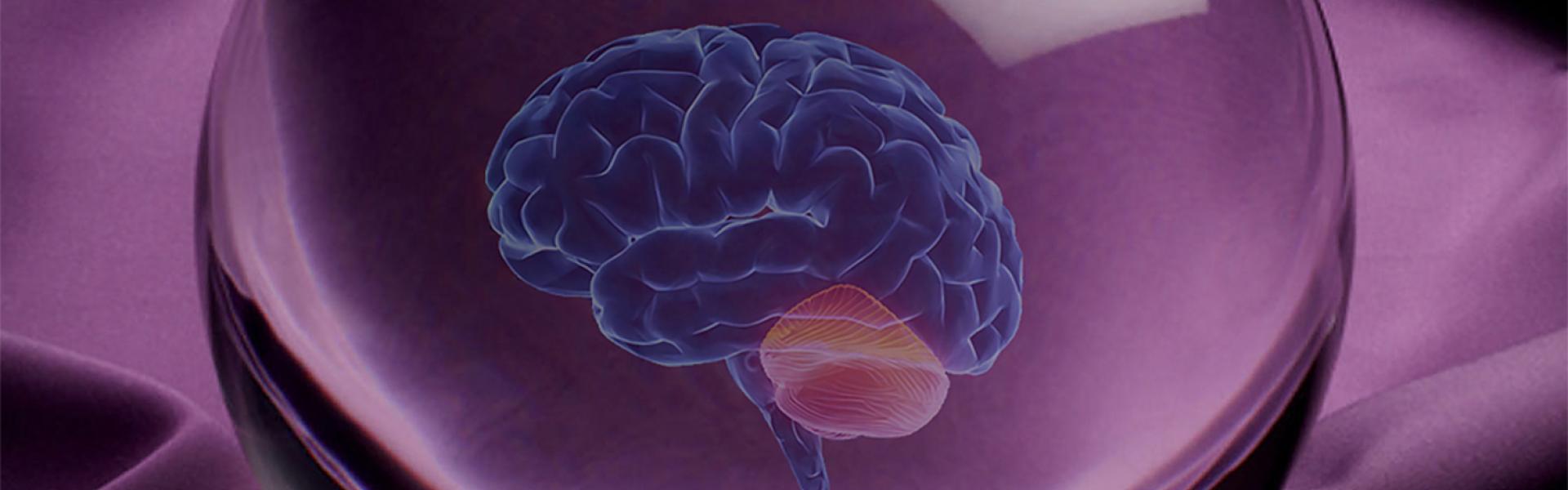
Predicting and preparing for the future
An interview with Professor Javier Medina, Baylor College of Medicine, conducted by April Cashin-Garbutt, MA (Cantab)
Many parts of the brain are involved in prediction, so what makes the cerebellum special? In the following interview, Professor Javier Medina explains how the cerebellum not only helps us predict the immediate future but also how it allows us to prepare for it.
In what ways does the cerebellum help us predict the future?
A lot of the areas in the brain help you predict what is going to happen next, but what makes the cerebellum different from other parts of the brain that are trying to accomplish the same thing, has to do with how far ahead the cerebellum is looking.
Your brain can try to predict what’s going to happen in the next few minutes, the next few hours, in the next few days, but what the cerebellum is really good at it, is telling you what’s going to be happening in the next few 300 milliseconds, so over a very short time scale. But how does the cerebellum achieve this?
