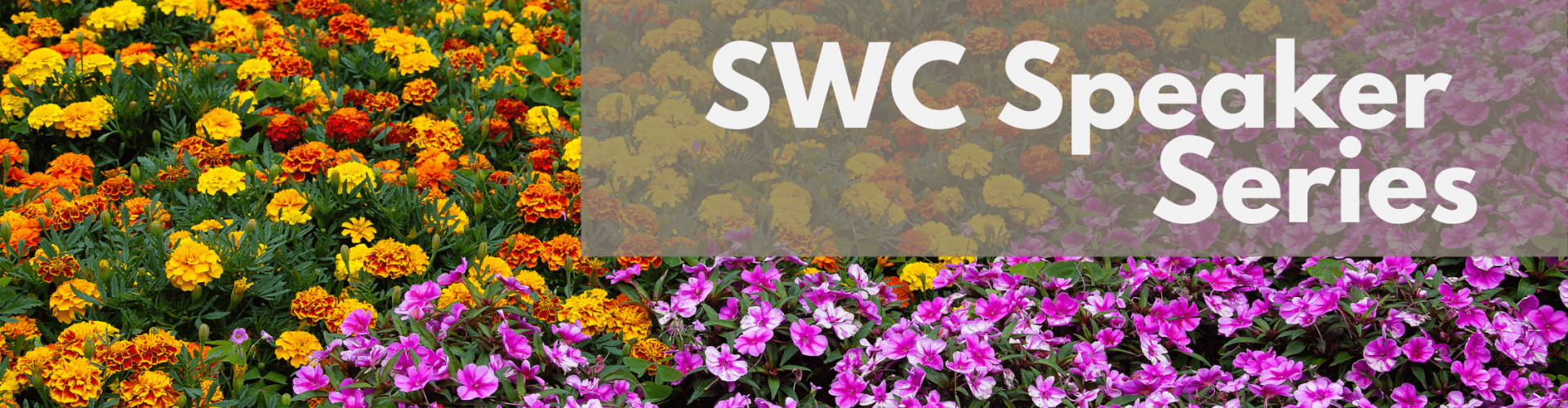
How does the brain represent categories?
An interview with Dr Pieter Goltstein, Max Planck Institute for Biological Intelligence (Munich), conducted by Hyewon Kim
Comedy. Thriller. Romance. Drama. Science fiction. We know these refer to different categories of film, but how does the brain learn and represent such categories? In a recent SWC Seminar, Dr Pieter Goltstein discussed his work exploring how the representation of a learned category in the mouse prefrontal cortex, as well as in higher visual areas, emerges over time. In this Q&A, he discusses what has already been known about category representations in the brain, the paradigm he used, future research plans, and more.
What was already known about how the brain represents categories?
There are two lines of research to consider. On the one hand, there are functional magnetic resonance imaging (fMRI) studies in humans that focus diversely on anything from identifying stimulus-specific sensory components of categorisation that activate early sensory areas in the brain, to how different types of categories activate different brain-wide networks.
And, there is indeed a big distinction there. First, there are verbal/explicit types of categories that humans are most familiar with. These can be described usually in a single sentence – for example, ‘categorize everything by colour: green or red.’ This is a very simple, one-dimensional categorization rule and is also referred to as rule-based categorization. Then, there are information-integration categories, which cannot be so easily described in words, the only way to learn them is through trial-and-error, in a process of implicit learning. A classical example is the task of a radiologist to discriminate tumours in X-ray images from smudges in the background. There is no simple way to describe the task, as it depends on multiple combinations of features and can only be learned by many repetitions and lots of feedback.
A model based on several fMRI studies argues that these two types of categories are learned by two different systems in the brain: an explicit, hypothesis-testing system and an implicit learning system, which takes longer to learn, but includes higher-dimensional comparisons. The model points towards the prefrontal cortex as being more involved in explicit learning and rule-learning, and parts of the basal ganglia as more involved in implicit tasks.
