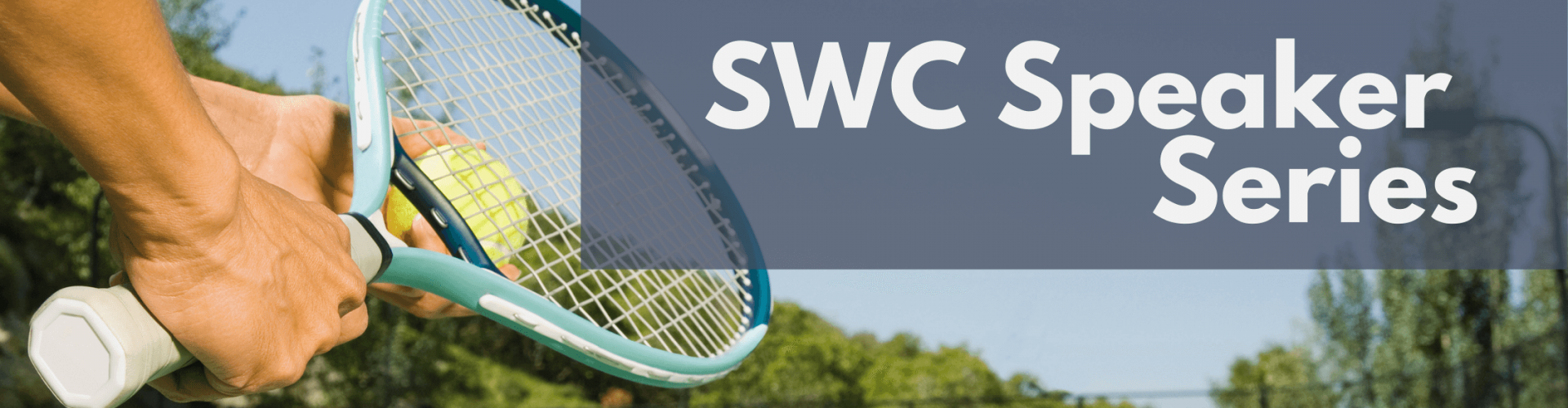
Planning, knowledge, and motor skill
An interview with Dr John Krakauer, Professor of Neurology, Neuroscience, and Physical Medicine and Rehabilitation at the Johns Hopkins University School of Medicine, conducted by Hyewon Kim
When we type on a keyboard, our minds may not be able to grasp the location of each key, but our fingers can. What does this phenomenon say about how we learn motor skills and is this reflected in higher order cognitive processes of the brain? In a recent SWC Virtual Seminar, Dr John Krakauer shared his work exploring intelligent reflexes, as well as first- and second-level explainers for the cognitive-motor interface. In this Q&A he highlights how motor planning has been historically defined and how such definitions are undergoing a makeover.
What is currently known about how our brain plans for motor actions?
‘Planning’ is a word in neuroscience that has been used in many different ways. You can plan a vacation and plan a reaching movement, but the word probably has different connotations in these two cases. What we have argued against is that you actually have to plan the detailed spatiotemporal dynamics of execution in the reaction time before movement initiation.
A better use of the word ‘planning’ is at the goal level. For example, do you want to reach for a cup, or up to a shelf? It’s really at the level of the goal that you plan between the alternatives. Based on our work, we think that this is a more useful cognitive notion of planning. You plan at the goal level and execute at the action level. We’ve moved away from the idea that you actually plan the parameters of the movement itself – the velocity, the acceleration, the direction, etc.