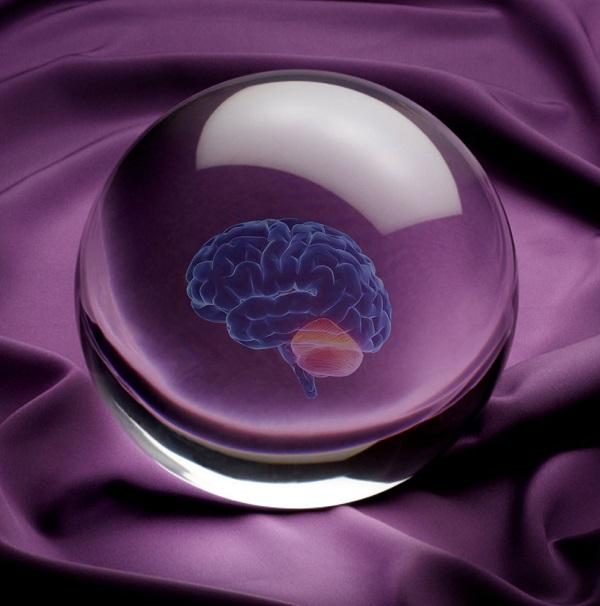
One way to predict the future, such as the weather, is to gather lots of data, for example on the atmosphere, and then feed the data into complicated physics equations, which predict how those current conditions are going to develop over the next few hours.
I don’t think that the brain has a set of equations that it can use to feed in all the data that’s coming through your senses about the current state of the world, and mechanisms relying on those equations to figure out what is going to happen next. Instead, I think the brain uses supervised learning.
To understand supervised learning, imagine you are trying to predict whether it will rain in the next 15 minutes: you make a prediction based on the evidence that you have right now, and then you receive feedback about whether your prediction was right or wrong.
In 15 minutes you will know if it rained or not and if your prediction was right, then okay, you’re good; if it was wrong, then the key to supervised learning is that there is an error signal that says, “Your prediction was wrong.” Then you can use this error signal to update your predictions, so that next time those conditions happen, you may end up making a better prediction.
How do we know that the cerebellum uses supervised learning?
We’ve known since the 1960’s that the architecture for the cerebellum, how the circuit is wired, is almost identical to an architecture that machine learning experts use to do supervised learning.
There are parts of the cerebellum that can detect error signals and then, directed by those error signals, other parts that can change the strength of connections to update predictions. This allows the circuit to make better predictions in the future by trial and error.
Why does supervised learning play a key role in current theories about cerebellar function?
The word “supervised” means you’re learning from a teacher, which is how we, as humans, often learn. Teachers can tell us whether what we are doing is right or wrong and once you have a teacher you can use supervised learning to make predictions but also do things like classification.
Classification is something a lot of people are interested in. For example, imagine you had to design a machine that can distinguish different breeds of dog from photos. The way that you would create the machine would be through supervised learning, by showing the machine millions of these pictures and acting as the teacher by feeding back whether the machine was right or wrong.
Can you please give a brief outline of your recent experiments and what they reveal about the relationship between the source of the error-related signals and what the cerebellum learns?
We use a very simple task for studying supervised learning in the cerebellum that requires mice to make predictions about the future. In the task, there is a stimulus, which is something in the world that can be used as a cue. In our experiment, the cue is a little light that comes on and then a few hundred milliseconds after the light comes on, the mouse gets a small amount of air puffed in the eye.
It doesn’t take very long before the mouse realises, “every time I see this light, I’m going to receive an air puff.” So the mouse first needs to predict that the puff is coming, and second, use that information to protect the eye, for example by starting blinking before the air puff comes.
We use this task to study the role of the cerebellum both in making the predictions about whether the puff is coming or not, and also taking preparatory actions by making these protective blinks. The mouse learns whether the puff is coming through supervised learning.
In the task, we think of errors as “sensory prediction errors.” On the first trial, the mouse doesn’t know what the light means and so the air puff hits the eye as it wasn’t predicted, thus there is an error in sensory prediction.
And it turns out, there is a little part of the brain that’s connected to the cerebellum that can detect the air puff. What makes these cells special is that, if the mouse is predicting that the puff is coming, then when you puff the eye, these cells don’t respond. So they’re not just sensory cells, they don’t just respond when you get an air puff in the eye, they only respond when you receive the air puff in the eye and you were not expecting to be puffed. Thus the cells fire whenever there is a mismatch between what happened and what was expected.
