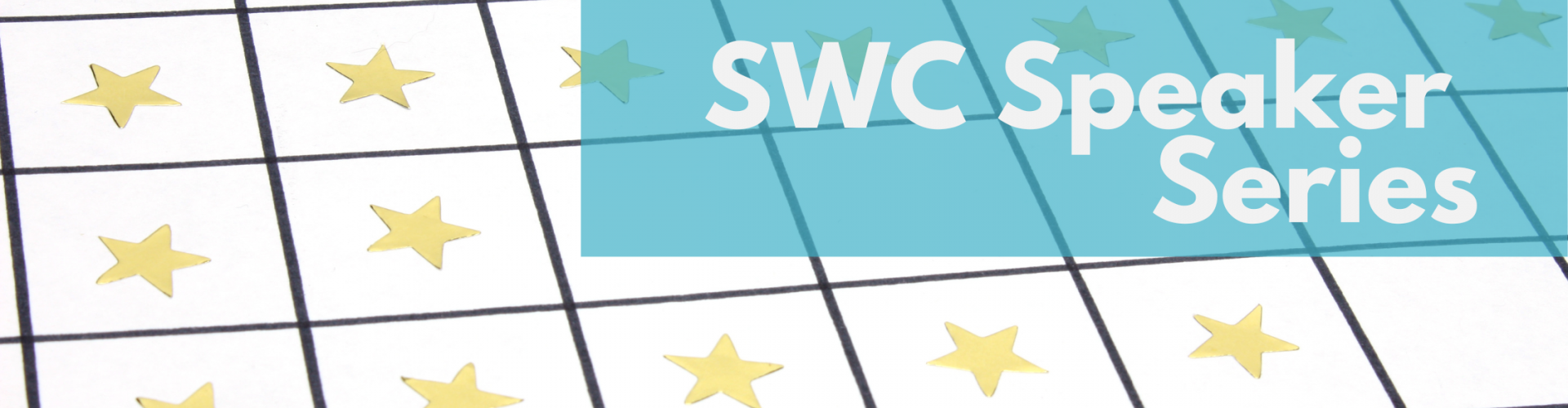
Examining neural mechanisms of reinforcement learning
An interview with Professor Ilana Witten, Princeton University, conducted by Hyewon Kim
Imagine you have a delightful experience at a new restaurant – the dish is perfectly seasoned and the atmosphere great – and you decide to return in the future. At another restaurant you find the food is oversalted, cup stained, and service rude. You decide that one visit is enough. How does the brain use the presence or absence of reward to influence its decision-making? Recent SWC Seminar speaker Professor Ilana Witten shares her research on the midbrain dopamine system, its role in reinforcement learning, and state-dependent decision-making in the striatum.
Can you share some examples of reinforcement learning during decision-making?
We are constantly getting reinforcement for our decisions. We could be getting reinforcement based on whether a food tasted good, if we decide we want to go back to that restaurant. Or we could get positive or negative reinforcement from social interactions, whether someone laughed at our joke or rolled their eyes.
How much is already known about state-dependent decision-making mechanisms and what remains unknown?
The idea that there are different brain states is a very old concept. Sleep, hunger, and arousal are all old concepts of brain states. What is new about our research is that we showed that manipulating striatal neurons contributes to decision-making only in one brain state, but not others. This means the neural basis of decision-making varies across states.
Why has dopamine in the ventral tegmental area (VTA) been paid so much attention in the context of reinforcement learning?
It has been shown that dopamine neurons encode an error when predicting reward. In other words, they respond when unexpected rewards happen, but not when a reward is predicted. This has been such a central finding because reward prediction errors are very important for reinforcement learning algorithms. So, they provide a clear hypothesis about how learning algorithms map onto brain circuitry.
What did you do to find out what the heterogeneity in the VTA dopamine system means?
Our lab and other labs have found a lot of unexpected heterogeneity in the dopamine system, in terms of what aspects of signals are easy to immediately reconcile with reward prediction error, in other words being a teaching signal to support learning.