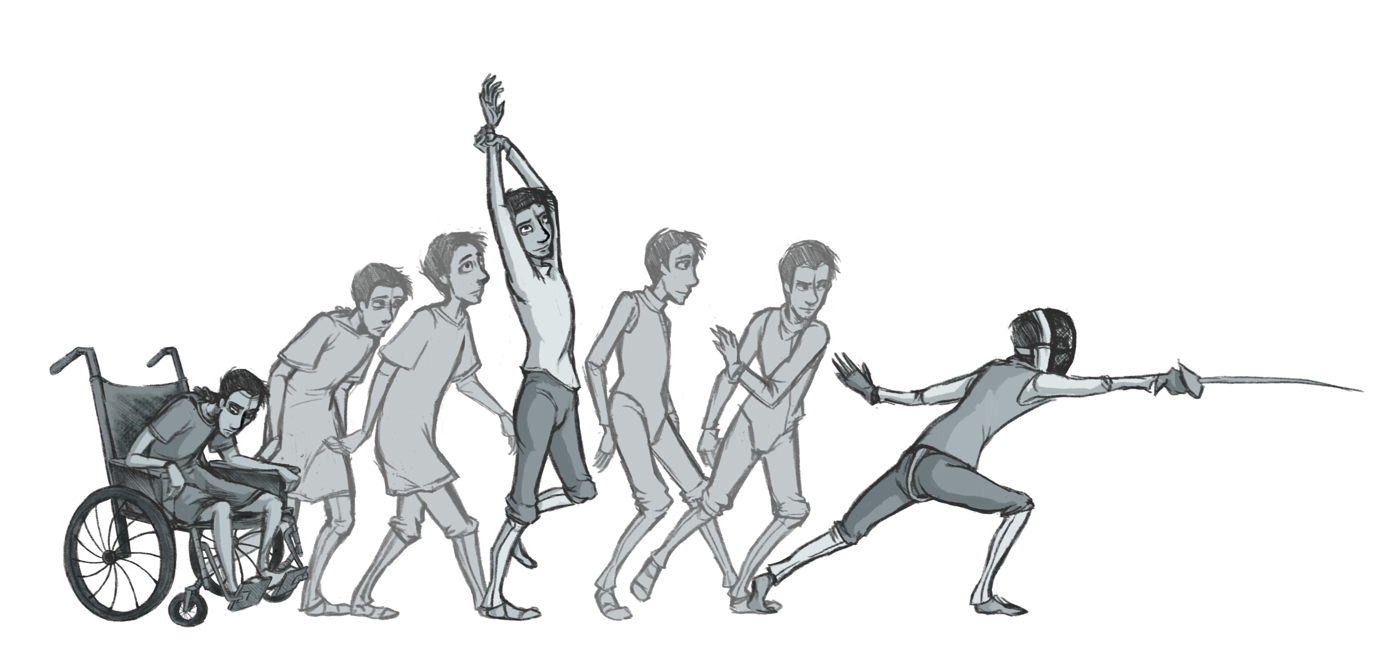
From motor impairment to expertise (Image credit: Kat McNally)
How is planning observed to be different in people with apraxia/stroke patients?
Apraxia is a confusing disorder that has long fallen into the space between cognition and action. Apraxia an interesting blanket term for a series of problems that humans have after predominantly left hemisphere strokes where they lose the ability to imitate gestures, pantomime the use of objects, and use tools.
One of the things we have studied was the ability of patients with left hemispheric damage to imitate meaningless gestures. Humans are great at watching and copying any gesture; if we didn’t have that ability there would be no choreography, for example. Even our closest relatives, the chimpanzees, are not very good at copying gestures. We found that the patients appear unable to t imagine or visualize the shape of the gesture they need to perform. The interesting possibility is that this may also underlie their difficulty in pantomiming and tool use. Thus by studying imitation we gain insight into the more abstract goal-level version of planning.
In what situations do learning systems compete?
There’s something quite spooky that we found, which is that there is a ‘winner-takes-all’ effect when the brain has more than one learning algorithm to solve a task. We report in several papers that, depending on how you frame the same challenge, you can favour and weight one learning system over another. Even if the learning system in question is broken, in patients with cerebellar ataxia for example, and when they have another learning system that is intact that can substitute in for it, they still don’t use it! In other words, they keep on using their broken system rather than switching over to the intact learning system.
We published a paper few years ago investigating why one may persist in using a particular learning system, almost as it is a learning habit. A particular framing of the problem seems like it’s good food for one learning system. As soon as that learning system is not working very well, you’d think that there would be a way to hand it over to another one. But that’s quite difficult. Through a third-party experimentalist, one could start to reframe the problem to weight towards the intact learning system. But can the patients do it on their own? In our study, we showed that even if we have the patients realise they have an intact learning system that can control the task, as soon as we take away the proper framing, they revert to the habit of the broken learning system. This is slightly discouraging.