These kind of error signals are the ones that are critical for learning. So you want these cells to tell you that you were not making the right prediction, so update your prediction. But you want these cells to stop firing, once your prediction is correct, and you’re not making any errors. They are the drivers, or the teachers, of this kind of supervised learning.
Can the cerebellum learn from only errors or can it also learn from rewards?
It used to be thought that that these cells, in the inferior olive, were classed as “error cells”, meaning they compare the actual sensory input with a prediction input. In this case the comparison would be did I get puffed in the eye, and was I expecting to be puffed.
But it turns out that these inferior olive cells do more than that. They also signal in response to any stimulus that is a predictor of an “error” event, even if it is farther into the future. For example, if a cue, such as a tone, tells you the light is coming on, which in turns tells you an air puff is on the way the olive cells would develop a response to the tone, as long as the tone robustly predicted the air puff.
This is called temporal difference learning and it is something that is now used in machine learning to make learning even more efficient, to be able to do more things than just use a particular cue to predict that an event is going to happen in the future. You can now use earlier and earlier events, and find, what is the very first event here that makes the prediction.
How far back along the chain can we go?
We haven’t tested this yet, but the theory is that as soon as you’re learning this sequentially you’re always transferring. These cells will, initially, fire to the puff. After you’ve learned that the light predicts that the puff is coming, they fire to the light. If you have a tone that predicts that the light is coming, they will stop firing to the light, and they start firing to the tone.
If something, somewhere along the chain changes, how can the prediction be corrected?
We don’t know exactly how this works, but somehow these cells respond to the chain being broken as errors are being detected, so the stimulus is now unpredictable.
Describe why the changes in the activity of Purkinje cells are idiosyncratic.
Purkinje cells are the ones that receive this error related information and we think that they play a very important role in supervised learning, because they can use that error information to update predictions, and to change prediction. And the way that you change the predictions that the Purkinje cells make, is based on this error information that they’re getting.
