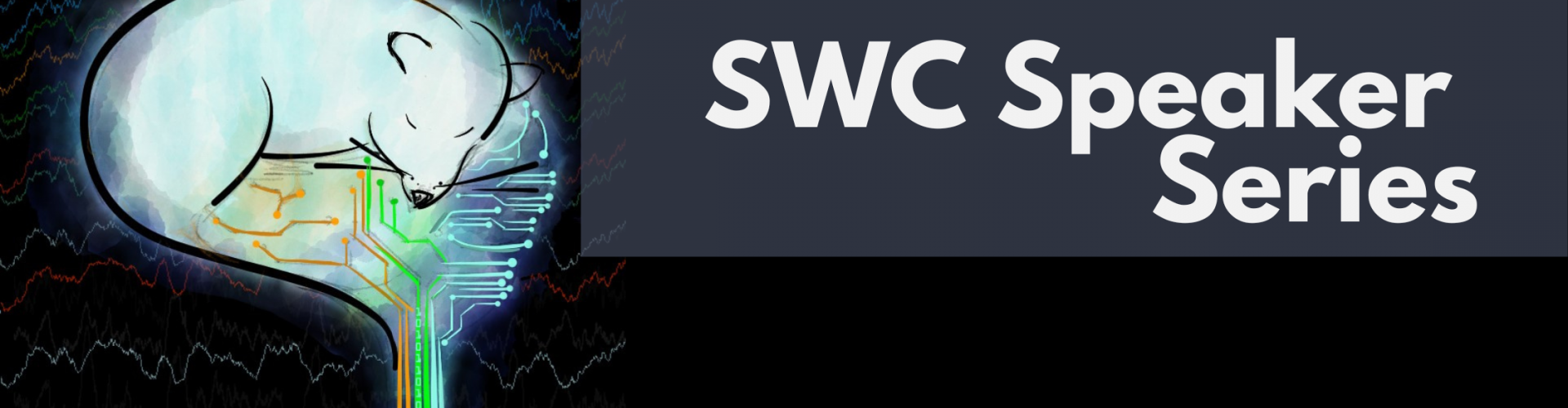
Cross-area coupling and consolidation during sleep
An interview with Dr Karunesh Ganguly, UCSF and the San Francisco VA Medical Center, conducted by Hyewon Kim
How do brain areas talk to each other during sleep to enable us to wake up one morning and realise we’ve gotten better at a new motor skill like biking or skiing? In a SWC Seminar, Dr Karunesh Ganguly discussed his work uncovering a dialogue between cortical and subcortical areas leading to memory consolidation during skill learning. In this Q&A, he goes into detail about what has been historically known about the neural basis of consolidation during sleep, translational implications of his work, next steps, and more.
What first sparked your interest in skill learning and how activity patterns in the motor network drive skill learning?
It goes back to my undergraduate days when I took some psychology courses and was learning about how stroke can result in syndromes like neglect, movement disorders and memory deficits. This got me interested in understanding the effects of brain lesion on behaviour. It also got me really interested in neuroplasticity and how it drives recovery.
Then, I started an MD-PhD programme. My PhD work was more abstract and cellular, but when I went into neurology training, I saw that a big part of neurology is about deficits in movement. I learned that we know a lot about the anatomy of weakess and deficits, but very little about the physiology of how the network responded. This seemed like an area of interest, a gap in our knowledge.
At the same time, there had been work on brain machine interfaces trying to make movement better. It was exciting to pursue the basic understanding of learning to control movement and, in parallel, how movements go wrong and can be made better.
So bit by bit, this area of study seemed to fit what I was doing from a clinical and translational perspective. It wasn’t all planned, but these experiences came together.
Why is it important to understand systems consolidation or how new memories are integrated into cortical and subcortical networks over time?
Even a century ago, it was clear that sleep played a role in the learning and recall of information. Over the past decades the field of neuroscience has really elucidated how neural networks can create long-term representations. A key principle has been the idea of ‘systems consolidation’, where memories are represented in different networks over time. This is also probably important for things that we take for granted, e.g. general knowledge and understanding that is subconscious. More recently, we have been able to manipulate the processing to create forgetting or to even strengthen memories. All of this knowledge is helping us fashion new ways to improve recovery after injury. We hope that over the next decade this understanding will lead to new therapies.
How much is known historically about the neural basis of memory consolidation and sleep?
The phenomenon of sleep altering memory has been known for over a century. Thereotical work in the 1970s from David Marr suggested that ‘neural replay’, or reinstating awake patterns during periods of rest or sleep, might be important. In the 1990s, it became clear that this was indeed the case in animal models. There was also a lot of work in human subjects showing the importance of sleep for memory consolidation. Growing work now shows that this is causal, that is we can manipulate it to strengthen or weaken memories. Ongoing work aims to understand different memory systems, for example memories about facts or movements. Also, a key area of uncertainty is precisely how different brain areas contribute. Also, it is unclear how the brain decides what to remember or what to forget.
