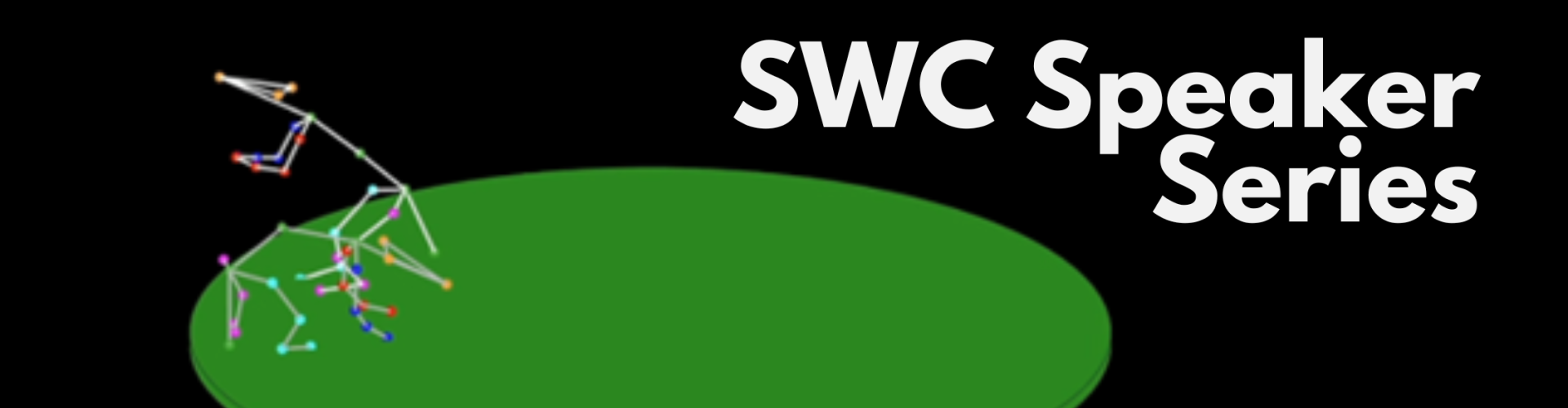
Tracking behaviour in 3D
An interview with Dr Timothy Dunn, Duke University, conducted by April Cashin-Garbutt
While we have increasingly advanced tools for recording neural activity, behavioural quantification remains an underdeveloped component of the modern neuroscience toolkit. In his recent SWC Seminar, Dr Timothy Dunn, an assistant professor of biomedical engineering at Duke University, presented a new approach for multi-animal 3D tracking and social behaviour analysis in rats. In this Q&A, Dr Dunn explains how his new approach differs from previous tools and the implications of 3D tracking on understanding autism and Parkinson’s.
What first sparked your interest in developing technologies for high-resolution movement quantification?
My PhD research involved the development and application of new technologies for large-scale neural recordings across expansive, brain-wide populations of neurons. As systems and computational neuroscientists, we’re generally trying to understand animal behaviour and explain its underlying computations in terms of neural activity. And recently the field has been trying to do this using recording from larger and larger populations of neurons.
But while we now have all of these amazing tools for neural activity measurements, our ability to measure behaviour has lagged far behind. This fundamentally limits our interpretation of large-scale neural recording data and our understanding of animal behaviour in general. It was clear to me that if we have high-resolution neural recording tools, we need high-resolution behavioural recording tools to go along with it.