We have here a credit-assignment problem and a learning partitioning problem. For example, there’s the alien hand syndrome: you could get a stroke in a certain part of the brain which produces a disconnection. When you reach for a glass of water, one hand will try to reach and the other hand will try to stop it. You can actually watch a competition between the two hands in the same person. That’s a quite dramatic precedent for showing competition between two systems. What we’ve been looking at are conditions under which patients fail to switch learning systems even though they have one available to switch to.
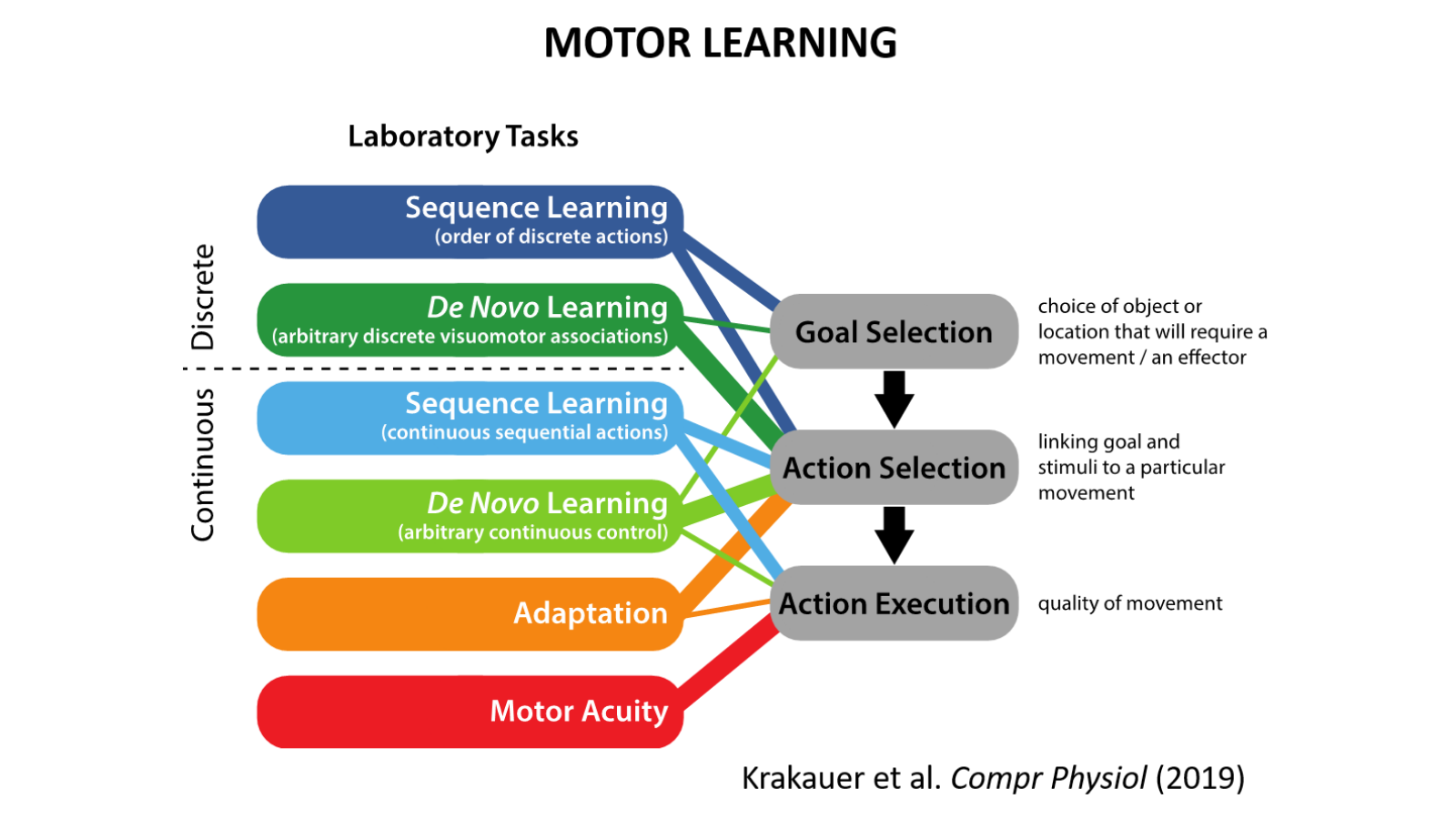
Who was Patient HM and how has this case traditionally been interpreted? In your opinion, what was missed from that interpretation and how can we rethink this famous case?