If the inferior olive cells are saying, “You’re not making any errors,” they continue to do whatever they were doing because they are predicting things correctly. However, if they get told by inferior olive, “No, no. You are making a prediction error,” they will change. It’s controversial about what actually changes, but the idea is that there are some synapses, ultimately leading to changes in the way that these Purkinje cells fire.
What we found is that, not every Purkinje cell in this particular task changes in the same way. There are some Purkinje cells that, as the mouse is learning to make this prediction, start firing less action potentials at a precise time relative to the predicting stimulus.
What’s amazing is the precision of this timing. Over a matter of tens of milliseconds they’ll start firing less and less and less and less, up to a minimum, but then they’ll start firing again at the same level they were firing before the cue came up (i.e. the baseline). The time when they go back to firing their normal way, marks exactly the time when the puff is about to come!
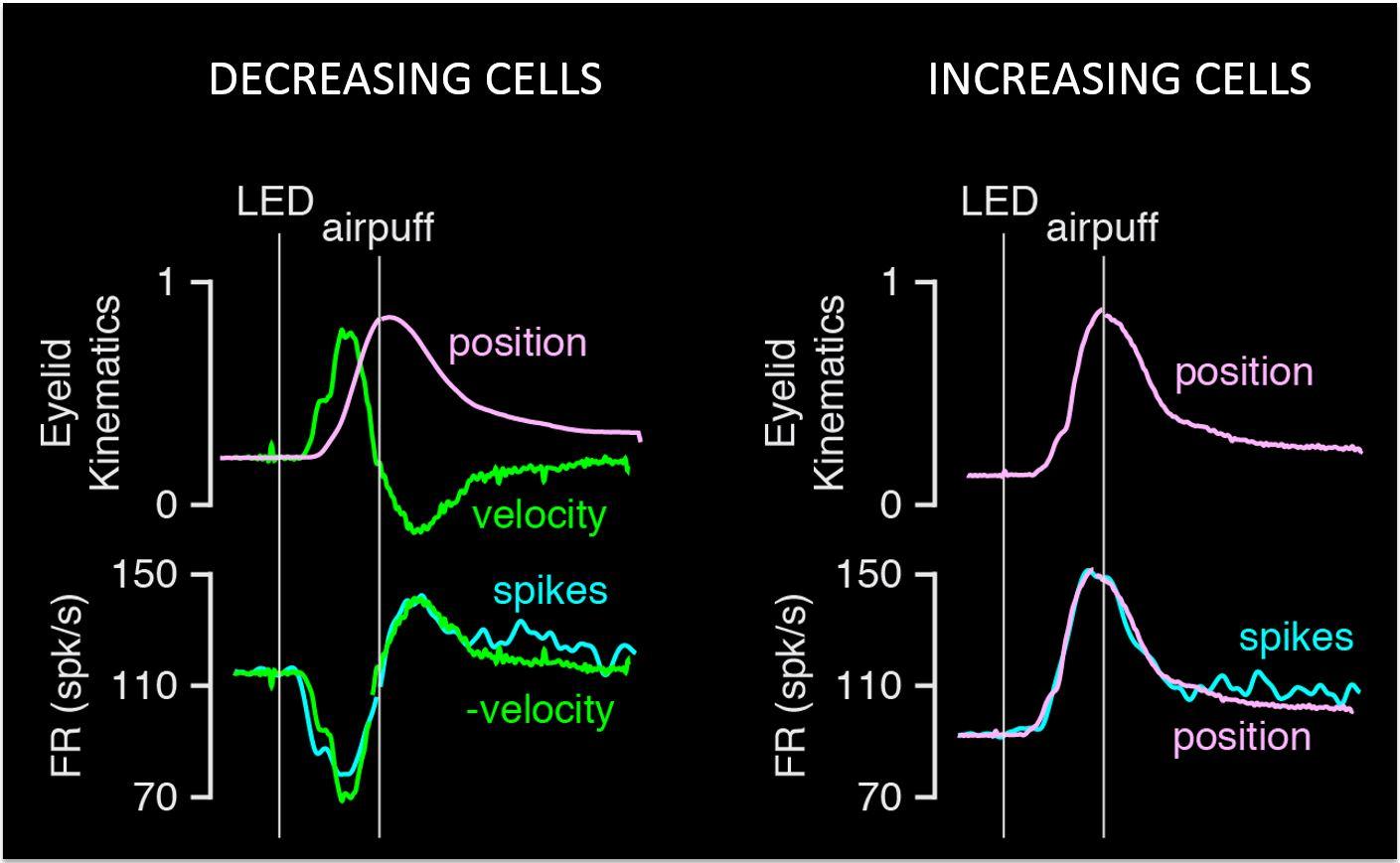
There is also another kind of Purkinje cell that does something very different in that they start firing more and more as you get closer to the air puff. So they’re making a prediction also about exactly when the puff is going to come, but they’re representing that information by increasing the firing rate, as opposed to this ones that fire less and less.
We have yet to discover, why do you need both kinds of Purkinje cells? Or is there more information in one versus the other? What is that modulation firing rate being used for?
One of the interesting things is that, for both kinds we know that you can look at the firing rate of the Purkinje cell and predict exactly when the puff is going to come. But you can also take the eye blink movement, and the speed at which you’re closing that eye, is perfectly correlated with these changes in firing in the Purkinje cell. So it seems that not only is the Purkinje cell providing information to the rest of the brain about when the air puff is coming but also how fast the movement needs to be to avoid the puff.
Do these changes also enable the cerebellum to prepare for the future by taking anticipatory action?
A lot of people that study prediction feel like, if we can find how the brain, or the cerebellum, or cells make predictions, that’s good enough. But I disagree as although it is interesting to know how predictions work, you only want to know that information so that you can take anticipatory action that’s going to help you. Knowing that the air puff is coming is no good unless you can use that information for something.