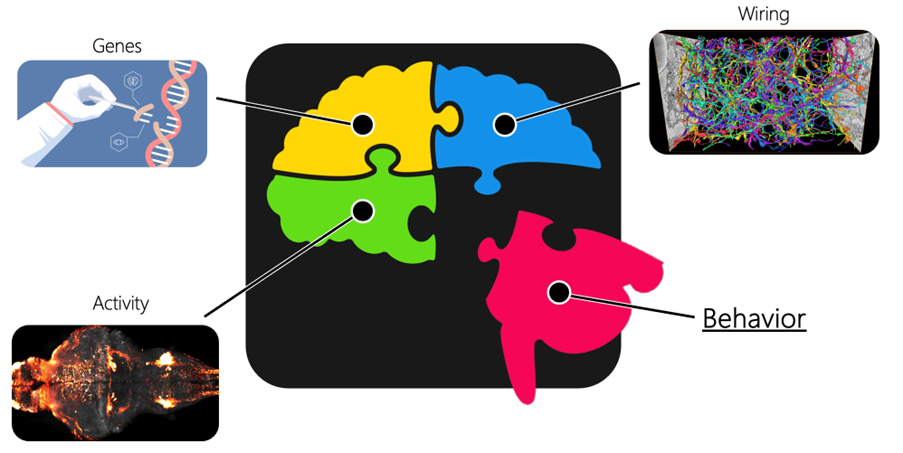
Why is advanced behavioural quantification an underdeveloped component of the modern neuroscience toolkit?
First, I think it’s because we have only recently had the powerful computer vision methods necessary to precisely track body movements in freely moving mammals. This is actually a surprisingly hard computer vision problem.
Also, the way we think about behaviour needs to change. We need to figure out how to deal with the massive influx and complexity of new high-resolution behaviour datasets. The scale of the problem is a little intimidating. You can collect these data. But then, what do you do with them? We need to continue to develop novel frameworks and analyses for working with these data and relating them to brain systems.
I do also think it has to do with how one can see behaviour with the naked eye. Connectomics, neural recordings, gene expression, these all require special instruments to resolve anything at all. So it’s understandable that these instruments were developed first. But I think this also led to a belief that behavioural quantification was trivial, and thus it wasn’t a major focus of innovation.
What are some of the key limitations of 2D pose tracking?
2D pose tracking is easier to deploy and is sufficient for many neuroscience lab applications. But 2D pose tracking is inherently limited in its precision and comprehensiveness, especially in freely moving animals.
There's a few reasons it’s limited. 2D pose tracking uses a single camera, which means you typically have one side of the body occluded from view. With 2D pose tracking, you’re going to miss what’s happening on the other side of the body.
If you’re using a top-down camera, which is a typical setup for doing 2D tracking, you cannot see what’s underneath the animal. You cannot consistently track its limb movements, if you can track them at all. And similarly, if you have a bottom-up 2D camera view, you're missing animal body movements when they are away from the floor of the arena. So there's a portion of the behavioural repertoire you cannot capture using a 2D technique.
There’s a separate geometric issue with use 2D pose tracking to capture bodies and movements in 3D space. Projecting three-dimensional shapes onto two-dimensional planes leads to measurement artefacts from perspective ambiguities. This fundamentally limits the precision of 2D measurements.
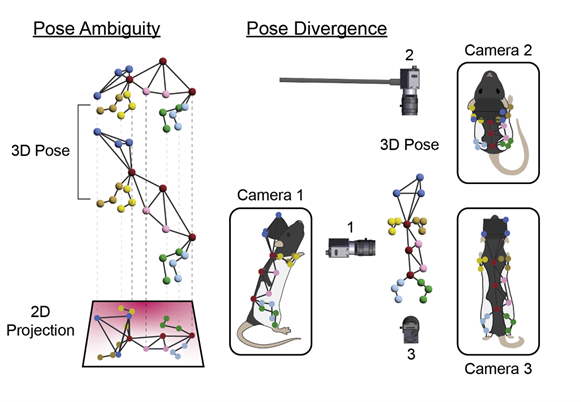
How does your new approach for multi-animal 3D tracking differ from previous tools?