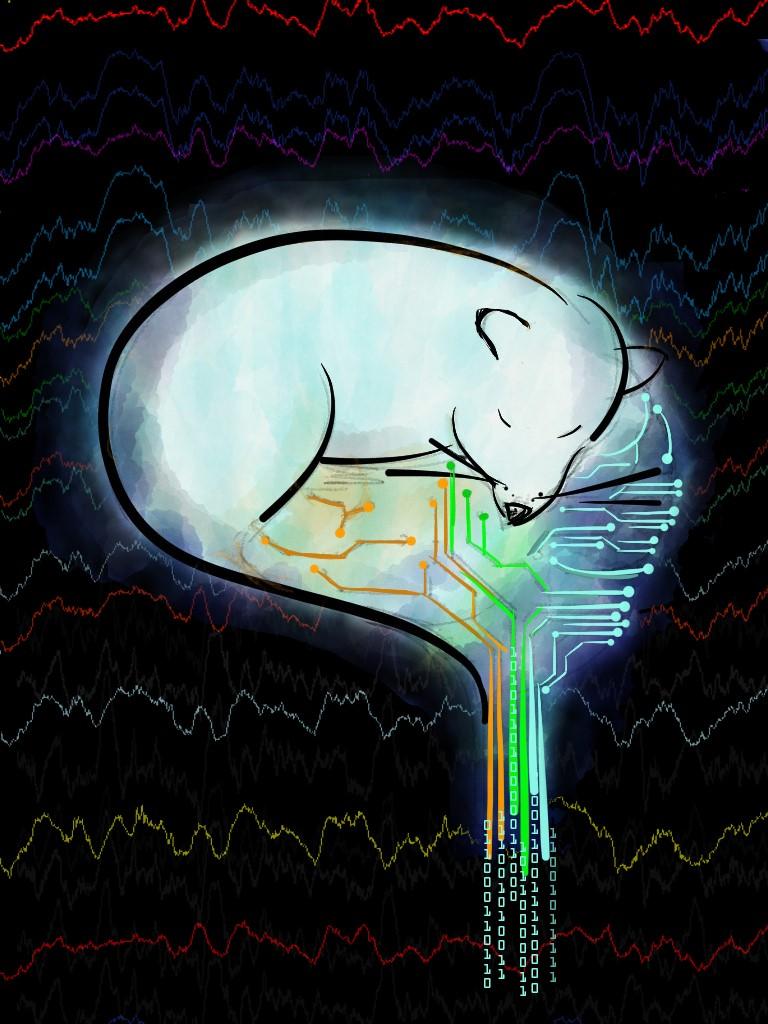
Image by Chelsea Wong
Why do you look at cross-area coupling during Non-Rapid Eye Movement Sleep (NREMS)?
One of the things that interested me about sleep was NREMS, which involves whole brain processing. For example, during NREMS, most parts of the brain are in synchrony and moving together in an up and down manner. In other words, the brain is being turned on and off at fast timescales. What we did not understand is how the content of experiences is being processed. We have focused on how NREMS processes information and leads to changes in cross-area coupling.
What would you say are some of your key findings so far?
Learning a skill is known to have an early cognitive phase and then a slower motor phase. It was also known that multiple brain areas (e.g. the hippocampus/HC, prefrontal cortex/mPFC and motor cortex/M1) are involved. Our early work focused on understand how M1 activity changed during learning. This involved recording activity in both sleep and awake periods. We also focused on how motor areas downstream of M1 changed. We were also able to manipulate sleep processing to make animals forget the task or even get better at the task upon awakening.
Our most recent efforts have tried to understand how the broader brain network drives skill learning. The plot below shows our current view on how processing during sleep changes across brain areas with practice. We have found that there are apparent transfers from cognitive areas (HC, mPFC) to motor areas (M1) during sleep. This transfer seems to be related to when each animal ‘gets’ the task. After this, we see the slower motor learning phase kick in (‘manifold consolidation’). Prior to this the animals seem to be exploring how to do the task. Thus, we think that NREM sleep is used to replay past activity to determine whether a skill should be formed, or instead allow an animal to remain in an early state of exploratory learning.