We trained mice on a virtual reality T-maze, where they were rewarded for choosing to go in the direction where there were more visual cues. Ben Engelhard, a postdoc in the lab, imaged from large populations of dopamine neurons during this task. What he found was surprising levels of heterogeneity at the single neuron level, particularly during the ‘cue period’ – when they are seeing randomly appearing cues on the left and the right. Subsets of dopamine neurons encoded subsets of different features, including position, speed, kinematics, view angles, cues, and task accuracy. But during the outcome period, the responses across neurons were much more homogeneous.
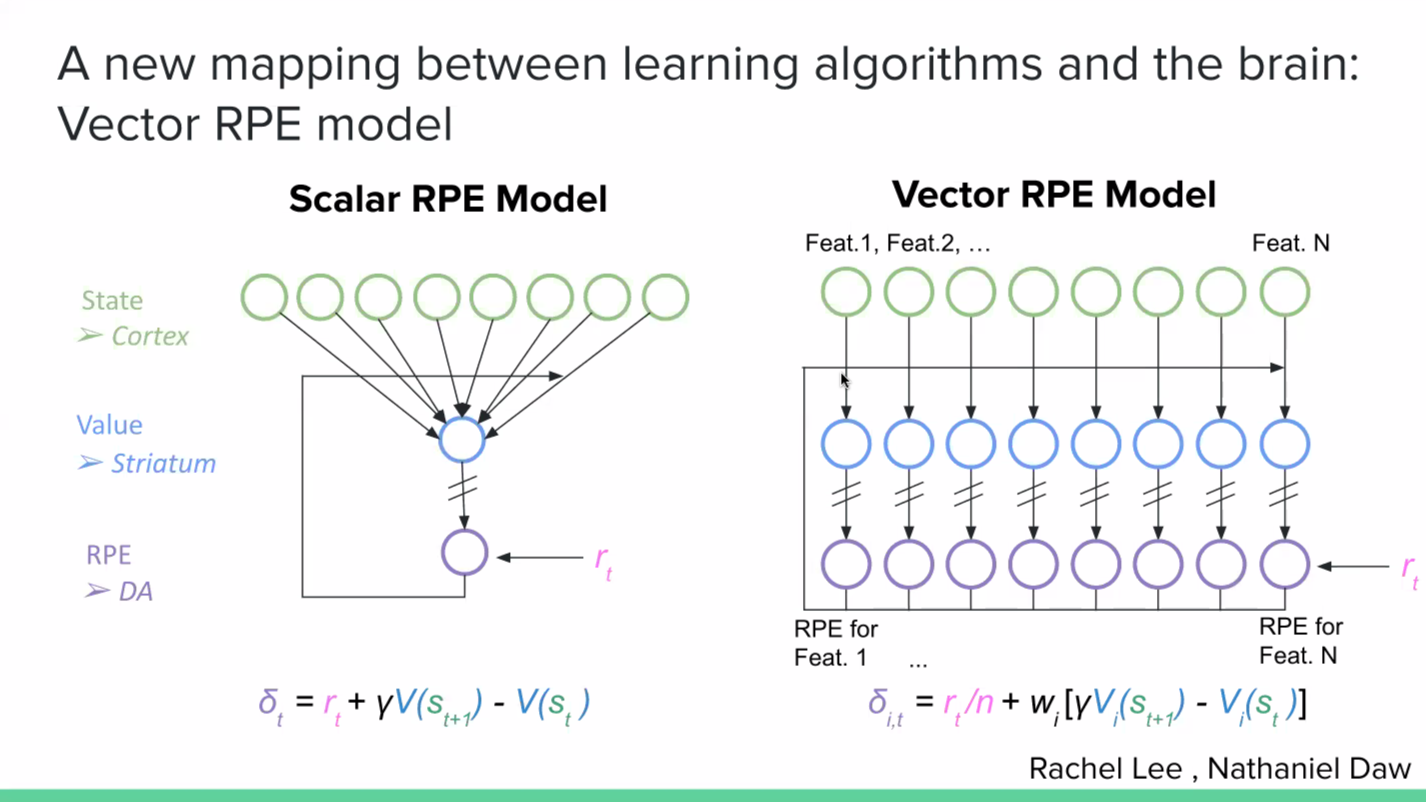
It is not immediately obvious how to reconcile this data with the traditional ‘scalar’ reward prediction error model. A new model that we have been working on to make sense of this data is the ‘vector’ reward prediction error model. Here, dopamine is a vector, rather than being a scalar signal. The dopamine neurons still calculate reward prediction errors based on the value representations in striatum, but rather than thinking of value just as a single scalar, you can think of different striatal neurons calculating values based on different cortical neurons. And the dopamine neurons are calculating reward prediction errors from those different striatal neurons. So if some neurons encode motor variables while others encode position variables, you could end up with dopamine neurons that are only that subset of the state feature space, and therefore only calculating reward prediction errors with respect to those features.