People have recently developed 2D multi-animal tracking techniques, which can be powerful in certain situations. But for the reasons I just stated, they're missing out on a certain level of detail in terms of accurately capturing the 3D kinematics of these moving bodies. This level of detail is needed to fully describe social interactions, where there are lots of subtle body movements. Multi-animal experiments also pose a much more challenging computer vision problem, as animal bodies are often highly overlapping and occlusive during social interactions. We address these issues using multi-camera 3D tracking. The method is similar to our single-animal 3D tracking system but uses a graph neural network to resolve animal body overlaps.
Past approaches for automated social tracking have been coarse. For example, one can detect the position of each animal in a video and measure the time the animals spend together versus apart. But these coarse metrics belie a richer social interaction space. In our work, we have found that only when we quantify and analyse this richer space do we actually see consistent effects in response to drugs or in rat disease models. Many of these effects get averaged away when using coarse categories or metrics.
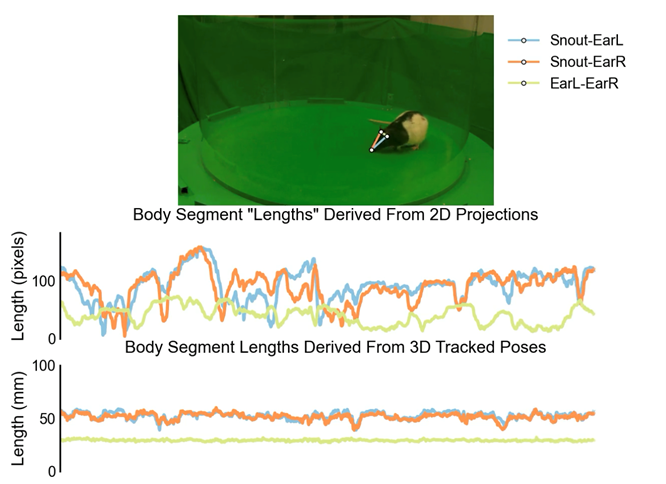
Why are you looking to simplify and standardize 3D tracking and how have you achieved this?
Several labs in the neuroscience community have developed their own software for pose tracking, and most labs are using a unique set of hardware. Everyone seems to be doing it differently. Their data formats, recording environments, hardware, and controlled variables are different. Often even the body parts being tracked vary. For these reasons, it's difficult to combine datasets across labs, hindering collaboration and preventing data consolidation on a large scale.
Unlike genomics, where standard protocols enable the aggregation of gene expression data, modern behavioural quantification lacks such standardized practices. This bottlenecks the establishment of global repositories and the leveraging of insights gained from large-scale studies. Standardization is crucial to support reproducibility and scalability in neuroscience. My lab, in collaboration with the Tadross lab at Duke, is working to simplify and standardize 3D tracking by developing compact behavioural enclosures that use a single camera plus mirrors in lieu of multiple independent cameras. We designed these enclosures to have standard illumination conditions and viewing angles, so that if a new enclosure is built to specifications then 3D pose tracking will just work out of the box.
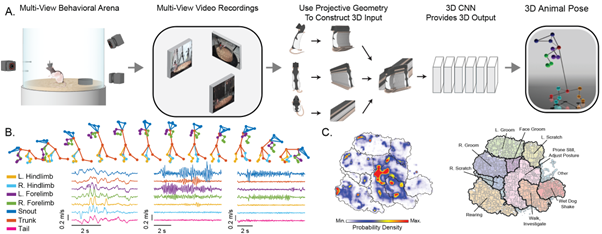
(A) Schematic showing how our system creates 3D volumes from multi-view video to infer the 3D positions of anatomical landmarks on the animal skeleton. Volumetric representations allow the CNN to learn spatial priors of the animal’s body. (B) Top, example inferred 3D wireframes of a rearing sequence. Bottom, examples of high-resolution kinematics for different behaviours and body landmarks. (C) 2D embedding (left) of hours-long 3D kinematic recordings reveals novel behavioural maps illustrating the organization (right) of hundreds of high- and low-level behaviours (dark and light lines, respectively). Map structures are one of many ways to phenotype with DANNCE.
