amount of things are the same.
In order to learn efficiently in these kinds of situation, we think that you need models of the world that are modular or factorised. The idea is that you can learn about the physics of parabolic trajectories just once and then apply that to throwing a ball or to throwing a stone. You don’t have to relearn how thrown objects behave for each new object.
We don’t really have a good understanding of how the brain handles both the common elements across tasks and the differences across tasks. How do you represent the things that are shared across behaviours and then how do you combine those shared bits with the bits that are specific? For example, if someone throws you your phone versus a ball, there is a huge amount that is the same, but then the physics of the interaction with your hand is going to be different. The ball is soft and bouncy whereas your phone is hard and you shouldn’t drop it on the floor.
We try to get at these types of question in mice. We aim to use a situation where we can give mice a set of behavioural tasks that have a lot of shared structure across them but also have some aspects that are not shared. We took a probabilistic reversal learning task, which is a very classical decision task where the animal initiates a trial and chooses between two different options. Sometimes one option has a high reward probability and sometimes the other option does and this changes in blocks. To learn to solve the task, firstly you need to learn how to do trials and secondly that sometimes one option is good and sometimes the other option is good so you need to switch back and forth.
These tasks have been studied for a long time, but our twist on them is to present many different instances of these tasks in series to a mouse, using different physical configurations. For example, the place where they have to poke their nose to initiate the trial is different from task to task and the place where they have to poke their nose to choose the two different options is different from task to task. So the structure of the task is the same, but the motor and sensory correlates are different.
Mice are very good at this and the more different configurations they are exposed to, the faster they are in learning new configurations. Thus they are clearly generalising something about the structure of the task from one configuration to another.