A major open question in the field is how to make sense of this diversity. What I spoke about in my talk at SWC, which is a collaboration with Nathaniel Daw’s lab at Princeton with a joint PhD student Rachel Lee, is the idea that the brain uses a set of features to predict reward. And if you look at individual dopamine neurons rather than the average of the dopamine neurons, that heterogeneity is due to the fact that different dopamine neurons are using a subset of the overall feature space to predict reward. So, you see the feature space used for reward prediction leaking into the dopamine population code.
How did you test the vector reward prediction error (RPE) model? What did you find?
As a way of testing what predictions this model would make in the context of our task, we simulated the vector RPE model by training a deep reinforcement learning network on the task. The network basically did the same exact task as the mice and received the visual input of the maze, which got passed to convolutional layers, and then into an actor-critic model, where it had to perform action selection just like the mice. The way it made a decision was based on calculating a vector of reward prediction errors, using that vector to reweigh all the weights in the network to predict value, and taking the optimal action.
The heterogeneity was very apparent in the vector RPEs from the agent. Similar to what we saw in the neural data, we got different ‘neurons’, or units, tuned to different view angles of the agent, its positions, and the presence of left versus right-side cues. The basic concept of why we see this is because each individual neuron, rather than calculating RPE on the entire state space, only sees a subset of that state space. And by only seeing a subset of that state space, it can make idiosyncratic choices about how the agent predicts reward, even though those choices actually end up averaging out from the global signal.
And on top of just predicting heterogeneity, what this model shows is that these aren’t just sensory responses to these features, but actually prediction errors with respect to these features. What that means is that for neurons that respond to cues, they are responding not just to the presence or absence of the cue, but to whether the cue makes the reward more likely (positive RPE; confirmatory), or less likely (negative RPE; disconfirmatory).
Were you surprised to find that the vector RPE model produced results that closely matched the data?