How have you used the single-camera, mirror-based acquisition system to study motor deficits in Parkinson’s?
One of the exciting new results that I presented in my SWC talk was in profiling the movement of a mouse genetic model of Parkinson’s called VPS35. It's a mouse knock-in of a high penetrance mutation in humans that causes progressive motor deficits. This mouse mutant model first published in 2019 in PNAS. What was exciting about it was that for the first time someone had reported a histological phenotype in one of these genetic mouse models of Parkinson’s, which had never been seen before. There was a loss of substantia nigra neurons, which is a key component of human Parkinson’s.
While this was exciting, the paper also tried to look for motor deficits, the functional ramifications of this loss of neurons, and they couldn't find anything. They use standard assays: rotarod, gait analysis, balance analysis, and they couldn't find a difference in these mutants. There was a brain phenotype, but not the functional phenotype in terms of motor deficits.
When we used our single-camera 3D system to perform 3D pose tracking and analysis of this mouse line, we were able to identify motor deficits affecting the mouse behavioural repertoire. This opens the door to surveying new therapies, digging deeper into the mechanisms underlying these motor deficits, and how they relate to Parkinson’s neurodegeneration. All of this VPS35 mouse work was done in collaboration with the Tadross and Calakos labs at Duke.
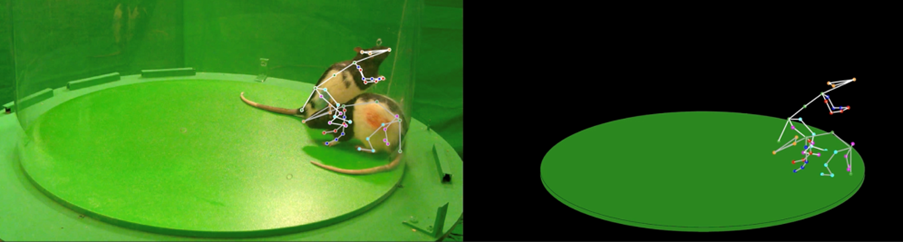
Social tracking by Dr. Ugne Klibaite (Olveczky Lab, Harvard) and Tianqing Li (Dunn Lab, Duke)
How have you used the 3D tracking to study social behaviours and what have you learned from using this approach to profile genetic models of autism?
We captured a surprisingly wide array of distinct social behaviours in rats using 3D tracking. This rich repertoire of social interactions exceeded our initial expectations. Our analysis pipeline is able to identify each of these interaction types and quantify how often they occur. This produces a behavioural profile, a fingerprint, for each animal that summarizes their social function, similar to how gene expression analyses profile the function of individual cells. Having quantitative and automated access to objective identifiers of various social behaviours provided us with a readout of nuanced behavioural changes associated with five different genetic models of autism.
This had eluded researchers for a long time. Similar to the challenges faced in Parkinson's models, traditional coarse metrics failed to capture consistent differences in social behaviour across groups compared to wild type controls. If you're solely focused on low-dimensional metrics like the time spent next to a social partner, it is difficult to measure significant differences between these groups. With a more precise decomposition of the social behavioural space into specific interaction categories, a consistent, significant difference emerges.
These autism phenotypes lay a foundation for future mechanistic studies and screens for new therapies in animal models.
