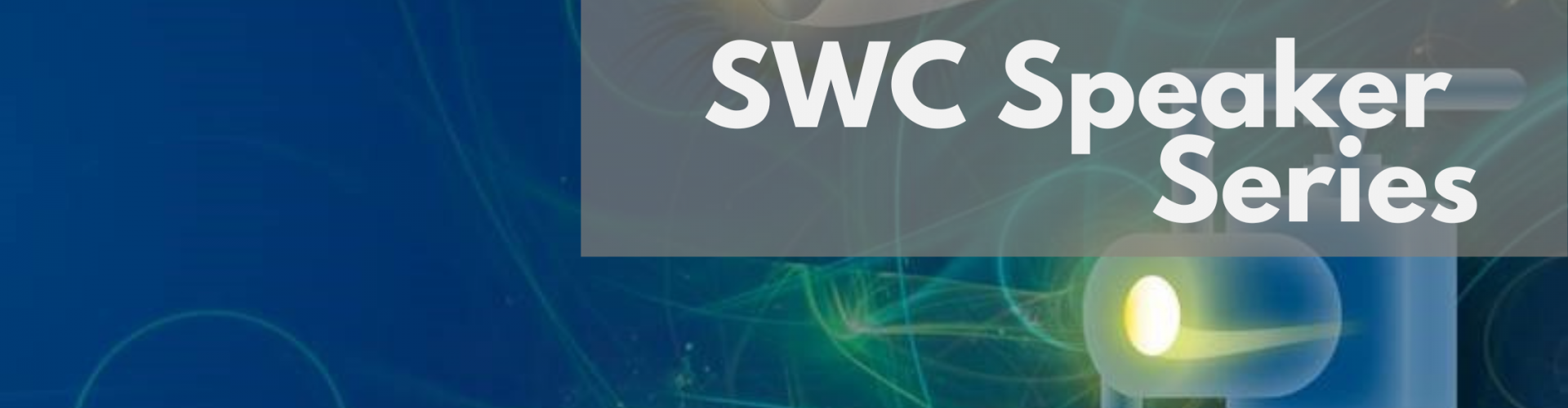
Converging toward closed-loop perception
An interview with Professor Ehud Ahissar, Weizmann Institute of Science, conducted by Hyewon Kim
We constantly perceive the world around us through our sensory organs when we’re awake, be it through our eyes, nose, or fingers. But how does perception actually arise in the brain? In a recent SWC Seminar, Professor Ehud Ahissar discussed accumulating evidence in support for perception being a closed-loop, brain-world convergence process. In this Q&A, he untangles what such terms might mean and the implications of his key findings.
What first sparked your interest in active perception?
This is a dynamic story in itself! I was interested in understanding perception and plasticity in the brain and started from the classic textbook approach – with passive perception. I studied passive perception step-by-step, recording neurons in the cortex but not understanding how it could work.
Then I found myself going down to the thalamus and to the brainstem – still not understanding how it works – and it was only when I got to the sensory organ in the vibrissal system at the receptor level that I understood that perception is not passive, but active.
This was a lightbulb moment. Perception must be active, otherwise it cannot work! Then, I started to go back to the higher processing levels with this new lens of active sensing.
How much is known about perception being a closed-loop, brain-world convergence process?
Perception being a closed-loop, brain-world convergence process was not known. Basically, it was our suggestion for the last 10-15 years. To this day, some people accept it, but most are suspicious. But the exact phrasing of perception being a convergence process within specific closed-loops aside, you can trace back the critical dependence of perception on closed-loop processes to more than 100 years ago. People like Dewey, von Uexküll, Powers and others in the last century explicitly talked about the closed-loop idea in one way or another.
Also, if you follow the earlier writings of the Greeks, you find that they usually belong to one of two broad views of describing the world, static or dynamic. The dynamic view is akin to the closed-loop thinking.