Henry Molaison (HM) was a famous patient who had intractable epilepsy and underwent bilateral temporal lobectomy in 1953 to surgically prevent his seizures. After the surgery he was found to have a profound, mainly anterograde, amnesia: he forgot episodes ten minutes after they happened. He’d say hello to you each day as if meeting you for the first time. This amnesia syndrome led him to be studied extensively up to his death in 2008. In one seminal study, the neuropsychologist Brenda Milner had HM learn to mirror-draw. For example, he had to trace in a mirror the double perimeter of a star. Milner found that HM acquired increasing skill at mirror-drawing across days even though each day, he said he’d never done it before. He was not even familiar with the apparatus. This profound finding showed that there was a disassociation between procedural memory and declarative memory.
Because mirror-drawing was a motor skill task of a sort, it led to the idea that learning motor skills doesn’t require declarative memory. This meant that skill learning became synonymous with procedural memory, which you could argue reinforces the notion of the dumb jock: ‘You don’t have to think very much.’ With a philosopher at Yale, Jason Stanley, I wrote a piece saying that motor skills do depend on declarative knowledge. Indeed, we wrote an article in the New York Times called “Is the Dumb Jock Really a Nerd?”
It was thought that HM had no memory or no knowledge of having done the mirror-drawing task, but was good at it; that he simply watched himself be good at it. But we don’t think this is correct. Our interpretation was that he listened to instructions and used explicit strategies to know that there was a mirror. He used that explicit knowledge to come up with a way to do well but then he forgot that he had thought about it. But that does not mean he wasn’t being declarative at the time.
What we’re arguing for is that you have an explicit scaffold and from that you automatise. Once you’ve automatised, you can take away the training wheels and the explicit scaffold. But you must not make a mistake and say, because they don’t remember using an explicit scaffold the next day, doesn’t mean they didn’t use one. In a way what happened was that the original interpreters of the study conflated the use of declarative knowledge with remembering the use of declarative knowledge. Based on that interpretation of the data and our own work, we feel that skills, even of the HM kind, are learned by an injection of knowledge and subsequent automatization.
An example I always give is your ATM number. Let’s say you have a six-figure ATM passcode. First you have to know it and actually remember it, but after you’ve been to an ATM twenty times, your finger just does it. In fact, I can put in my six-figure ATM passcode but I can’t tell you what it is!
Another example is people who are really fast typists. If you ask them, ‘draw out your keyboard for me, show me all the positions of the keys without practicing in your head or imagining moving your fingers,’ most people will not be able to draw out the QWERTY keyboard even though their fingers race across it. That’s another example of the disassociation between your ability to use the QWERTY keyboard and knowing where everything is. But again, to get to that point of automatised typing, at the beginning you had to go through the laborious scaffold of actually looking at all the keys and thinking about what you’re going to do.
In a nutshell, the way to think about HM is that it’s not about having a procedural system from the get-go. It’s about a transition from an explicit system that then proceduralises.