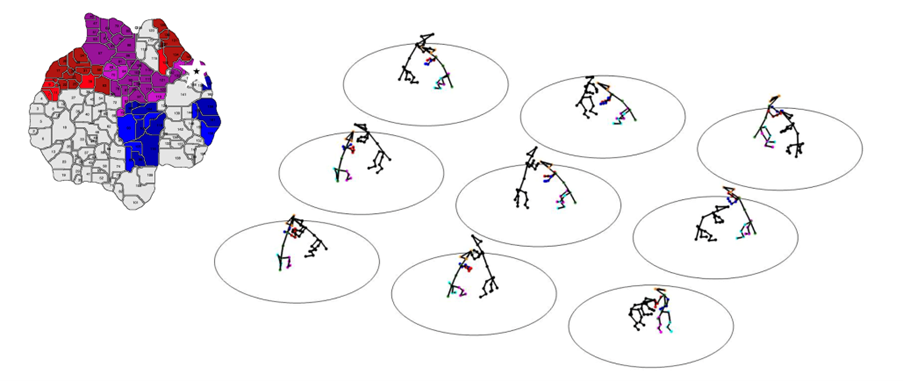
Social tracking by Dr. Ugne Klibaite (Olveczky Lab, Harvard) and Tianqing Li (Dunn Lab, Duke)
What other disease models do you hope the system will be used to understand?
In addition to Parkinson’s and autism, my collaborators and I are currently working on animal models of dystonia, stroke, and retinal degeneration. Ultimately, I think our system could be applied to any disease that has an effect on body movement. This includes diseases or disorders not traditionally classified as motor disorders. Autism is actually a good example of this. The motor system is normal but there is a cognitive effect that manifests in changes in behaviour. Behaviour is primarily conveyed by movement, which our system can measure. In the future, I am interested in exploring how our system can be used to understand other diseases, such as depression, that are considered particularly unique to humans and thus have been particularly difficult to study in animals models.
What do you think the future holds for 3D tracking technologies?
I believe the next significant step is to make 3D tracking technology more accessible to researchers around the world. I regularly encounter researchers eager to incorporate it into their work. However, the complexity of assembling and utilizing 3D tracking systems remains a barrier. Streamlining the process to make it more turnkey and easily integrated into experimental designs and workflows will be essential for advancing the technology and its impact.
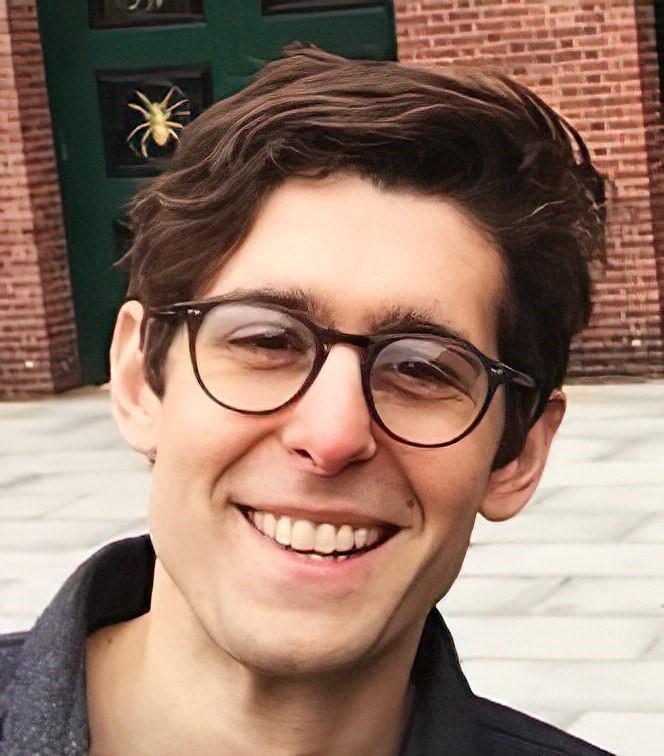
About Dr Dunn
Tim Dunn is an assistant professor of biomedical engineering at Duke University. His goal is to understand the neural basis of natural behaviour, which he has supported by developing technologies for high-resolution movement quantification in individuals and social groups. Tim was born and raised in Los Angeles, CA, USA. He graduated with a BA in Molecular and Cell Biology from UC Berkeley in 2008 and a PhD in Neurobiology from Harvard University in 2015. Tim received a McKnight Foundation Technological Innovations in Neuroscience Award in 2021.