So, the academic world has been divided and there is a long history behind these two streams of thought.
Would you say it’s still divided?
Yes. Today in neuroscience, most visual neuroscientists subscribe to the static and open-loop view of perception, and most tactile and somatosensory researchers subscribe to the closed-loop view, and maybe half-and-half are divided between the dynamic and static views.
What have been your key findings so far?
The key findings comprise a circle between theory and experiment. I must admit that the theory leads the experiment. We still have much more understanding (in some cases) or speculations (in other cases) than we have evidence for what we are proposing, so thinking goes first.
The key finding that lead to the active sensing idea in vibrissal touch has been that the receptors actually do not function properly if they are not in the active mode. They must be actively moving in order to function properly. Once we have realized that, the next key finding was the coding scheme of active touch – a triple code for the three polar dimensions.
The key finding for the closed-loop idea has been a combination of thinking theoretically and looking at the anatomy of the brain, realising that there are literal loops! We figured there must be a convergence process and then we looked for the evidence for such a process. We have now sufficient evidence for the perceptual convergence behaviour, and we show that it typically takes no more than 3-4 motor-sensory cycles to converge to a perceptual attractor in typical conditions.
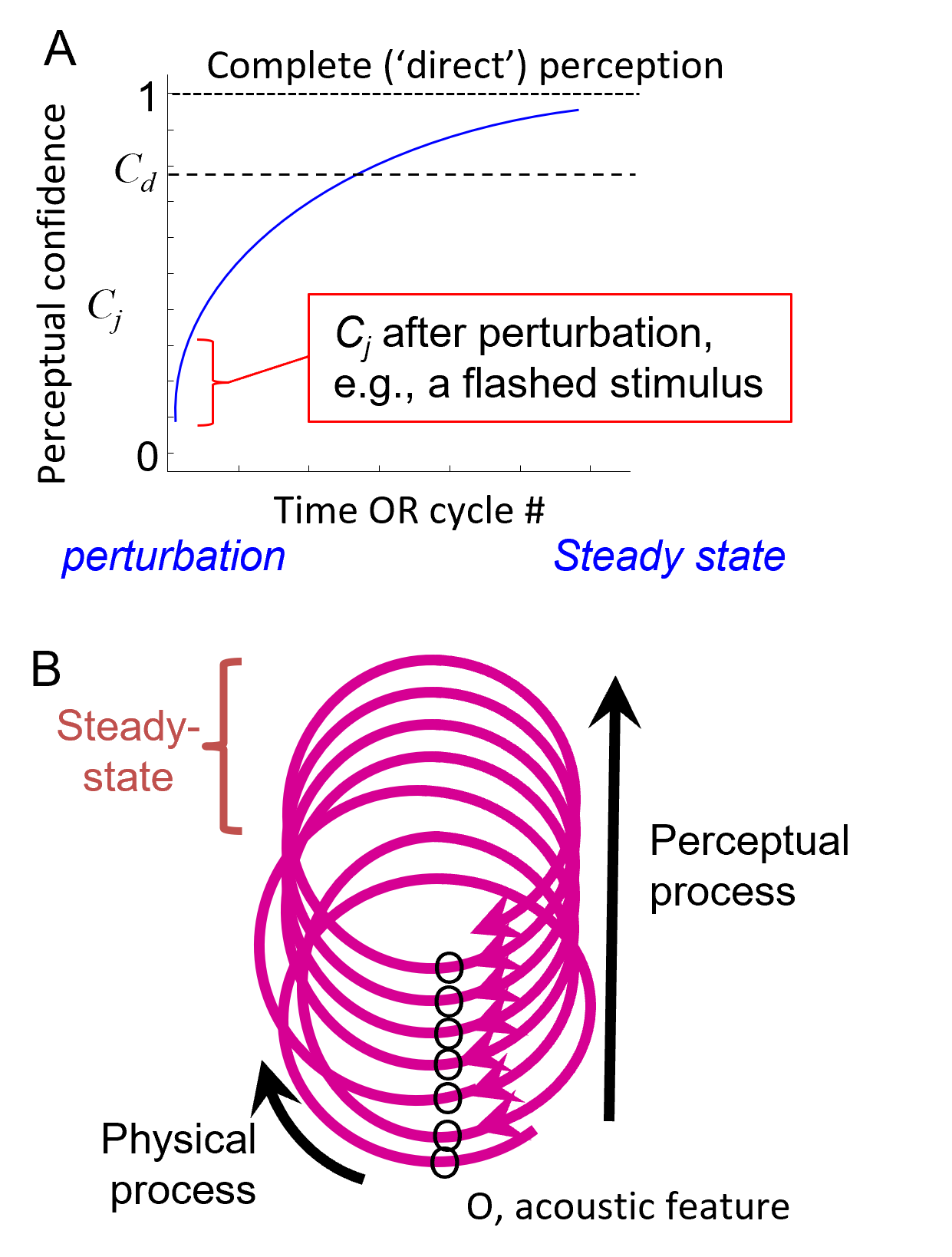
CLP convergent dynamics. (A) The loop starts converging towards its steady-state (in which state perception is complete) upon the first interaction with the acoustic feature. The confidence of perceiving feature j (Cj) gradually increases during convergence. The loop may quit the process when Cj becomes larger than a certain internal threshold (Cd) or upon an internal or external perturbation. (B) A spiral metaphor of the convergence process, showing the iterative sampling of the acoustic feature. Events along the loop in each iteration obey physical rules while events across iterations form the perceptual process leading towards a steady-state.
How would you describe convergence behaviour to someone who is not familiar with the term?
It could be confused with spatial convergence. Usually in anatomy, two inputs can converge on the same output, for example. Most people will go to this attractor of understanding.
The kind of convergence behaviour I’m talking about is temporal convergence and it’s a convergence of a process towards a steady state. It’s a dynamical convergence and not a spatial one.