We have recorded neurons in the hippocampus and prefrontal cortex and looked at the way the neurons are representing the task across multiple different configurations in one recording session. What we find is that some neurons really care about the differences between tasks and others seem to reflect very general aspects of the task. We can show that the prefrontal cortex cares more about the general aspects, which are true across all tasks, whereas the hippocampus remaps more between tasks.
This is a new line of research that we have been doing for about a year now and I think this new type of task is going to provide an interesting way of trying to understand the modularity of representations and how you can take knowledge learnt in one situation and apply it to an analogous situation.
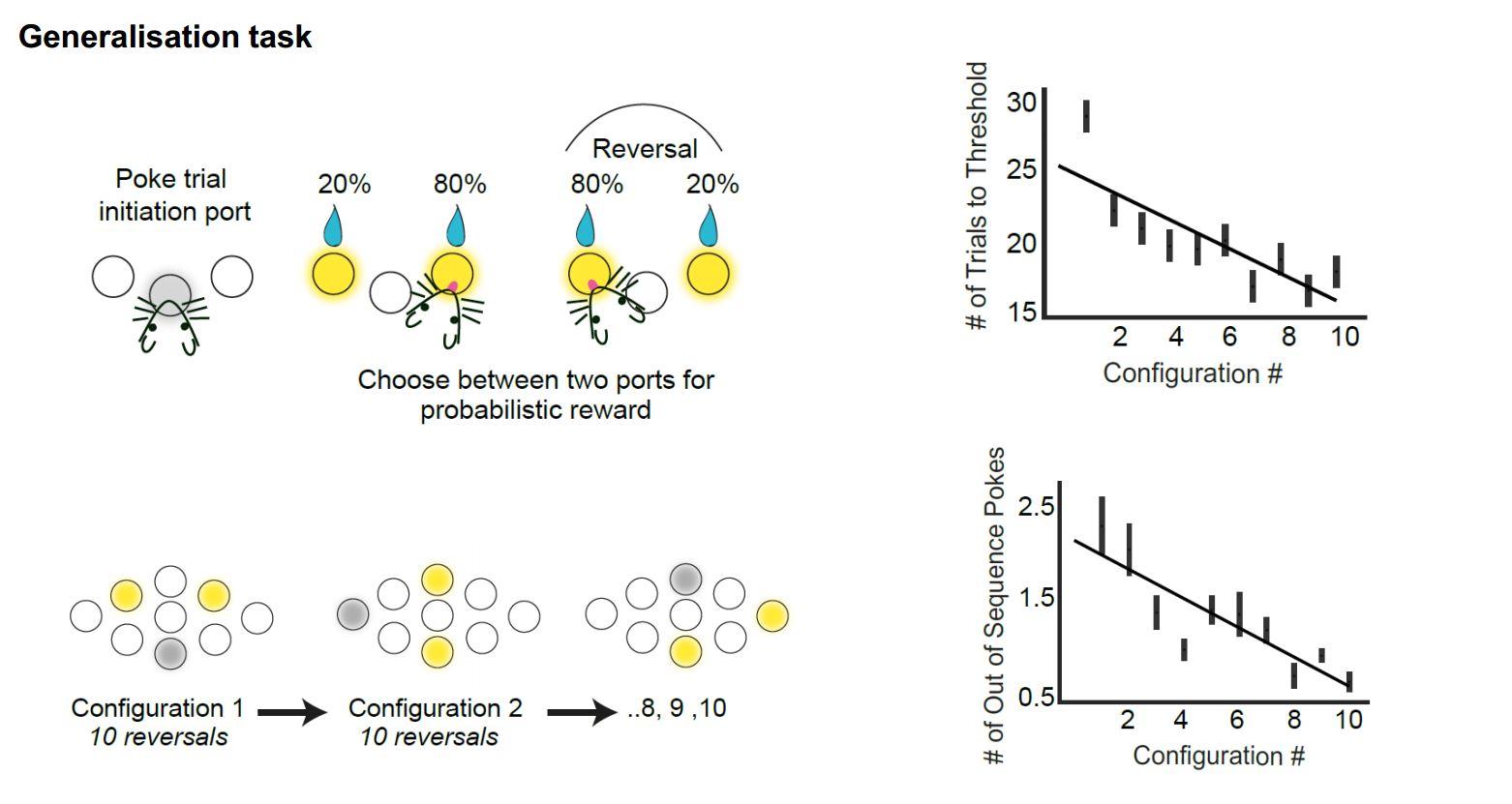
How do the prefrontal cortex and hippocampus combine these representations?
We don’t currently know but we have some ideas. One way I like to think about it is through an analogy to space. The hippocampus is famous for place cells, which fire at particular locations in space. When you move an animal to a new environment, place cells remap apparently randomly, so place cells that fire near each other in one environment won’t necessarily fire near each other in another environment. The hippocampus forms these apparently unique representations of each environment.
Whereas if you look in entorhinal cortex, you see grid representations where neurons fire at the vertices of triangular grids. But what is really interesting about the way they behave across environments is the neurons that fire together in one environment fire together in another environment. The grid may rotate or be translated, but the grid structure is preserved across environments.
One way I like to think about space is that grid cells are capturing the common features of the relationships between the place cells and extracting the invariant features of two dimensional environments. I think something analogous may be happening in these behavioural tasks, where the hippocampus is forming a unique representation for each task, but the common structure, that is present across all tasks, gets extracted by the cortex.
However, it clearly isn’t quite as clean as this neat dissociation, as we see some remapping in cortex across tasks and we see some generalisation in the hippocampus. So it is not all or nothing, but we think there are analogies in the way that task structure is represented in cortex and hippocampus and the way that spatial environments are represented. This is something we are keen to follow-up on.