Yes and no. I was surprised that it made new predictions that we were able to support and surprised by how much of the data that the model could explain. But on the other hand, our model was designed to explain the data, and it was inspired by the data.
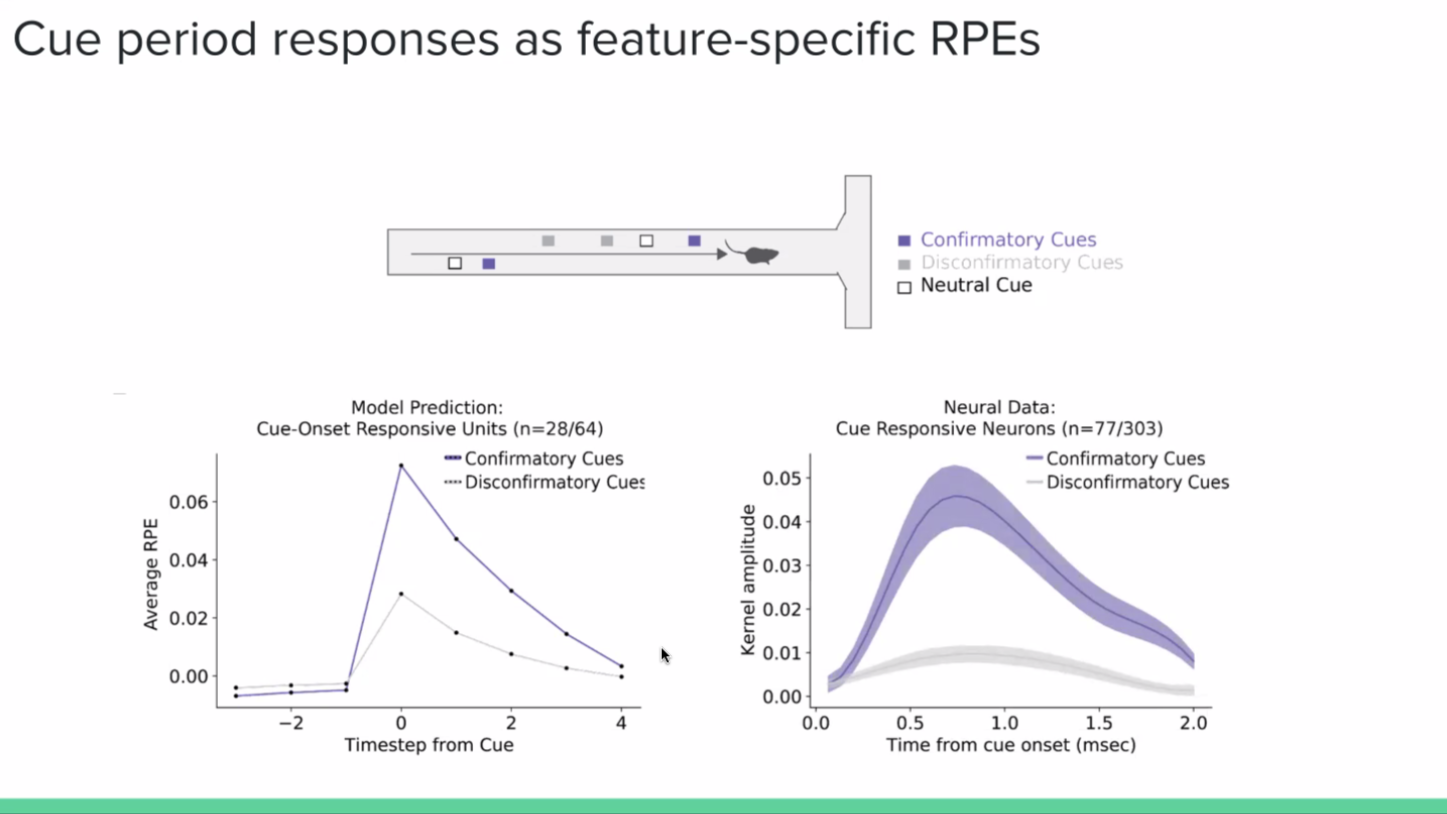
Why did you look at how aggressor mice influence stress in individual mice, and how individual mice use different behavioural strategies in response to social stress?
If the above reward vector RPE is correct, then this heterogeneity in the dopamine system is telling us something interesting about how the brain is doing reinforcement learning. In other words, the heterogeneity is a reflection of the so-called state representation and the features that are being used by the brain to predict reward. In general, in state representations, it’s hard to know what features of a high-dimensional and realistic environment an animal is using to predict reward. What’s cool about this model is that the heterogeneity across the population of dopamine neurons, the vector, basically tells you the feature space that’s being used to predict reward. One thing we’re working on now is changing the task demands or monitoring changes in an animal’s behavioural strategy to see if that produces the predicted changes in the vector RPE.
This model also makes predictions not only about neural activity within an individual animal, but also about activity differences across individuals. In other words, if different individuals are using different behavioural strategies, that should predict very specific differences that reflect those strategies in the dopamine signal across individuals. Individual differences in behavioural strategy could be another source of heterogeneity in the dopamine system. So we wanted to ask, does dopaminergic heterogeneity across individuals reflect the features each individual uses to predict reward, in other words, an individual’s behavioural strategy?
What did you do to answer this question and what did you find?
I partnered with Annegret Falkner, also at the Princeton Neuroscience Institute, to use a chronic stress paradigm. Many of us are exposed to stress, especially in this day and age, from a number of different sources, and there is a large spectrum of how differently people respond to stress. For example, some develop psychiatric symptoms while others seem to be resilient to the same source of stress.