What experimental techniques did you use to find this out?
We started looking at neuronal convergence, or signatures of neurons in the brain for convergence. This turned out to be very difficult. We figured that with neural recordings, you always have the sampling problem. Even if you have a thousand cells, it’s a very small sample relatively speaking. And you are never sure you got all the relevant neurons at any moment and you are not even sure that the same neurons serve the same process in different trials – the inter-trial variability is huge.
We came to understand that we should better find a bottleneck, where all the information about the loop must be present in each trial. Assuming that the entire loop converges together, its bottleneck are the interactions between the sensory organ and the external object. For the vibrissal system, measuring the bottleneck’s dynamics (and hence loop dynamics) means taking high-speed videos of the whiskers together with the object and tracking both the motor variable, which is the angle of movement, and the sensory variable, which is the curvature of the whisker (the curvature gives you the mechanical moments).
In this system, we are lucky – this is a very friendly system to the researcher because in the same video you can get both the motor and sensory variables. In vision, it’s more difficult because we have to use simulations of the retinal dynamics in order to know what the response in the retina is – it’s not so straightforward. But we do both. We track the whiskers, track the eyes, and now we have come to study the animal behaviour in a virtual reality environment where the head is free to move.
Head-free setups are a huge factor both in animals and humans. If you fix the head, the world is different. For example, we thought we discovered a phenomenon related to smooth pursuit for the first time, but then realised people reported it 50 years ago. If I ask you which organ is doing most of the job in smooth pursuit, what would you say? The eye or the head?
The eye?
Ok, that’s indeed what we said. We then looked at the data in head-free setups and realised that actually the head is doing all the job! The eyes only make small corrections. And it turns out people reported this, but forgot about it. In head-fixed experiments, you have no option but to look around by moving your eyes, but in the freely moving set-up, you move your head all the time. In rats, it’s the same story. Both the mechanical and neuronal physiology is totally different between the head-fixed and head-free agent. So now, we only use head-free experiments.
