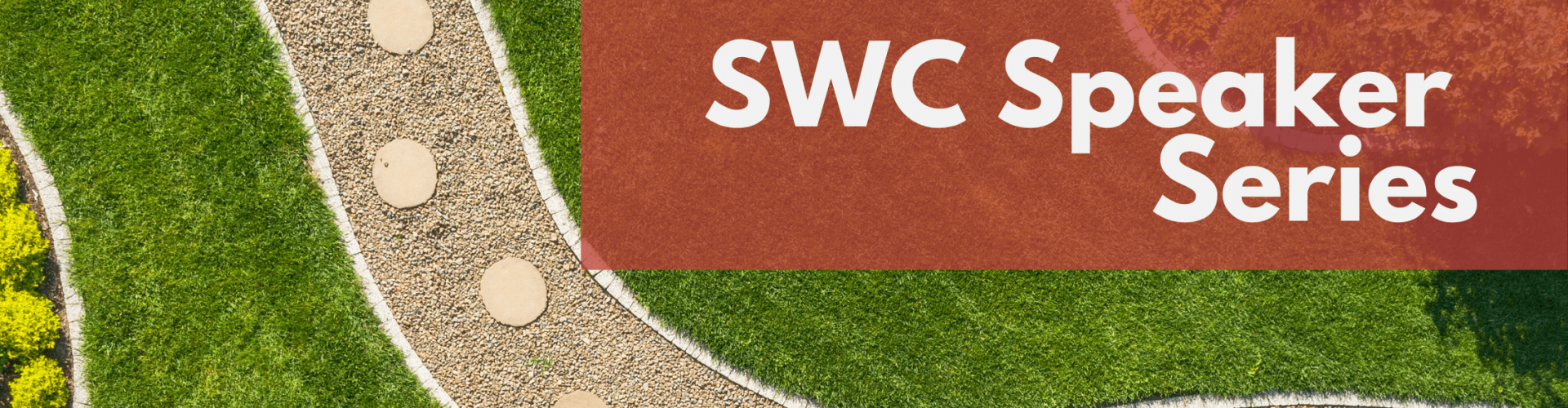
Treating space as a sequence
An interview with Dr Dileep George, DeepMind and Vicarious AI conducted by April Cashin-Garbutt
In response to environmental changes, hippocampal place fields show a variety of remapping phenomena. Dr Dileep George recently gave a seminar at SWC where he described how treating space as a sequence can resolve many of the phenomena that are ascribed to spatial remapping. In this Q&A, he shares more about the Clone-Structured Cognitive Graphs model and the implications for neuroscience and artificial intelligence.
What are hippocampal place fields and how do they remap in response to environmental changes? Why are these remapping phenomena characterised in spatial terms?
If you take neurons in the hippocampus and plot their activity in correspondence to the location of the animal in the arena, you’ll see that specific neurons fire at specific locations. This overlay of neuron firing on top of the spatial map of the arena is the place field of that neuron. This was a landmark discovery because it gave evidence to the idea that the hippocampus is encoding locations to form a map of the environment.
The idea that hippocampal neurons fire to represent specific locations — places — was revolutionary and became a paradigm of its own. However, over time, anomalies to the location encoding story started popping up. For example, if the rat is first trained in a particular room, and then some aspect — geometry, colours, placement of barriers — etc. of the environment is changed, the place cell responses changed in a systematic manner. Collectively, these phenomena are called ‘remapping’ of the place fields.
Researchers started probing these anomalies as variations from the place field story — even the term “remapping” implies a location encoding framework. People started asking questions about why these remapping happen? One particular phenomenon — the remapping that occurred when the testing arena was an elongated version of the training arena — could be characterized using the distance from the boundary of the arena. This then suggested that place cells are not just encoding location, but also distance to the boundaries. Another set of researchers observed that place cell responses remap in relation to movements of objects in the arena, not just the boundaries. Then maybe place cells are encoding distances to objects as well as boundaries?
So basically, the paradigm of place cells as location encoding influenced how we looked at every new phenomena. We tried to interpret those as variations of the location-encoding theory of the hippocampus.
Can you please outline your Clone-Structured Cognitive Graphs (CSCG) model and explain how it shows that allocentric “spatial” representations naturally arise?
Animals like rats and humans do not sense location directly. We don’t have a GPS wired into our brain. Any location encoding that is happening has to be learned from sensory inputs like vision, touch or smell. These senses are experienced sequentially as the animal makes actions in the world. Therefore any theory of place cells has to explain how location encoding can develop from sequential inputs.