Chronic stress can be modelled in mice in a compelling way in the form of a social stress paradigm. Mice are exposed to a social stressor, for five minutes a day across multiple days where every day they get a new aggressor mouse that is mean and stressful. In response to this stressor, some mice are so-called resilient – they don’t seem to display any changes in their behaviour across a number of measures compared to controls that are not exposed to this stress. And in about half of the mice (in our case a little bit less), they show susceptibility. This means they are anti-social, anhedonic, and show increased anxiety-like behaviour after the stressful encounter. They generalise from the stressful experience to fall into a state of low motivation and depression, as confirmed across a number of behavioural readouts.
The question that our PhD student Lindsay Willmore asked is, what is happening during stress and do susceptible and resilient mice have different behavioural strategies in response to that stress? If so, is that reflected in differences in their dopamine system? She tracked the behaviour of the aggressor and stressed mice and collected videos from a couple different angles, quantifying the postures of the animals. She turned these behaviours into an intuitive feature space – she selected about ten features from the angles and proximity between mice to calculate what is going on in the mice across time. To quantify the behaviour, she used a technique called t-SNE to produce clusters of behavioural strategies during the social interactions.
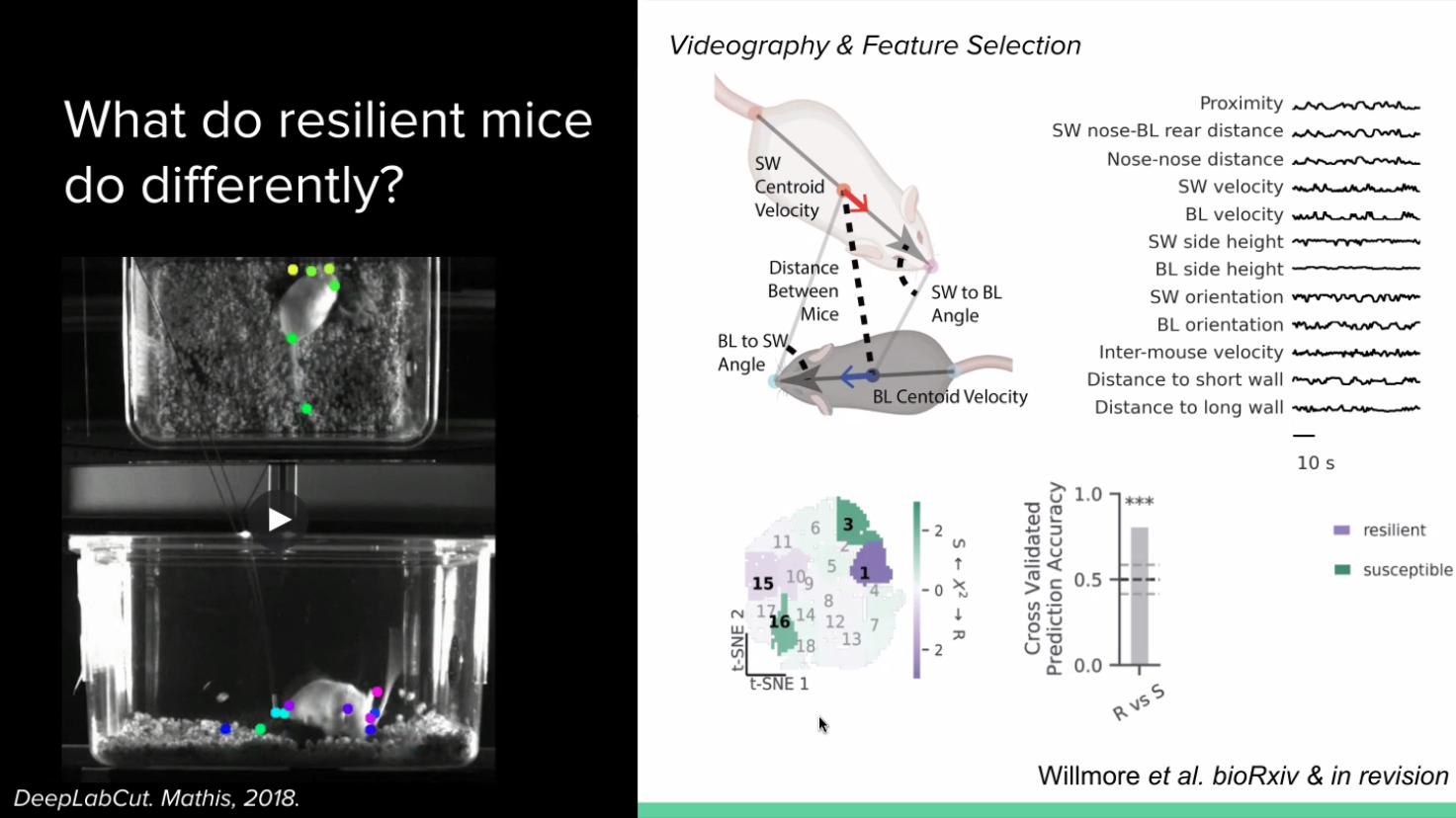
Lindsay then compared the cluster occupancies between the resilient and susceptible mice. She found that the resilient and susceptible mice take different strategies during defeat. She was also able to accurately predict the resilience or susceptibility of a mouse using these behavioural strategy cluster occupancies. Qualitatively, she found that when a stressed mouse fights back against the aggressor more, it becomes resilient.
Then, the question was – do these different behavioural strategies reflect different dopamine correlates? She found that indeed, the mice that fight back more and tend to be more resilient have more dopamine in the nucleus accumbens of the ventral striatum associated with the behaviour. This goes back to the idea that the heterogeneity in the dopamine system is a reflection of the state representation – which relates to the strategy that mice take in any environment.
Why were you interested in seeing how behavioural strategies change across time? What did you do to see whether different strategies have different underlying circuit mechanisms?