The pathway through the deep nuclei is very well known and if you inactivate any part of the output pathway, you may still see Purkinje cells making this beautiful prediction, but if you inactivate the cells that the Purkinje cells are contacting, the mouse will not be able to make these protective blinks anymore.
The cerebellum is heavily connected to motor areas in the rest of the brain, sometimes directly to the spinal cord and brain stem, which are involved in controlling the muscles directly, but also sending information back up to other areas of your cerebral cortex, or neocortex, that have to do with motor control.
What is the next piece of the puzzle your research is going to focus on?
A postdoc in my lab recently found that the mice are not just blinking in preparation for the air puff but they are learning to do all sorts of protective movements. The mouse tries to push the whole body away from the air puff, so it is a full synergy coordination of different muscles across the body.
We now know that there is a little part of the cerebellum that is controlling this whole synergy, and it’s a very small area of the cerebellum. But we have no idea how it’s possible for this little part of the cerebellum, which we thought was very much involved in controlling blink movements, to control the whole synergy.
We know it could be through direct connections to motor neurons, or it could be that a lot of the information is being sent to motor areas in the neocortex, and the neocortex is helping organize, and coordinate, this whole synergistic movement, so that is the next piece of the puzzle we are trying to solve.
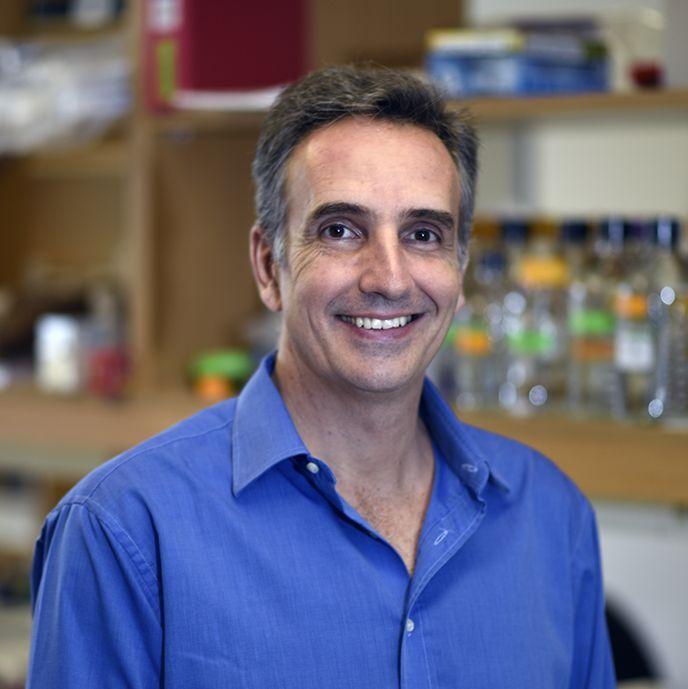
About Professor Javier Medina
Dr. Medina was born in Madrid, but grew up in sunny Malaga on the Mediterranean coast of Spain. After high school, he was awarded a full-tuition scholarship to study in the United States. He graduated summa cum laude from Drexel University (Philadelphia) in 1993, with dual degrees in Physics and in Computer Science. He then moved to The University of Texas (Houston), where he received a PhD in Neuroscience in 2000.
His thesis work and subsequent post-doctoral studies at The University of California (San Francisco) have a common goal that continues to drive much of his research program today: to achieve a full mechanistic understanding of how the brain learns to control our movements with precision.
In 2008 Dr. Medina became an Assistant Professor in the Psychology Department at The University of Pennsylvania (Philadelphia) and in 2014, he was promoted to the rank of Associate Professor. In 2015, Dr. Medina moved his laboratory to Baylor College of Medicine (Houston), where he is currently Associate Professor and the recipient of the Vivian L Smith endowed chair in Neuroscience.
His laboratory is currently using computational approaches and tools from pharmacology, in vivo neurophysiology and optogenetics to examine the mechanisms of cerebellar learning in mice.