But this does not tell us much about how information of a single category is actually represented. Therefore, we need to look at what the cells in the brain are doing. The second line of research here has been carried out using single cell electrophysiology in the monkey. A landmark study was published in 2001 by David Freedman, in Earl Miller’s lab. Here, the authors asked monkeys to categorize morphed stimuli. They started with a picture of a dog and of a cat, and then gradually changed the shape of the dog into that of the cat. In addition, they generated images of these dog-cat like animals ranging from being very muscular to very slender, thus adding another dimension to the categorization problem. So now there could be different sorts of categories: cat versus dog and muscular versus slender – two different rules. Monkeys turned out to learn the categories very well, and they found that neurons in monkey prefrontal cortex were highly selective for the learned category rules.
More recent work has started to focus on the question of where in the brain we can find category selectivity; it is certainly not only in prefrontal cortex. For instance, there are neural correlates in the lateral intraparietal area (which is part of the parietal cortex) as well as inferotemporal cortex (IT). The idea is that category selective cells in these regions encode higher-level perceptual features. Thus, they tend to reflect the perceptual aspects of the category. For example, when confronted with a stimulus looking like something in-between a dog or a cat – IT cortex more directly reports what is seen, while prefrontal cortex very critically and strongly distinguishes categories.
The problem with early visual areas, on the other hand, is that the identity of most of these morphed stimuli is not represented directly. The above-mentioned stimuli are very complex patterns, while early visual areas rather serve as feature-detectors that detect e.g. the orientation of a line segment. However, when using simpler stimuli for categorization problems, i.e. oriented gratings, it is easy to find cells in the primary visual cortex that are also perfectly category-tuned. But this simplification of the stimulus comes with a problem: we don’t know if the category-selective neuronal responses were achieved with category learning or whether they were just a representation that was already there. This is why we decided to study category learning in the mouse over long periods of time, following the entire learning process from naïve to expert mouse. This is where we were able to go beyond earlier work in monkey and humans, where neuronal recordings were only done in highly experienced subjects. What we found is that also in higher visual areas, neurons change the way they respond to visual stimuli, such that they encode the learned categories better.
So, while a lot is known about how neurons in the brain responds to categories, we have a very limited idea of how the brain truly represents the categories. We know areas in the brain that are activated, cells which respond strongly to one category and not to other categories. But whether that is the representation, or just one activity pattern that is part of a population code or a higher-dimensional representation – we don’t know. That is something I would like to follow up on in the future.
How does your research try to understand how category-defining associations are represented in the brain? What is the paradigm that you used?
We recognised that in order to understand whether a representation or neuronal correlate in the brain is learned, we needed to follow neural activity over time. We needed to understand what was there before learning and how it then changed with learning. This could obviously be done in many types of model organisms, but to achieve tracking of neuronal activity at single-cell resolution over long periods of time, it was best done in the mouse. This is because in mice, we can use two-photon microscopy and calcium imaging to look at cortical activity as deeply as layer 5 of the visual cortex, and by using genetically encoded calcium indicators, we can follow the activity of identified cells over a long time. In addition, using mice also provides options for using other techniques like optogenetics, viral tracing, and other types of inactivation studies.