Thus far we were able to conclude that dopamine neurons can be heterogeneous in high dimensional tasks. And this heterogeneity may reflect the features being used to predict reward and provide a window towards understanding individual differences. We then wanted to understand how behavioural strategies change across time and whether different strategies have different underlying circuit mechanisms. We therefore shifted our focus to striatal neurons, where the cortex projects to and from which projections go to the dopamine neurons.
The striatum is composed of two principal output pathways, which are the direct and indirect pathways. The classic view has been that the striatal pathways oppositely modulate behaviour, more specifically, movement. The direct pathway, called the “go” pathway, promotes behavioural output and the indirect pathway, the “no-go” pathway, suppresses behavioural output. And there have been a lot of influential optogenetic studies in the last decade supporting this classic view: if you activate the direct pathway, you get the animal to “go”, and if you activate the indirect pathway, the animal will “not go”.
But one thing that has been a bit curious in the field is that there have been a few reports of seeing effects from inhibiting these pathways. There have also been studies where the two pathways were activated but there were no opposite effects on behaviour, and where the pathways were recorded from and there were no opposite correlates of behaviour. So that has led to some people wondering if it is not as simple as there being two straightforward pathways. The question is then, what is the effect of naturally occurring activity on behaviour?
So what we did was inhibit the two pathways in the striatum, and see what effect it had on behaviour, and whether or not, and under what conditions, they produced opposite effects. At the big picture level, we wanted to understand how striatal neurons contribute to state-dependent decision making. The following work was done by Scott Bolkan and Iris Stone, in collaboration with Jonathan Pillow. Using optrodes, we could indeed inhibit the neurons in the respective pathways, but when we observed behavioural effects of a running animal going versus not going, we did not see anything. This was consistent with there not being publications saying that an animal stops or goes when you inhibit the two pathways.
We concluded that instead of the neurons having a direct effect on the action, perhaps they influence the decision on how to act or what to do. So we wanted to test the paradigm in the context of a decision-making task. We also wanted to vary the cognitive demand of the task – by employing three different T-mazes: a regular evidence accumulation task where the mouse turns to the side with more transiently available visual cues, a control task with the same design as the original but with no distractor cues on the opposite side, and another control task where the cues are permanently present.
