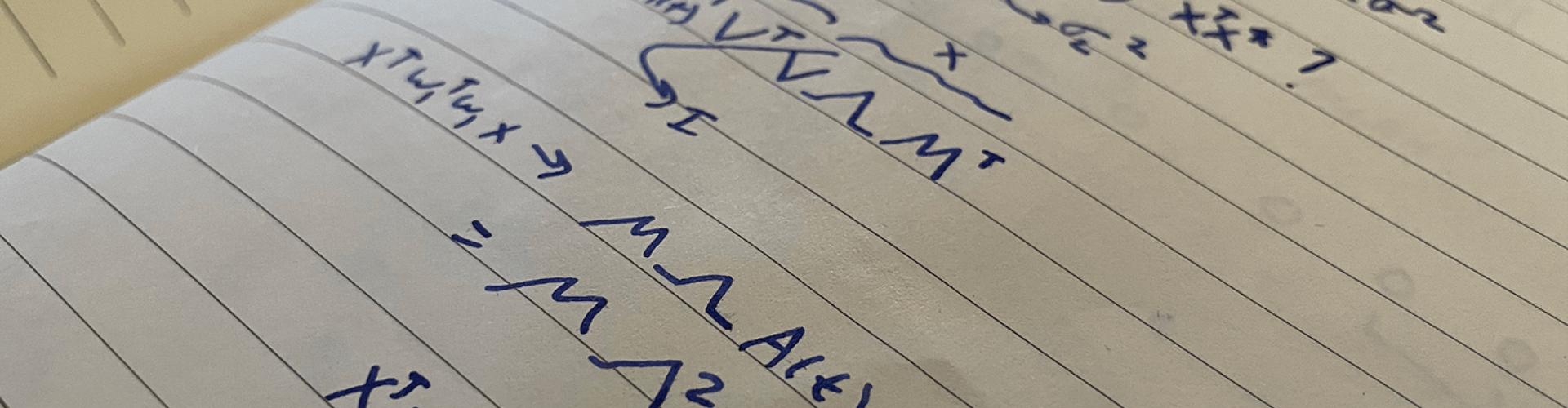
Theory of Learning Lab
Saxe Lab
Research Area
The interactions of billions of neurons ultimately give rise to our thoughts and actions. Remarkably, much of our behaviour is learned starting in infancy and continuing throughout our lifespan. We aim to develop a mathematical toolkit suitable for analysing and describing learning in the brain and mind. Our current focus is on the theory of deep learning, a class of artificial neural network models that take inspiration from the brain. Alongside this theoretical work, we develop close collaborations with experimentalists to empirically test principles of learning in biological organisms.
Research Topics
Unravelling the influence of learning on neural representations is a fundamental goal in neuroscience, because learning underpins a great diversity of behaviours; and developing better learning algorithms is a fundamental goal in machine learning, because it has proven essential in real world applications. Current research focuses on three directions:
1. Learning in complex architectures
The brain contains many layers of interconnected areas. We are investigating the dynamics of learning within complex recurrent, lateral, and top-down network structure, yielding a window into the relation between neural structure and learning dynamics. What are the principles that describe how neural representations change across a distributed network of interconnected brain areas during learning?
2. Learning with prior knowledge
What we already know changes how we learn. We are investigating the dynamics of learning new tasks with a neural network that already has pre-existing knowledge. The sensitivity of learning dynamics to prior knowledge is of fundamental importance to a variety of empirical phenomena including the impact of curriculum, schema-consistent learning, continual learning and meta learning.
3. Learning with nonlinearity and context
Living in our world requires responding differently to the same sensory input depending on context. We are investigating learning dynamics in nonlinear neural networks solving rich tasks. Nonlinearity is essential to nearly all real world tasks, and our approach aims to shed light on neural representations underlying task switching, multitasking, and abstraction.