To enable head-free environments, there are more technological challenges involved. And in terms of modelling, you have more degrees of freedom, which makes it difficult. But there is no other choice. You always have to reduce the system in one way or another because you cannot track everything. But you should recognise what things should not be reduced out. One is motion because we are interested in active sensing. You cannot immobilise the system in order to understand active sensing. You could limit the stimuli, context, and other conditions, but not the motion itself. Motion should be factored in if you want to understand natural perception.
What are the main implications of your research?
For science, it’s simply understanding how we perceive. For robotics, there’s a huge potential implication because it means that if the field wants to deal with real-world scenarios, we should move on from the open-loop, deep network-like technology which is only passive and feedforward. Deep networks are great at classifying fixed computer images, but they are not so good at processing the real world in real time. The potential lesson from our findings is that a system has to move to closed-loop perception if it wants to excel in this kind of real world classification.
To help people with visual and hearing impairments by means of sensory substitution or sensory addition, you have to understand how the sensory system works. For example, one lesson is that you need to be active in order to perceive. You cannot passively put something on the tongue or the back and expect a blind person to infer from this the real world. You should let it activate the sensor – for example, put the camera on the finger and let it scan the world if you want to use sensory substitution. By doing this, you are operating on existing sensory motor loops. If you put the camera on the head and activate it on the tongue, you don’t have an existing loop for this split scenario. This would be very hard for blind people because they then have to move through the cognitive level of interpreting the relationships between their motion and sensation instead of relying on low-level existing loops and their existing attractors.
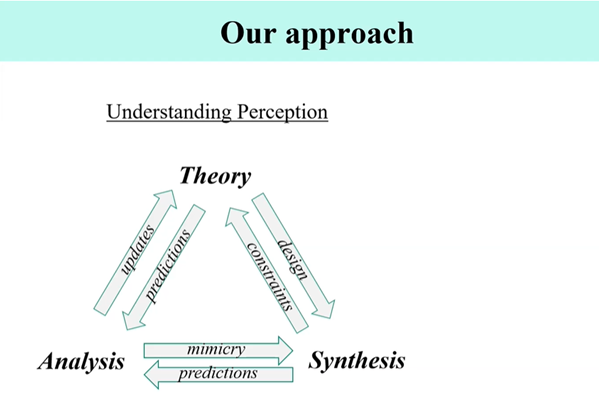
What’s the next piece of the puzzle you’re excited to focus on?
There is this triangle that I presented in my talk which describes our approach to understanding perception through theory, analysis, and synthesis. We want these to converge – get to an agreement between all these parts. In vision, for example, we started with understanding a single perceptual loop, which is the drift loop. We are now starting to expand and follow the steps of evolution, grow to evolutionary-newer loops. The next piece of the visual puzzle is the saccade loop that we need to integrate together with the drift loop, and the next loop we need to integrate will be the head motion loop. These are several pieces of the puzzle, helping us “building up” the visual brain from inside out.
For vibrissal touch we have started by building up the whisking loop – the loop controlling self-motion of the whiskers. This loop is usually at steady-state. The next loop in the speculated evolutionary order, and thus also in the inside-out loop architecture, is object localization and the further newer one is the loop controlling object identification. All object-related loops are dynamically roving between perturbation to steady-states, attempting to converge at familiar perceptual attractors or learning new ones.