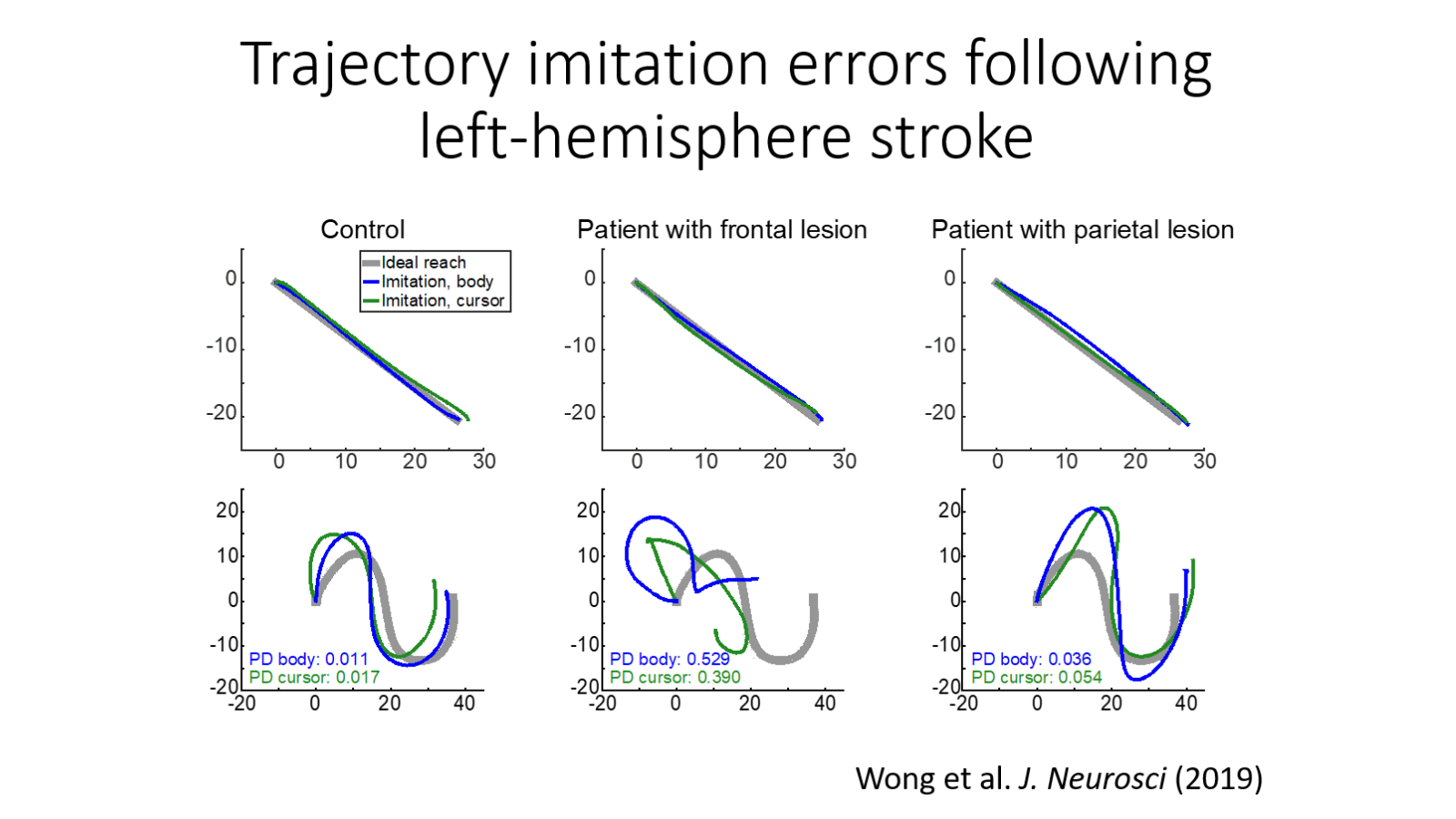
How do you use Marr’s three levels of understanding, as described in your 2017 Neuron paper, in interpreting the results from your own research? Do we understand the cognitive-motor interface in the brain, and what more needs to be done to boost our understanding of it?
The Marrian notion of the computational, algorithmic, and implementational levels can be applied just as easily to a dendrite or to an axon as it can to cognition. It’s better to think about Marr’s three levels as levels of realisation and that you can be computational, algorithmic, and implementational for any structure or behaviour.
The way we use Marr’s idea is that when we’re talking about understanding something, we’re actually asking an algorithmic question. What are the steps from an input to the requisite behaviour? In the 2017 paper, we argued that if you want to get an algorithmic understanding of the brain, you should directly observe behaviour. Only after the algorithmic characterization should one address the implementational level.
In a recent article in Quanta magazine you said, “sometimes an overly broad, vague use of a term can have detrimental effects on the types of experiments and hypotheses we generate.” Have you seen that be the case in studies of the motor cortex/domain?