We also used a generalised linear model (GLM) to predict the animal’s choice during evidence accumulation. And we hypothesised by looking at our data qualitatively that the behavioural strategies do not stay constant but change across time. We then applied the hidden Markov model (HMM) to the model to create different GLM weights at different points, or states, in time.
What did you find?
We found that the inhibition of the direct and indirect pathways of the striatum can cause big and opposite control of behaviour. But context matters. In the case of the dorsal medial striatum, it has to be a cognitively demanding task. And even within that task, mice go through different internal states and only one of them is sensitive to the striatal inhibition.
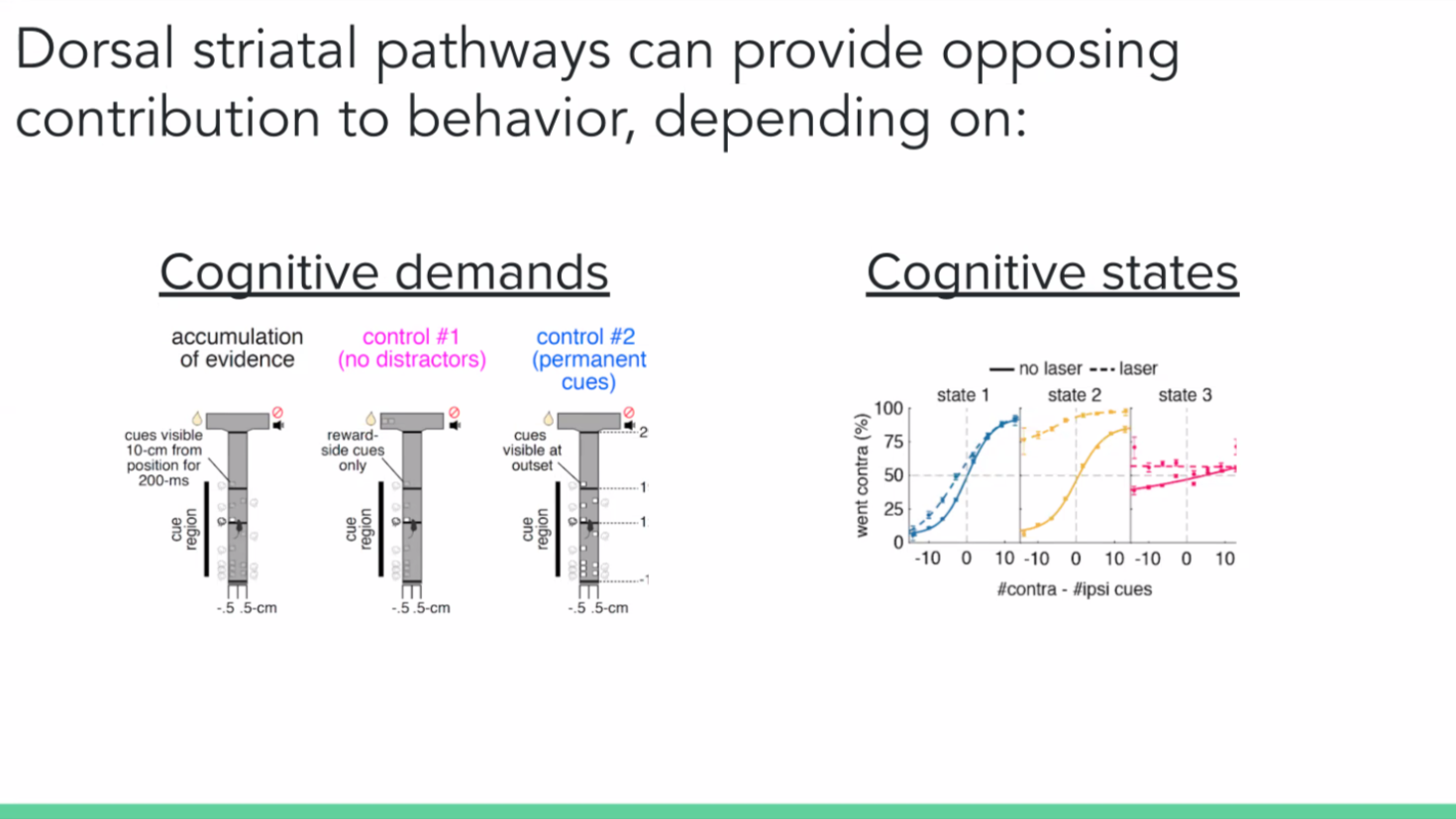
What implications do your research findings have for state-dependent decision-making and the use of behavioural strategies in humans?