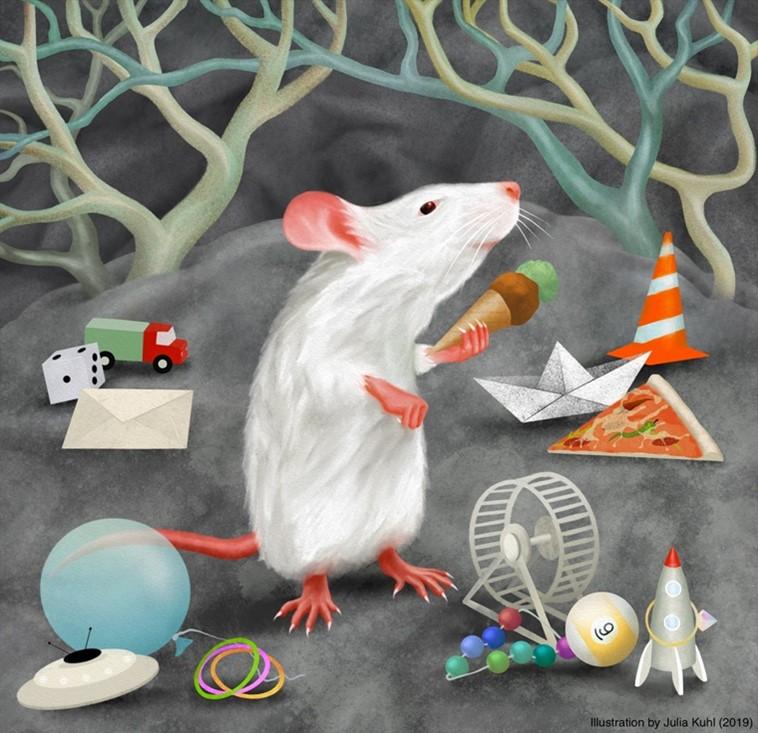
A mouse categorising several objects by shape (Illustration by Julia Kuhl, 2019)
We used a three-step approach, the first two of which we have achieved already. The first step was to behaviourally test whether mice can categorise. And using that behaviour, we needed to rule out the possibility that the animal is using a cognitive trick to ‘make us believe it is categorising, but is actually learning individual stimulus responses instead’. Using a method of rule-switching in the task, and then specifically investigating generalisation to stimuli that had previously been associated with another category – we could see that learning a category rule can override an existing learned association without any experience. That argues that existing single stimulus-response associations, if they had formed, were not the driving force behind the behaviour – it was actually the category rule. Therefore, we think that mice tend to categorise rather than learn lots of stimulus-associated responses for many different stimuli.
The second step was to follow neuronal activity with learning over time. There were two ways of going on the quest to find where in the brain this all happens. One way was to use the existing literature (let’s call it common knowledge) to make a best estimate of a candidate brain area, and then just go for it. That is actually what we did. We chose the prefrontal cortex based on the parallel with monkey research and we chose visual cortex because we know the visual cortex is the place in the mouse brain where visual information in the cortex is first processed (and visual cortex cells can also represent the stimuli that are shown). The alternative would be to use methods that look at brain-wide activity to potentially identify areas that we haven’t even thought might play a role in the task, but are still relevant. This is something I plan to do in the future.
Having identified those brain regions, we then followed neuronal activity over time when the mouse was performing the task to see how the activity changes with category learning. We found that prefrontal cortex cells become highly category-selective, but don’t respond to visual stimuli at all before learning. However, in the visual cortex, it’s different – cells already respond to visual stimuli before learning. And we only see in the higher visual area called POR (postrhinal area) a clear change that biases cells toward categories. However, one cautionary note is that these are only a small subset of POR neurons that do this and also, the change in activity is not very large. The change in comparison to overall visual activity, and other task related variable like reward activity, choice selective activity, and motor activity, is very minor. The reason we could detect the change is because we use a computational approach (an encoding model) to separate out all the different components driving the neurons. We are relatively confident that we are not extracting signal from pure noise because we of course perform the same analysis for eight other visual areas, including the primary visual cortex. In seven of them, we find no change at all, and only in V1 in addition to POR that we see a similar tendency. The third part of our approach that we did not get to do yet is to understand whether these changes depend on specific changes in synaptic weight or synaptic connectivity. We hope to answer this in the future.