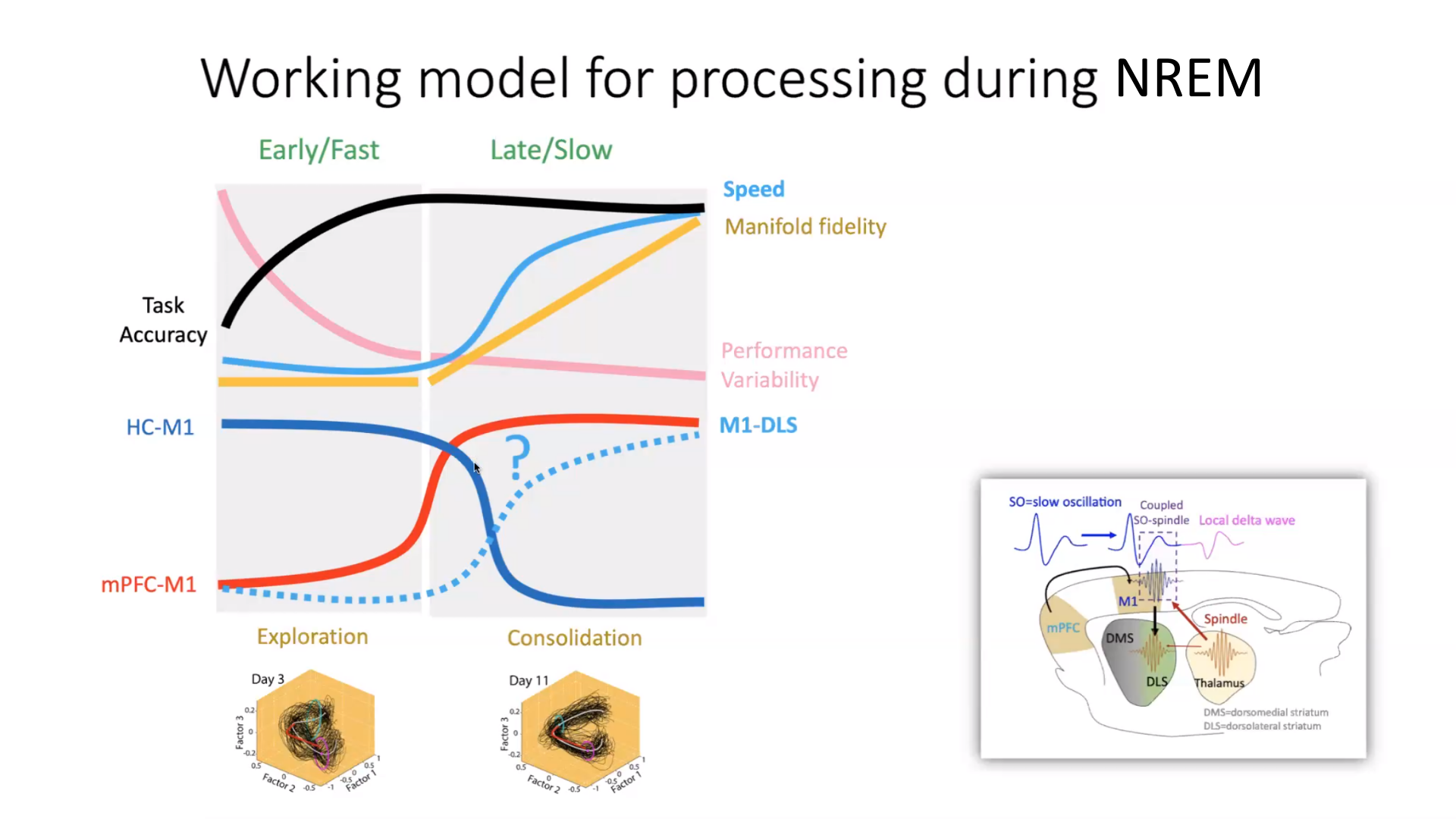
And what is the task that the rats had to do in your experiments?
They have to reach and grab a pellet. For a primate, reaching an apple or a ball would be very trivial. But for rodents, it's not as natural and counts as a skill. For a rat, it would take about 1 to 2 weeks to master this to the point where it looks fluid. The rats are also learning the cognitive aspects of the task: the cue, the time of door opening, the location of the pellet. This same task also helps us understand motor recovery. One of the big deficits after stroke is loss of dexterity in grasping and reaching. So this task has allowed us to understand both normal learning and recovery.
On top of what you already discussed, what are the implications of your research?
One of the things that we've been excited about is that our results suggest there's certain rhythmicity in sleep, a low frequency oscillation. What's striking is that we found the same patterns during awake processing. For example, similar low frequency patterns are disrupted in stroke during awake behaviour. We've found a new way to stimulate the brain using low frequency stimulation post-stroke. Thus, when we artificially create low-frequency patterns using electrical stimulation we can improve behaviour after stroke.
What's the next piece of the puzzle of your research that you're excited to work on?
For a long time, the field of neuroscience has been studying each area in isolation. However, it is also well known that the brain achieves its complexity because of the presence of multiple areas that dynamically work by themselves and together. Over the past 5-10 years, it has become possible to record from and manipulate activity across multiple brain areas. This approach will hopefully allow us to really understand how the brain processes information and allows truly complex behaviours. Also, because recovery after stroke involves compensation across the remaining network, this same approach is very promising for further refining brain stimulation approaches to improve recovery.