Terms like ‘error’ or ‘skill’ are so vague that you can end up calling anything that generates a learning curve across trials ‘learning.’ I’ve always been a splitter rather than a lumper. For example, it’s useful to ask what kind of error: a prediction error, sensory prediction error, or target error? It’s also useful to break down ‘skill’ into the ability to select the right action given the context. For example, a drop shot is a good action to select when an opponent is behind the baseline. That’s skilful selection. And then you need the skill to execute it fast. There’s no point in deciding to play a drop shot, and taking so long that the opponent has already gotten back up to the net. And thirdly you need to execute it right. You might have the idea quickly, but execute it poorly by hitting the net. So ‘skill’ needs to be split into: selection of the right action, retrieval of it at very short latency, then accurate and precise execution of it.
It’s much better to partition behaviours into components that actually increase your chances of cutting and carving nature at its real joints rather than vague blanket terms based on intuitions that may end up taking us in the wrong direction.
For example, in the case of HM, the very impoverished notion of motor skill deriving from mirror drawing does not apply to a game of tennis. If Nadal or Federer had no declarative memory, they would not be able to learn tennis, or even necessarily play it very well had they learned it before HM-like amnesia.
These are examples of how folk psychological terms can lead one astray. And even in actual psychology, as that Quanta article talked about, there may be some terms that are so nebulous and abstract that it may never be possible to get any experimental traction on them.
Could you elaborate on your work with MSquare Health and KATA: how did they come about and what was your motivation for getting involved?
They came about out of an awareness, which I wrote about in a book three years ago, that a century of work in nonhuman primates and rodents showed that there can be quite remarkable gains after high-intensity, high-dose training very early following brain injury. In addition, there was the awareness about the benefit of enriched environments. When rodents are placed with toys, places to explore, friends, ramps, spinning wheels, etc, you see a lot more plastic change in their brain, compared to when the rodents are in isolation training on a boring task.
The question then becomes: how do we combine 75 years of research on enrichment in rodents and 100 years of work on recovery in nonhuman primates, and take that knowledge and translate it to humans? Our intuitive way of translating it to humans was to develop a proprietary, novel form of animation, which we call neuroanimation, where you combine enrichment and training together in a video game. KATA was the design studio that I helped set up at Hopkins, and MSquare was the start-up company that we spun-out to robustify, productise and commercialise the initial insights.
What are some technologies you are excited about for neuro-restorative behaviour training targeting neurological conditions and aging?
I’m biased in believing that we’re entering an era of digital therapeutics, the notion of software as a pill. The doctor would describe video games just as naturally as they prescribe drugs or perform surgeries. For chronic conditions in general, and neurological conditions in particular, the behavioural platform will be the most important. Riding on top of the behavioural platform would be drugs and invasive approaches. But none of these adjuncts will work unless the immersive digital therapeutic intervention is worked out first, which is what we’re working on.
What is the next piece of the puzzle your research is going to focus on?
There are so many different areas! We’re developing new ways to train the hand, and working with a group in Pittsburgh to study the application of implantable epidural electrodes into the cervical spinal cord to treat stroke. I just got a grant, along with the neuroscientist Stuart Baker to study a new nonhuman primate model of stroke in England. We’re beginning to do studies of long-term skill learning, along with my brother at the Santa Fe Institute.