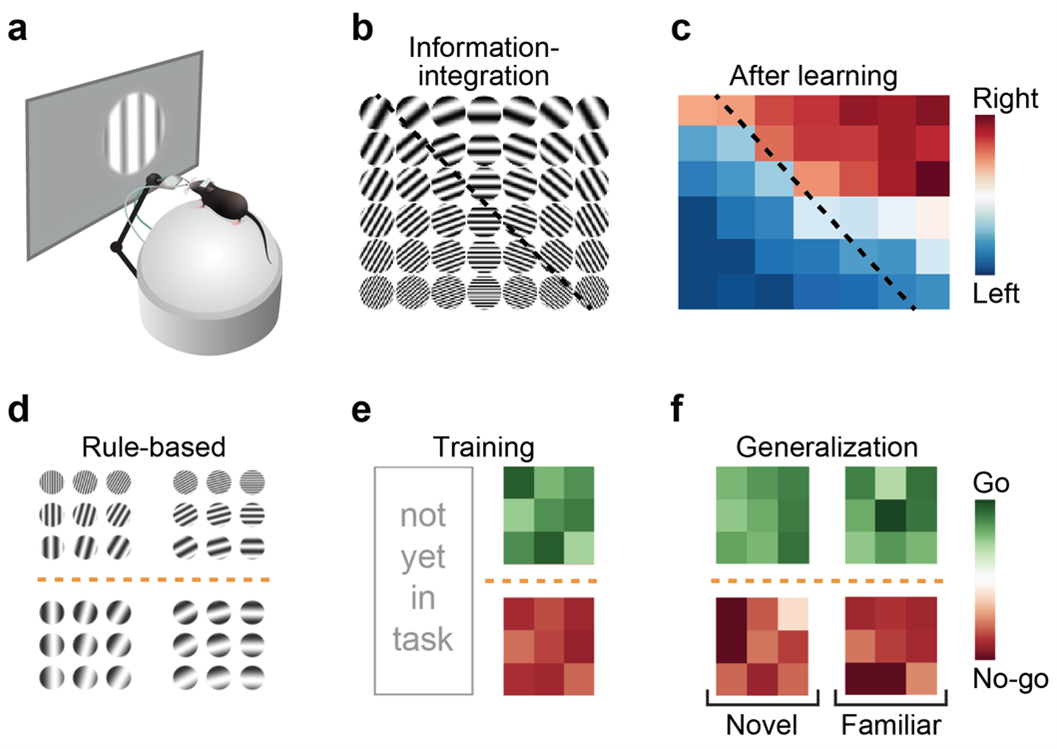
Visual category learning in mice. A Schematic showing a head-fixed experimental setup for visual learning in mice. B, C Example two-dimensional stimulus space with an information-integration category boundary and the categorization performance of an example mouse. D Stimulus space with a rule-based category boundary. E Example rule-based categorization performance. F Generalization of the learned category rule (novel versus familiar stimuli).
Were you surprised to find that some neurons are already selective to categories before learning, and that category selectivity after learning increases most strongly in area POR?
When I started my postdoc, we initially looked at the primary visual cortex of mice that I had trained on a categorization paradigm in behavioural chambers (which did not allow us to observe and follow neuronal activity directly during learning). We thought that we might find some differently tuned neurons after learning. This is when we realised that in the visual cortex, there are so many diverse tuning properties that we would never find a clear signature of a category learning paradigm, unless we follow over time how changes occur on top of what is already there.
I actually found it surprising that we ended up finding only area POR as increasing its selectivity for categories. I thought about this for a while, but I cannot come up with a watertight explanation. There is a model predicting that the convergence of choice and reward activity with stimulus drive can lead to category selectivity. But, those signals are available in all visual areas. So it must be something else that is special about POR – it could have to do with the reward activity being stronger, but it could also be that the reward activity in area POR is somehow more strongly tied to synaptic plasticity rules while in the other areas, it is possibly less so.
Another possibility is that area POR may receive more diverse visual inputs and therefore has a large possibility of sampling other stimulus properties, and so, maybe the POR cell gets a broader synaptic drive. In other words, possibly, POR has more diverse synaptic inputs, giving the neurons a better chance that they already have some synaptic inputs from neurons encoding the different stimuli that will become part of the category, thus only requiring specific synapses to scale up in synaptic weight to make the POR neuron more category selective. Vice-versa, it could be that other areas have more selective input distributions. Thus, if a cell were to change its response property and extend to include more stimuli belonging to a category, it would have to find altogether new synaptic partners. That is maybe a somewhat far-fetched hypothesis, but it is a prediction that could be tested – whether the tuning properties of synaptic inputs to POR cells show fundamentally different distributions from other higher visual areas.
The biggest surprise was when I performed local inactivations of either V1, the pathway from V1 leading to POR, or visual areas AL and RL. While all these areas are part of different stages and pathways of visual information processing, the effect on the behaviour was almost the same. I found that very surprising. What that tells us is that a significant part of the behaviour is likely carried out by circuits that are (possibly far) more downstream. From primary visual cortex, the signals first diverge into higher areas, but somewhere they must converge again, and that convergence will include signals that went over all the multiple parallel pathways. Apparently, all those pathways are involved in categorisation behaviour and visual behaviour. I found this surprising, even though it should not have been.