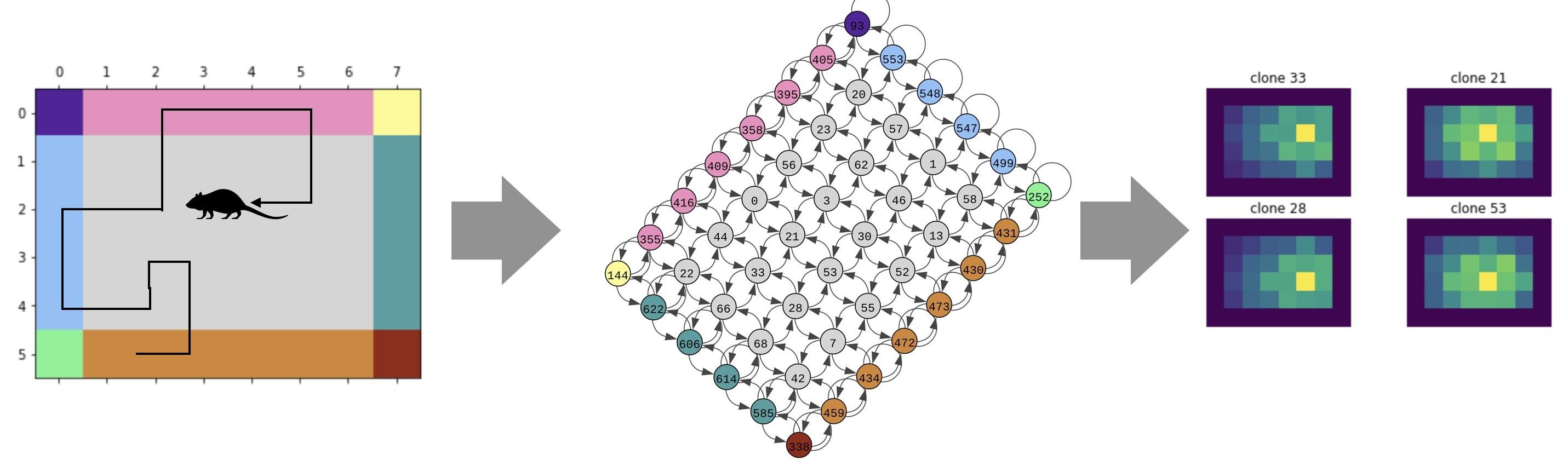
CSCG formulates the problem that the hippocampus solves as the problem of learning a hidden graph from a sequence of observations. The observations we receive as we walk in the world can be thought of emissions from the nodes of a hidden graph. What makes this a hard learning problem is that the observations do not directly identify the nodes in the graph due to sensory aliasing — different nodes in the graph can produce the same sensation.
CSCG tackles this by introducing a structured graph with “clones”. Clones are a set of nodes in the hidden graph that will emit the same observation. These clones are hard-wired to have the same observations, but they can differentiate themselves using sequential context. Consider the word “bank”. It can mean a river bank or a money bank. In CSCG, a set of hidden nodes will be allocated to represent the word “bank”, and initially they will be randomly wired in their contexts. With learning, the different clones will come to represent the different temporal contexts in which the word “bank” is used.
How can an organism utilise CSCG for navigation without having to compute place fields or think about locations?
It’s that same idea of sequential context what is used to represent locations. A location is nothing but a set of sensations that appear in a particular context. Learning in CSCG successfully groups or splits sensations according their contexts to reveal their correspondence to locations without having to make Euclidean assumptions. If the same sensation occurs at multiple locations or heading directions, they just get represented by different clones in the model.
Let’s take a step back and ask the question -- how do we as humans represent location. What does location mean to us? We experience location as a sequence of sensations — visual, tactile, sounds, or odour — that we experience when we are at that place, in response to actions we take. We don’t experience a location as a (x,y,z) coordinate.
It turns out that there is no need to decode locations either. The memory of a location is just a clone or a set of clones that were active when you were there, and those correspond to the sensory observations that occurred in that context. Index and remember those clones, and you can plan a path to those set of clones from elsewhere in the environment — the elsewhere is also represented as the set of clone activations. So, there is no intermediary of location variable that needs to be decoded.
More importantly, this is also robust to errors. Even if the centre of a uniform room is not represented with the same resolution as the periphery of the room, it doesn’t affect navigating from one end of the room to another.