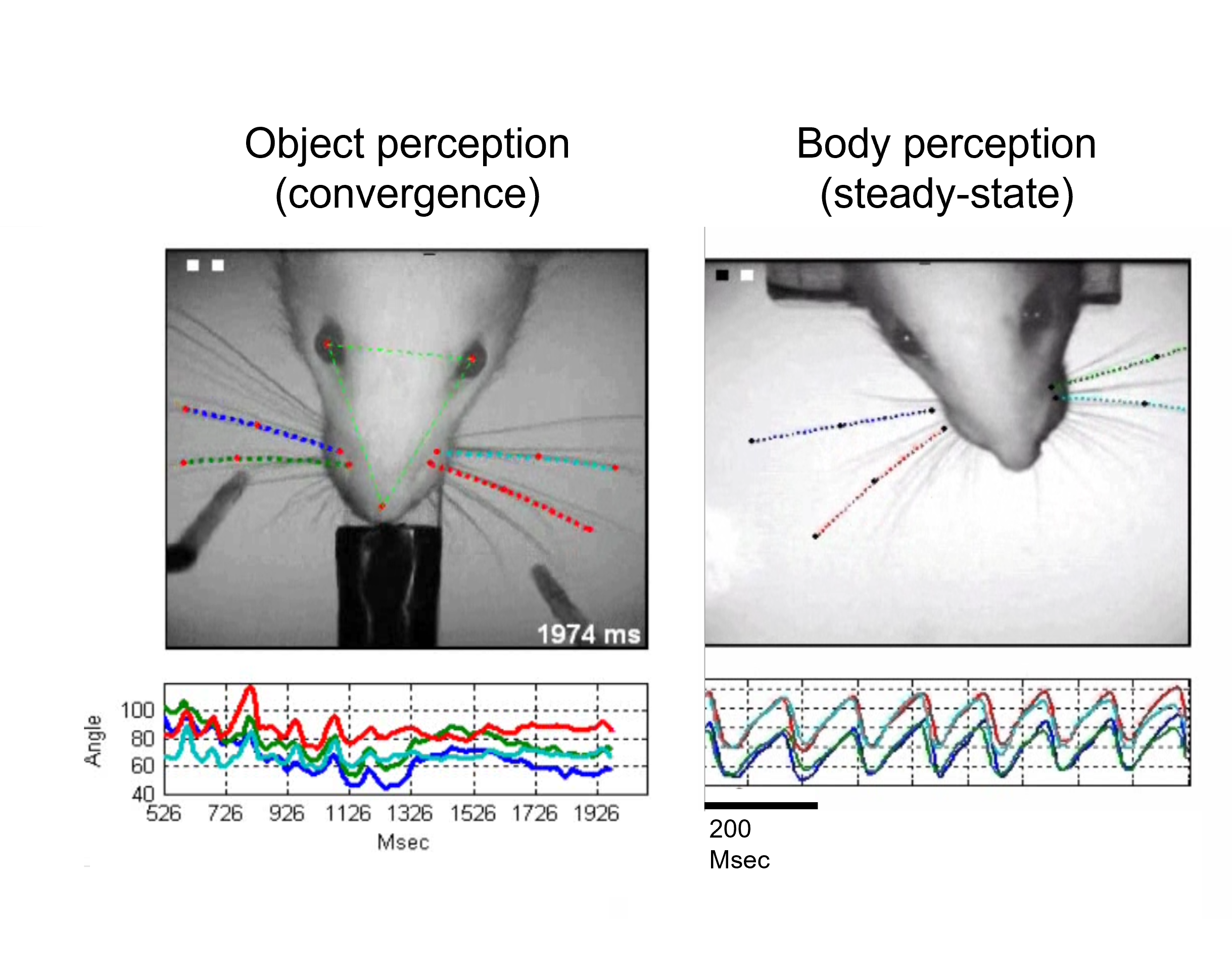
About Ehud Ahissar
Ehud Ahissar is a professor of Brain Sciences at the Weizmann Institute since 1991, studying embodied perception of touch, vision and speech in rodents, humans and artificial devices. He earned a BSc in Electrical Engineering from Tel Aviv University in 1978, worked at ELTA Group and Elbit Systems Ltd., and then went on to earn his PhD in Neurobiology in Moshe Abeles’ lab at the Hebrew University of Jerusalem in 1991.