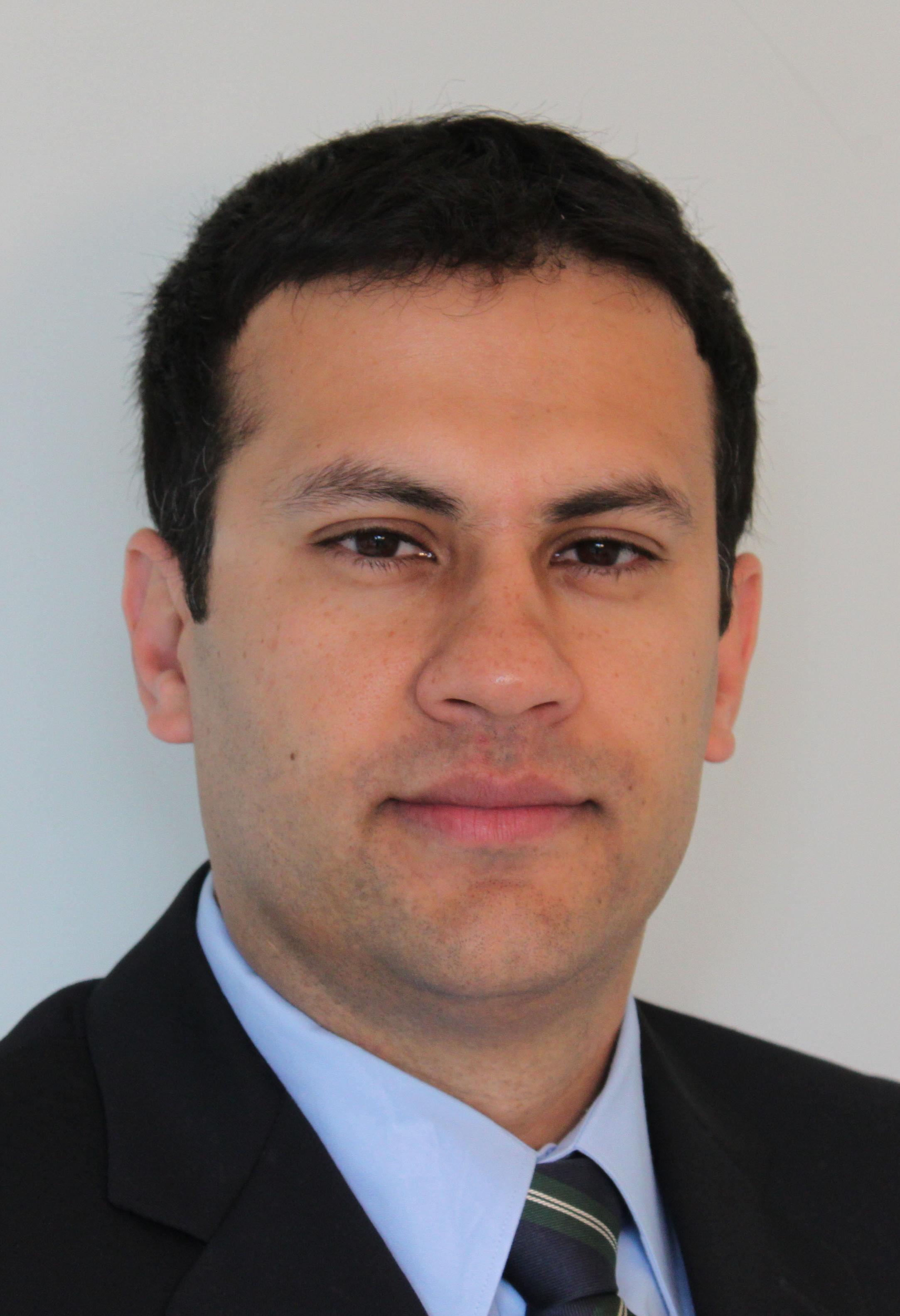
About Karunesh Ganguly
Karunesh Ganguly MD, PhD is a neurologist and scientist at UCSF and the San Francisco VA Medical Center. He graduated from Stanford University and then completed his MD/PhD degrees through the Medical Scientist Training Program at UCSD. Concurrent with his residency at UCSF, he conducted research into the development of ‘Brain-Machine Interfaces’ in the Department of Electrical Engineering at UC Berkeley. He is currently the Director of the Neural Engineering & Plasticity Lab at UCSF. The laboratory’s research program focuses on the development of neural interfaces to augment neural recovery. A key related focus is to understand how activity dependent processing drives new skill learning and recovery of motor function after stroke. This includes understanding how activity across multiple timescales, both during task learning and during offline periods, drives learning and consolidation.