What will the work in your future lab focus on?
My future lab will try to establish what the cognitive components of the categorisation process are, and how the brain is solving those components. Categorisation is not a one-step process, there are many aspects to it and different variations of it. One idea is to contrast such tasks, varying one feature at a time, to isolate the component defining that specific version of the category task. One task could, for instance, include a component of linearising a continuous space of orientations, and another task could have arbitrary categories. If you contrast those, you may find that there are specific brain areas involved in this perceptual process of extracting features into a single linear representation as compared to when that operation is not needed. As such, there are many different aspects of categorisation that one could test and then identify which brain areas are most strongly involved respectively. My idea is to do this using brain-wide methods, such as using immediate early gene expression, but also using newly developed methods for whole-brain imaging, such as functional ultrasound imaging.
Next, we need to verify whatever hints of activated brain areas we find using such approaches, whether those areas are indeed involved in behaviour. Using the contrasting tasks, but now inactivating the identified brain areas, we will confirm whether one of the two tasks will be disproportionately affected.
A final component is to understand what the cellular correlates are. Even if we know that the area is activated by a specific part of the categorisation task, and that the behaviour is causally affected by inactivating the brain area, we don’t know if the cells in the brain area directly contribute to categorisation or if they are part of some other process that supports the behaviour in a sideways related manner. As an example, it could be that we identify an area involved in reward feedback, which shows as activated during category learning, and taking out that area will make the task unlearnable. Only by knowing the cellular correlates we can learn if this area represents categories or some other variable, such as reward feedback.
That is where electrophysiology and calcium imaging come in. The goal would be to first identify in a single area, and later from multiple brain areas simultaneously, how cells represent the categories. This would include not only single cell correlates, which I think are a first step to look at neural activity, but also include methods like manifold analysis to better understand how the brain is trying to represent the information across a large group of neurons. By disentangling the subprocesses of categorisation, we can also have a better understanding of what should be the representation at different points in the information pathway, from sensation to motor action. The idea is that the manifold dimensions might align with various cognitive, sensory and motor related components of the task, and can thus be tied to what is specifically being represented by the recorded population activity.
In addition, something that I find very interesting, but is a part of my project that always ends up being pushed toward the longer term, is that when we learn, many things in the brain physically change. And the brain is able to hold onto these changes. Obviously, this is likely achieved by synaptic plasticity, either being a change in synaptic weights, by adding more inputs to the same cell, or by creating completely novel synaptic connectivity. Any of these would be interesting to study in the context of category learning. When we have identified areas where we know that there is a change between area X and area Y, for example, or there is a transfer of neural activity where area X affects area Y more directly, then we can look at whether there are structural changes underlying these functional changes.
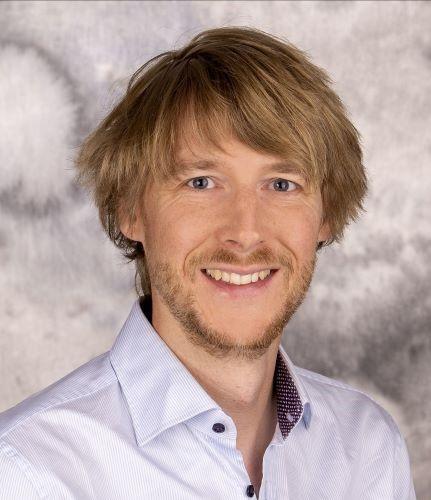
About Dr Pieter Goltstein
Pieter Goltstein is a postdoctoral researcher working with Mark Hübener and Tobias Bonhoeffer at the Max Planck Institute for Biological Intelligence in Martinsried, close to Munich. During his postdoc he developed paradigms for studying category learning in mice, with the goal to investigate neuronal mechanisms of semantic memory. Pieter has a Bachelor and master’s degree in psychology and obtained his PhD degree in Neurobiology at the University of Amsterdam, investigating effects of learning and behavioural state on primary visual cortex.