How does CSCG explain spatial and temporal phenomena like boundary/landmark/object vector coding and event-specific representations?
As I mentioned earlier, the right way to interpret locations would be as the set of sensations that occur in a particular sequential context. This is what CSCG does — the different clones in CSCG can represent different locations or heading directions, without having to think of x-y-z coordinates explicitly. The different clones in CSCG have a direct correspondence to place cells because they do respond at that location. But the mechanism by which they respond at that location is by representing the sensory sequences that pass through that location in a latent space.
The correspondence between hippocampal responses and locations was one of the early successes, and that might have anchored us into thinking of hippocampal responses in terms of space. When we map the sequential responses on to a static place field map, we lose a lot of information about the dynamic nature of those responses, and they create baffling phenomena in spatial terms.
One example of this is remapping of place fields in response to the elongation of an arena. The observed phenomena is that the place field splits into two and becomes bi-modal. If we try to characterize these in spatial terms, then we’ll notice that the split occurs in vector distance from the boundaries. But that misses the dynamics — half of this field will occur when the rat travels in one direction, and the other half when the rat travels in the other direction. If you think of place cells as encoding the sequential context, then this comes out naturally without having to think about boundaries or vectors. When the arena is elongated, the partial sequential contexts now occur in two different locations, and these contexts occur only during the specific travel directions. Moreover, it will also explain why place field remap when we move landmarks, or texture boundaries. In all cases, what we are changing is the sequential context that rat will experience.
Trying to characterize or model these in terms of space and location will require hypothesizing a new mechanism for each of these phenomena. Moreover, characterizing in terms of space might amount to characterizing the effects, not the cause, and could be a constant source of anomalies. The latent sequential model can explain multiple of these phenomena using just one mechanism — sequential context.
What other explanations can CSCGs offer?
The learned latent graphs in the CSCG is connected to the observations through a mapping matrix called the emission matrix. The latent graph can be thought of as a schema and the emission matrix is the binding of that schema to observations.
Interestingly, the clone structure also implies a slot structure on this latent graph. For example, it could encode the knowledge that the middle of the room is a uniform colour, irrespective of the particular colour. So all the nodes corresponding to the middle of the room are a slot for a single observation to bind to.
We think this is how knowledge about one situation can be transferred to other situations. When we go to a new place, we bring the abstracted knowledge from prior experience. Using the latent transition graphs and slots as schemas is a good way to do this. This is a good area for future exploration, and we’re hopeful that someone will build on this.
What impact do you think overall latent sequence learning using graphical representations might have for understanding hippocampal function? What implications could this have for artificial intelligence?
I do think we have brought together different pieces of the hippocampus puzzle into a very simple computational model that is not only easy to understand, but also easy to build and use. I think that simplicity is powerful, and I think this model will serve an important role in understanding hippocampal function going forward. I’d go as far as to say that a model like this was anticipated by Buzsaki, Eichenbaum, Behrens, Ranganath, and many others who have worked on the hippocampus. Behren’s lab also has a model called Tolman-Eichenbaum machine that tackles part of the puzzle.
Some of the capabilities of the model tackle exactly the capabilities that are lacking in current AI systems — model-based learning, rapid transfer of knowledge from one situation to another, planning, etc. So I think the model has significant implications for AI.
What’s the next piece of the puzzle you plan to focus on?
We’re working on bringing together the different pieces we have worked on — generative perception, dynamics, concept learning, and cognitive maps — into a coherent cognitive architecture that can tackle some important challenges in building general intelligence.
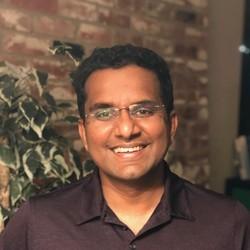
About Dileep George
Dr. Dileep George is an entrepreneur, scientist and engineer working on AI, robotics, and neuroscience. He co-founded two companies in AI — Numenta, and Vicarious. At Numenta he co-developed the theory of Hierarchical Temporal Memory with Jeff Hawkins. Vicarious, his second company, was a pioneer in AI for robotics, and developed neuroscience-inspired models for vision, mapping, and concept learning. Vicarious was recently acquired by Alphabet to accelerate their efforts in robotics and AGI. As part of the acquisition, Dr. George and a team of researchers joined DeepMind where he is currently at. Dr. George has an MS and Ph.D in Electrical Engineering from Stanford University, and a B.Tech in Electrical Engineering from IIT Bombay. Twitter: @dileeplearning Website: www.dileeplearning.com