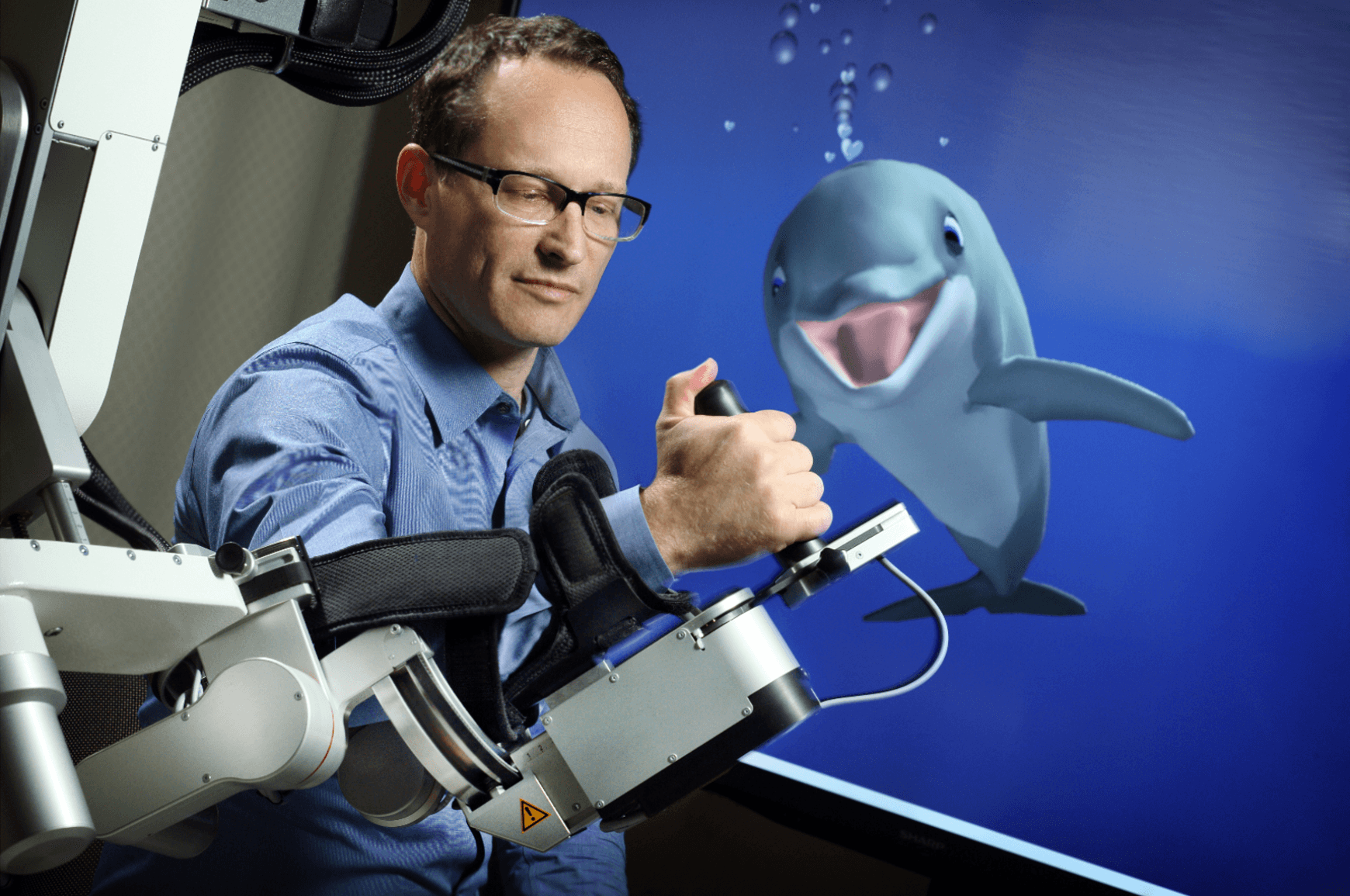
About Dr John Krakauer
Dr Krakauer is currently John C. Malone Professor, Professor of Neurology, Neuroscience, and Physical Medicine and Rehabilitation, and Director of the Brain, Learning, Animation, and Movement Lab (www.BLAM-lab.org).
His areas of research interest are: (1) Experimental and computational studies of motor control and motor learning in humans (2) Tracking long-term motor skill learning and its relation to higher cognitive processes such as decision-making. (3) Prediction of motor recovery after stroke (4) Mechanisms of motor recovery after stroke in mouse and non-human primate models, as well as in humans (5) New technology-based neuro-restorative behavioral training for neurological conditions and aging.
Dr Krakauer is also co-founder of the company MSquare Health and of the creative engineering Hopkins-based project named KATA. KATA and MSquare are both predicated on the idea that animal movement based on real physics is highly pleasurable and that this pleasure is hugely heightened when the animal movement is under the control of our own movements. A simulated dolphin and other cetaceans developed by KATA has led to a therapeutic game that has been interfaced with an exoskeletal robot in a multi-site rehabilitation trial for early stroke recovery, and with motion tracking for cognitive therapy in the normal aged. Dr Krakauer’s book, “Broken Movement: The Neurobiology of Motor Recovery after Stroke” was published by the MIT Press in November 2017.