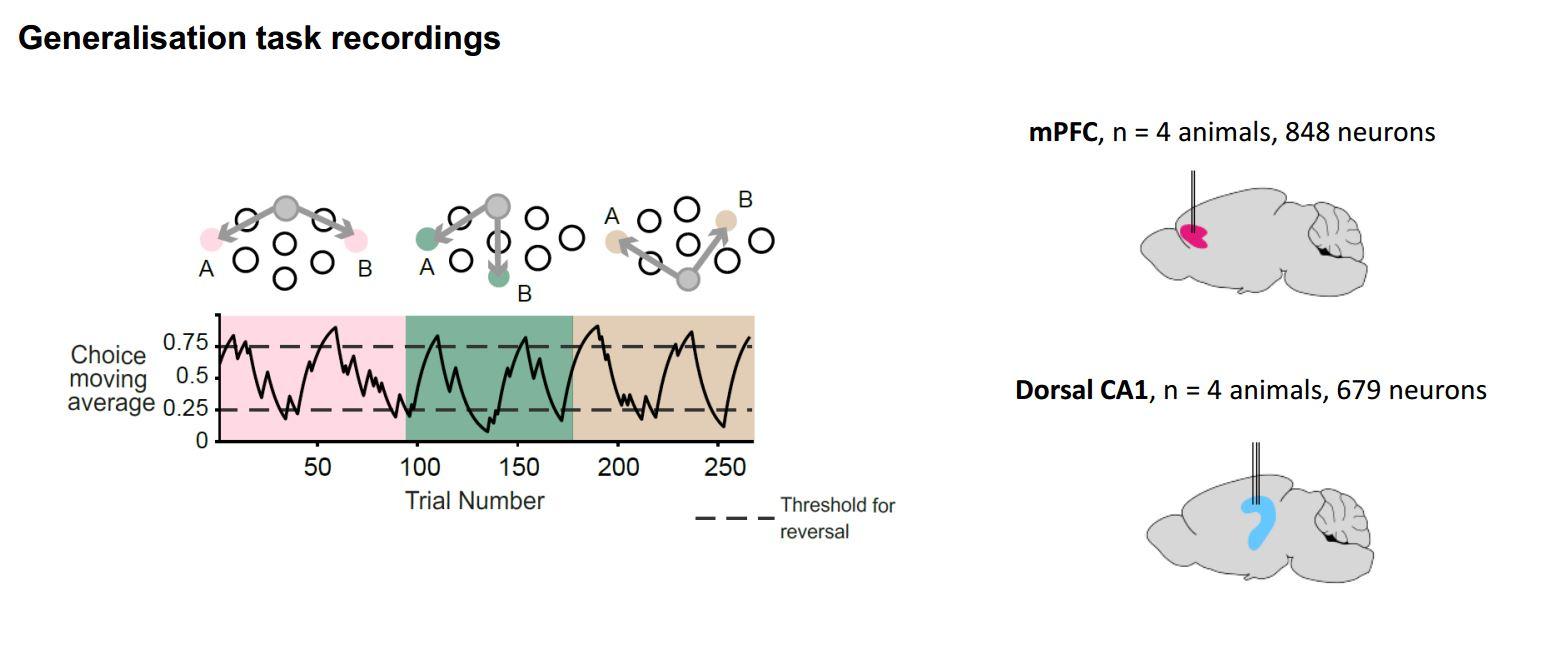
What is the next piece of the puzzle your research is going to focus on?
While we can show behaviourally that mice are planning a route to get to a goal, we would now like to know how this works mechanistically. How are the planning computations working? I think we are well placed to ask these questions, because we have this behaviour where we can get the animals to demonstrate planning again and again in one session and that's really powerful for really trying to link behaviour to brain activity.
The other question we are working on is how does the brain make good representations of the structure of tasks and the environment? For example, there is a lot of theoretical work about why grid cells are good representations of space, how they might be learnt, and how both grid and place cells might contribute to behaviour. We would like to try to take some of that theoretical understanding about how space is represented and apply that to how non-spatial behaviours are represented.
There is quite a lot of evidence that these grid representations get recruited in humans when you are thinking about conceptual decisions that can be represented spatially. For example, Tim Behrens and colleagues in Oxford did an experiment where people had to adjust the lengths of the legs and neck of a bird that is seen on a screen to morph the bird into the shape of a target bird. They found evidence that there is a grid-like representation in this abstract space of neck- and leg length. This is clearly not something the brain has developed for representing stretchy birds, it is just that the mechanisms the brain is using for representing physical 2D space seem to also be used to represent conceptual problems that can be thought of as having a two dimensional space.
About Dr Thomas Akam
Thomas studied physics at Oxford as an undergraduate then moved to UCL to join the Wellcome Neuroscience PhD program. For his PhD thesis in Dimitri Kullmann’s lab he worked on the hypothesis that oscillatory activity in brain networks plays a role in controlling information flow among brain regions. He changed research direction for postdoc, working with Rui Costa and Peter Dayan at the Champalimaud Foundation and UCL to develop methods for studying model-based decision making in rodents. He is currently a postdoc at Oxford, where he continues to work on these questions in collaboration with Mark Walton and Tim Behrens.