I think what it ultimately means is that the same behavioural output does not imply the same circuit mechanism. There is this parallelisation in the brain – as true in the mouse and I’m sure is even more true in the human – where you can get similar-seeming behaviours through different mechanisms and circuitry.
What’s the next piece of the puzzle your research is focusing on?
For the state-dependent decision-making component, it’s understanding what is different about the different states in terms of neural correlates and the effect of inhibition on downstream regions.
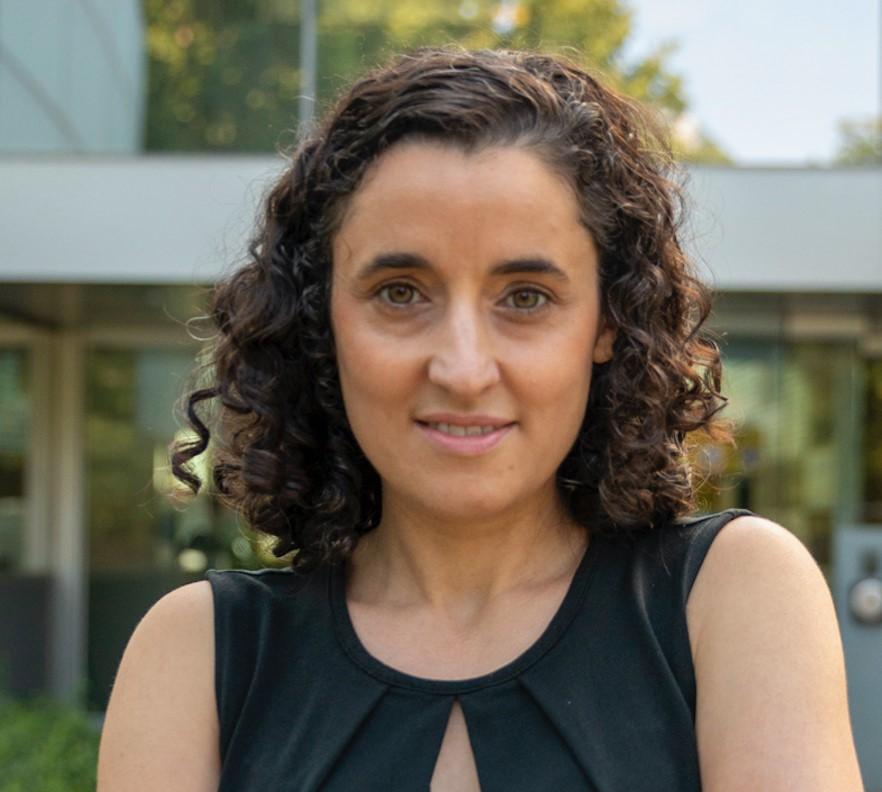
About Professor Ilana Witten
Ilana Witten is a professor of neuroscience and psychology at Princeton University. She was first introduced to neuroscience as an undergraduate physics major, when she studied neural coding in the retina with Michael Berry at Princeton. She then moved to Stanford to pursue a PhD in neuroscience, where she worked in the systems neuroscience lab of Eric Knudsen. As a postdoctoral fellow, she worked with Karl Deisseroth in the Department of Bioengineering at Stanford, developing and applying optogenetic tools to dissect the neuromodulatory control of reward behavior in rodents. Since 2012, her lab at the Neuroscience Institute and Department of Psychology at Princeton has focused on understanding the circuitry in the striatum that support reward learning and decision making. She has received multiple awards for her work, including an NIH New Innovator Award, a Mcknight Scholars Award, and the Daniel X Freedman Prize.